Does the asymmetric exponential power distribution improve systemic risk measurement?
JOURNAL OF RISK MODEL VALIDATION(2023)
摘要
The measurement of systemic risk using parametric modeling suffers from fat-tailedness, asymmetric kurtosis and asymmetric tails. Prior research shows that the asymmetric exponential power distribution (AEPD) can potentially avoid overfitting and underfitting problems because it can be reduced to a Gaussian distribution and a generalized error distribution. This paper implements a parametric estimation for the systemic risk measure CoVaR (ie, conditional value-at-risk) of Huang and Uryasev and compares the goodness-of-fit and backtesting performance of the AEPD with other commonly used distributions (ie, the normal, Student t and skewed t distributions). Based on data from the Chinese banking sector from 2008 to 2019, the empirical results show that AEPD has the best goodness-of-fit. Moreover, it is the only distribution that provides a validated estimation for CoVaR.
更多查看译文
关键词
systemic risk,asymmetric exponential power distribution (AEPD),conditional value-at-risk (CoVaR),Chinese banking sector,parametric estimation
AI 理解论文
溯源树
样例
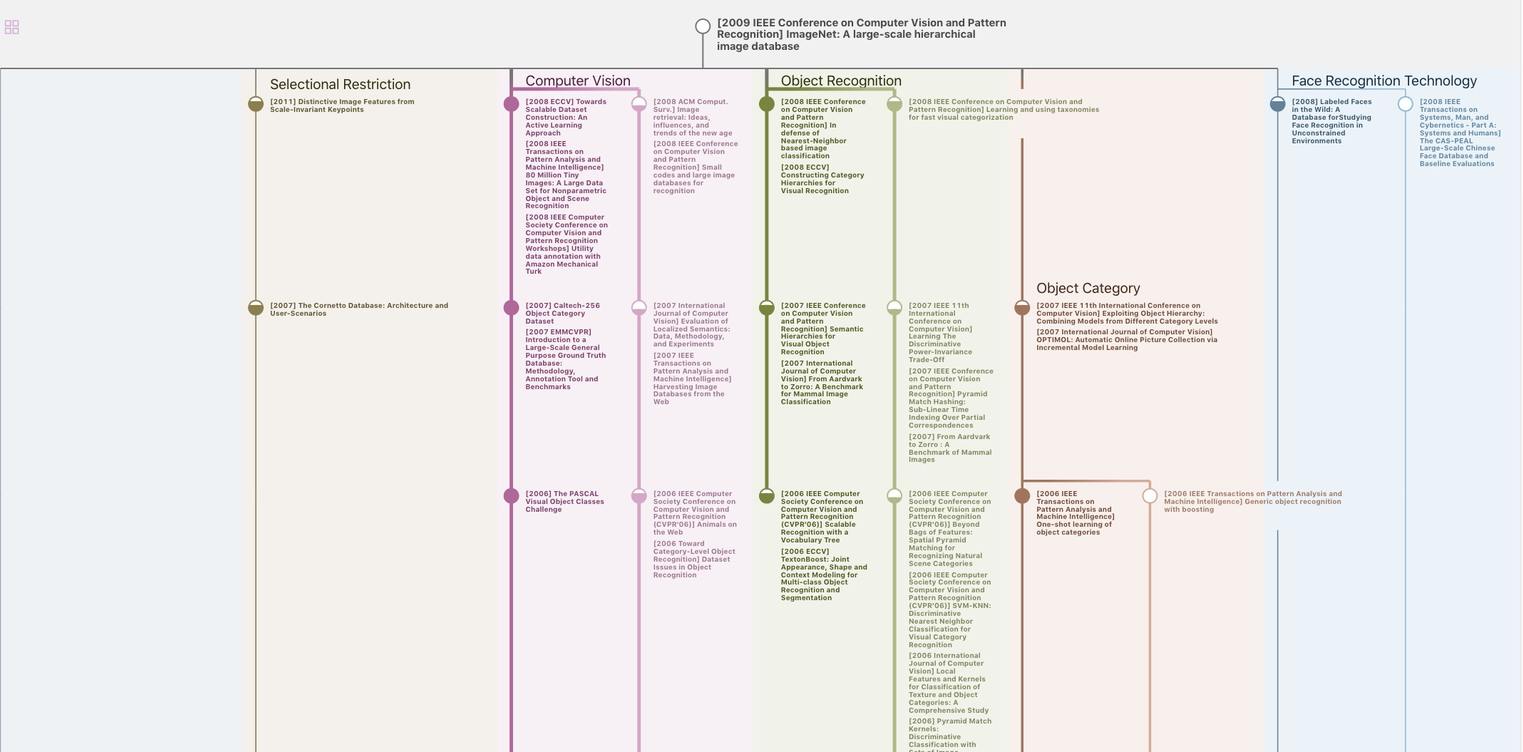
生成溯源树,研究论文发展脉络
Chat Paper
正在生成论文摘要