Neighborhood Graph Convolutional Networks for Recommender Systems
ADVANCES IN NATURAL COMPUTATION, FUZZY SYSTEMS AND KNOWLEDGE DISCOVERY, ICNC-FSKD 2022(2023)
摘要
Recommendation system based on collaborative filtering has attracted much attention because it can make personalized recommendations according to the different needs of different users. However, a training recommender system based on collaborative filtering needs a large amount of user-item interaction information. In practical applications, the collaborative filtering recommender system has problems of cold start and sparsity due to the lack of information in the data. The researchers noticed that the knowledge graph contained a lot of attribute relations and structured information. In order to alleviate and solve the problems of cold start and sparsity in the existing recommendation system, the researchers tried to introduce the knowledge graph into collaborative filtering. However, the existing recommendation systems based on knowledge graphs usually only pay attention to the project information and use the project information to model user preferences, but seldom pay attention to the user information. In this paper, we discuss the necessity of using user information and propose a new convolutional neural network framework, which is an end-to-end framework, which explores the correlation attributes in the knowledge graph to capture the correlation, and then to model high-order user information and high-order project information. It is worth mentioning that we use 9 different ways to aggregate high-order user neighborhood information and high-order project neighborhood information based on 3 different aggregators. The proposed model is applied to two data sets about books and music, and the results show that the accuracy is significantly improved, which proves the superiority of our method.
更多查看译文
关键词
Recommender systems,Knowledge graph,Graph convolutional networks
AI 理解论文
溯源树
样例
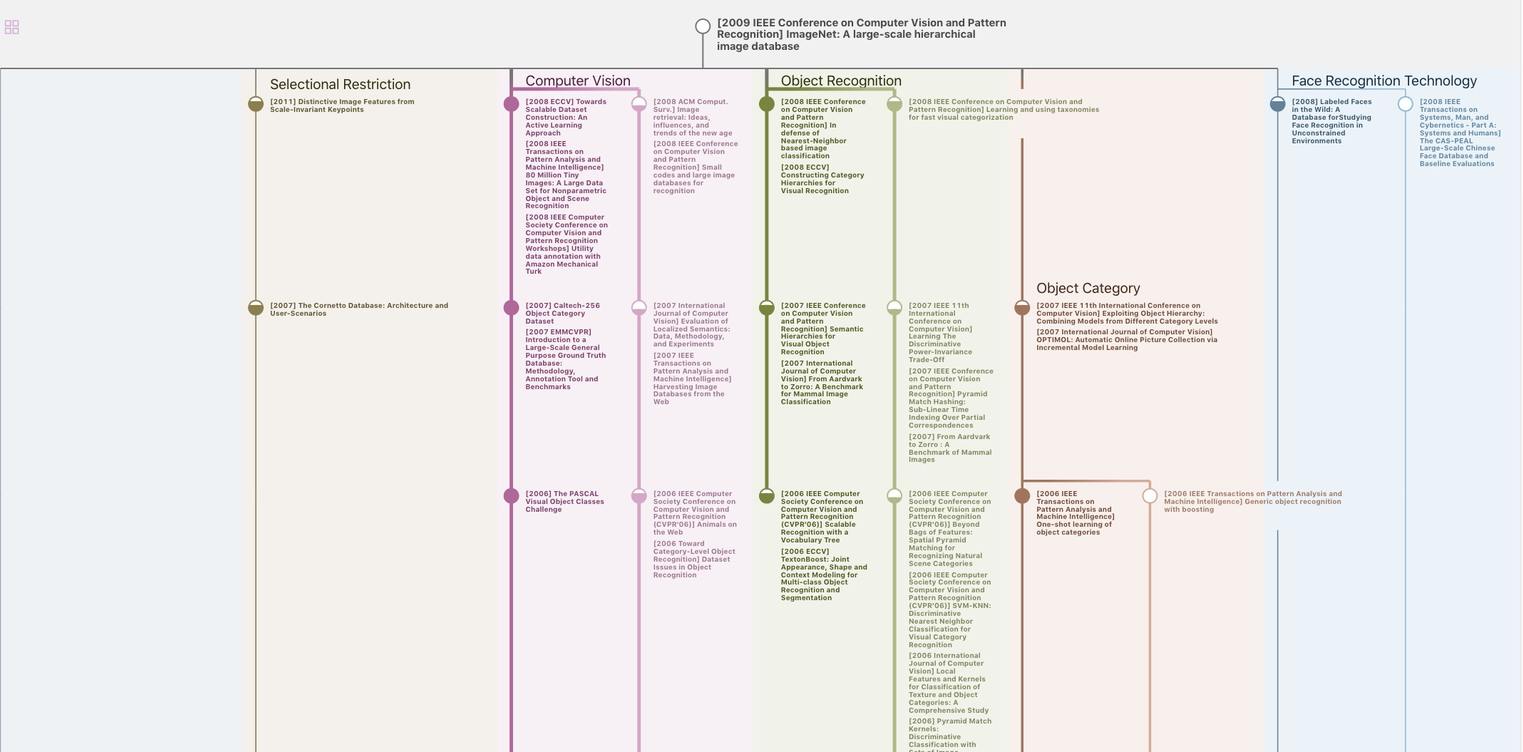
生成溯源树,研究论文发展脉络
Chat Paper
正在生成论文摘要