Machine learning-based beta transus temperature prediction for titanium alloys
JOURNAL OF MATERIALS RESEARCH AND TECHNOLOGY-JMR&T(2023)
Abstract
Beta transus temperature (8tr) is one of the most crucial features of titanium alloys. It is typically used as the index while designing the heat treatment process for titanium alloys. The 8tr is also a significant parameter to optimize the processing technology of titanium alloys. Four machine learning algorithms and one empirical formula is developed in this study to estimate the 8tr of titanium alloys: Artificial Neural Networks (ANN), Gauss Pro-cessing Regression (GPR), Super Vector Machine (SVM), and Ensemble Regression Trees (ERT). According to the correlation coefficient (R), Mean Absolute Error (MAE) and Root Mean Square Error (RMSE) to verify the accuracy of models, and the experimentally measured phase transition temperature of Ti600 alloy was also used to verify the gener-alization ability of the model. Choosing the best model to analyze the sensitivity of the elements and determine how each component affects the 8tr. The result demonstrated that the ANN model has the highest prediction accuracy among the five models, and different model structures have different effects on predicting new data. The ANN model with 10 neurons has the highest prediction accuracy, while the ANN model with 8 neurons has the strongest generalization ability. The results of the sensitivity analysis proved that all the alloy compositions used as input parameters were valid parameters. (c) 2023 The Author(s). Published by Elsevier B.V. This is an open access article under the CC BY license (http://creativecommons.org/licenses/by/4.0/).
MoreTranslated text
Key words
Beta transus temperature,Machine learning,Titanium alloys,Empirical formula,Sensitivity analysis
AI Read Science
Must-Reading Tree
Example
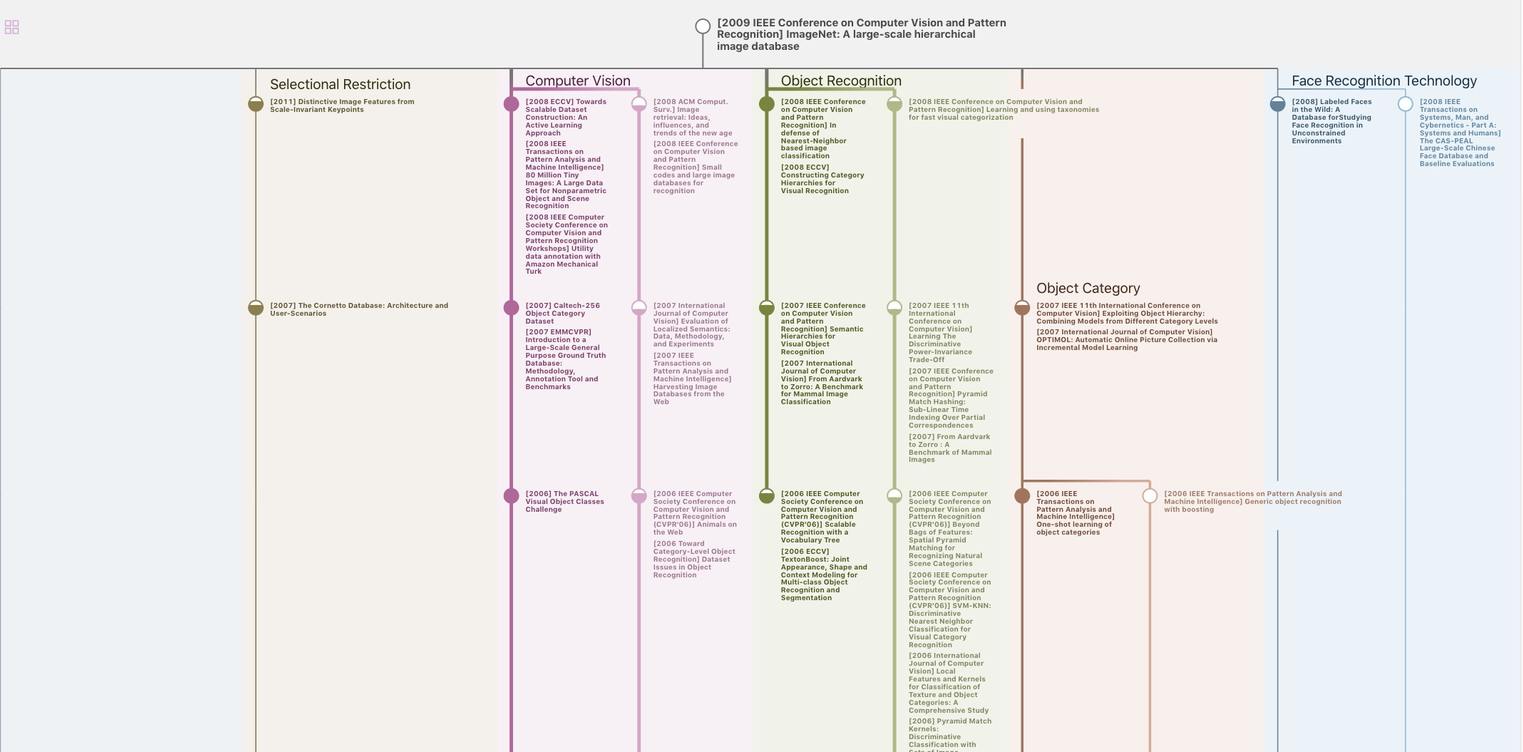
Generate MRT to find the research sequence of this paper
Chat Paper
Summary is being generated by the instructions you defined