Research on Quantitative Regression Method of IR Spectra of Organic Compounds Based on Ensemble Learning With Wavelength Selection
wos(2023)
Abstract
The application of the ensemble learning method in the quantitative analysis of organic infrared spectra and the influence of the characteristic wavelength selection method on the modeling efficiency and prediction accuracy of infrared spectra ensemble learning is studied. Taking the cetane number and total aromatic hydrocarbon content of diesel infrared spectra as the research object, firstly, a two-layer stacking ensemble learning framework is established by using extreme random forest (ERT), linear kernel support vector machine ( LinearSVM), radial basis kernel support vector machine (RBFSVM) and polynomial kernel support vector machine ( polySVM) as baselearners, and LinearSVM as meta-learners. The quantitative regression accuracy of diesel infrared spectra by single base learners and ensemble learning model is analyzed and compared. Compared with the partial least squares (PLS) quantitative regression model, the prediction accuracy of the Stacking ensemble learning model for two organic compounds in diesel spectra is improved. The ERT model for cetane number content is the best (r =0. 848, RMSEP=1. 603, RDP = 2. 627), the prediction result of Stacking model for total aromatic content is the best (r =0. 991, RMSEP=0. 645, RDP= 9. 243). Further, the characteristic wavelengths of infrared spectra are selected using the combined partial least squares (SiPLS) and successive projections algorithm (SPA), and the ensemble learning quantitative regression model is established using the selected characteristic wavelengths. Among them, the prediction results of the SiPLSERT model for cetane number content are the best (r = 0. 893, RMSEP= 1. 013, RDP= 3. 051), and the prediction results of the SiPLS-Stacking model for total aromatic content are the best (r = 0. 998, RMSEP = 0. 354, RDP = 11. 475), and the average training time of the model is reduced by more than 50% compared with the full spectra training time, and the modeling speed is significantly improved. The results show that the characteristic wavelengths combined with ensemble learning quantitative regression modeling can be used in the quantitative analysis of organic infrared spectra. Compared with the traditional quantitative regression method, the modeling efficiency and prediction accuracy of this method are greatly improved, which provides relevant method support for the further study of the application of machine learning in the quantitative analysis of spectra.
MoreTranslated text
Key words
Ensemble Learning,Quantitative regression,Characteristic wavelength selection,Infrared spectra of organic compounds
AI Read Science
Must-Reading Tree
Example
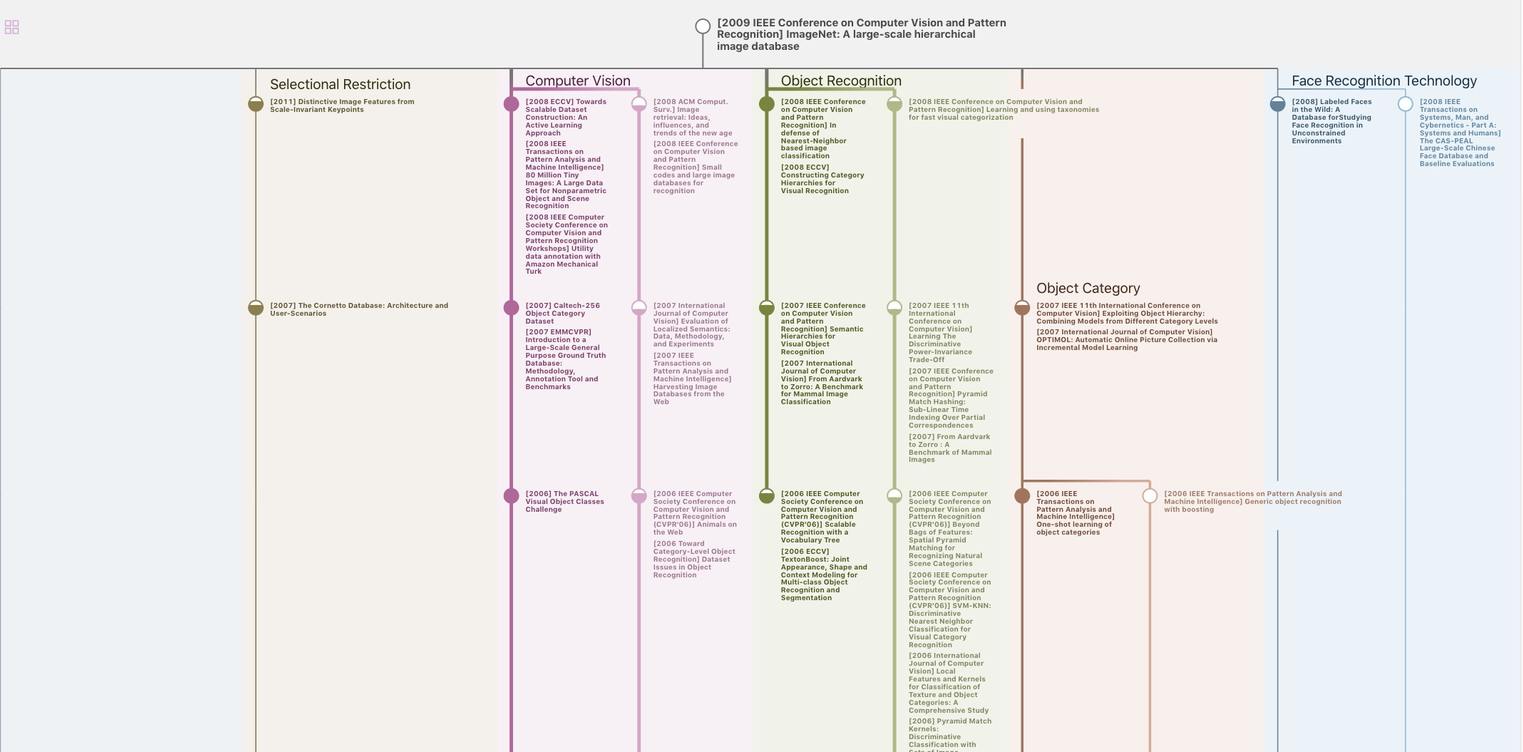
Generate MRT to find the research sequence of this paper
Chat Paper
Summary is being generated by the instructions you defined