Detecting and correcting for heteroscedasticity in the presence of measurement error
COMMUNICATIONS IN STATISTICS-SIMULATION AND COMPUTATION(2023)
摘要
The problem of measurement error in predictors has received a vast amount of attention for both linear and non-linear models. The major focus has generally been on inference for regression coefficients, while residual analysis, variance estimation and heteroscedasticity assessment have received limited attention. In this paper we propose and evaluate new methods, based on reconstructed predictors, to address these problems in the context of linear regression. Methods using moment reconstructed predictors are found to perform well, including a new model-based bootstrapping technique for testing heteroscedasticity. Motivated by an example where the predictor is methylation rate and the measurement error model is based on the binomial distribution, particular attention is given to being able to handle the situation where both the measurement error variances and the variance of the error in the equation may be changing as a quadratic function of the unobserved predictor.
更多查看译文
关键词
Bootstrap,Heteroscedasticity,Measurement error,Moment reconstruction,White's test
AI 理解论文
溯源树
样例
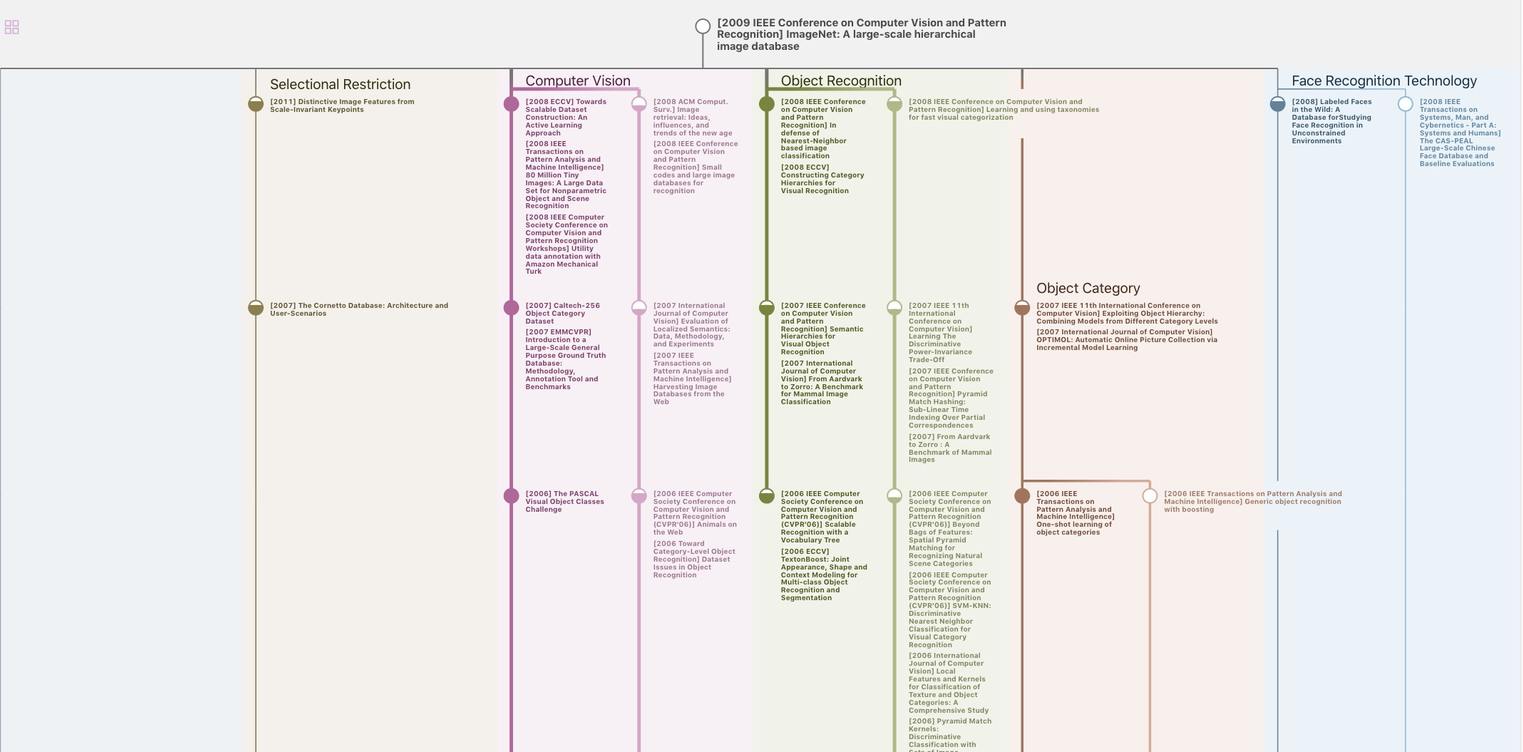
生成溯源树,研究论文发展脉络
Chat Paper
正在生成论文摘要