Performance Improvement of LSTM-based Deep Learning Model for Streamflow Forecasting Using Kalman Filtering
WATER RESOURCES MANAGEMENT(2023)
摘要
Prediction of streamflow as a crucial source of hydrological information plays a central role in various fields of water resources projects. While accurate daily streamflow forecasts are essential, predicting streamflow based on limited data is useful for minimizing computational time and supporting flood early warning systems. This study aims to improve Long Short-Term Memory (LSTM) performance by Kalman filter (KF) for streamflow forecasting. For this goal, the simulation has been specified according to the daily streamflow series for 60 years of Dez Dam, located in Iran. We compared the results of simulating the LSTM, LSTM-KF, LSTM-UKF, LSTM-KFS, and LSTM-UKFS models. In addition, the accuracy of the proposed method is evaluated with statistical analysis including Nash–Sutcliffe efficiency (NSE), root-mean-squared error (RMSE), average absolute relative error (AARE) and mean relative error (MAE). The results demonstrate that the LSTM-UKFS model is highly effective in flood forecasting, indicating the promising potential of a simple architecture deep-learning approach for predicting floods, even in the presence of dams in the study area.
更多查看译文
关键词
Streamflow forecasting,Machine learning,LSTM,Deep learning,KF
AI 理解论文
溯源树
样例
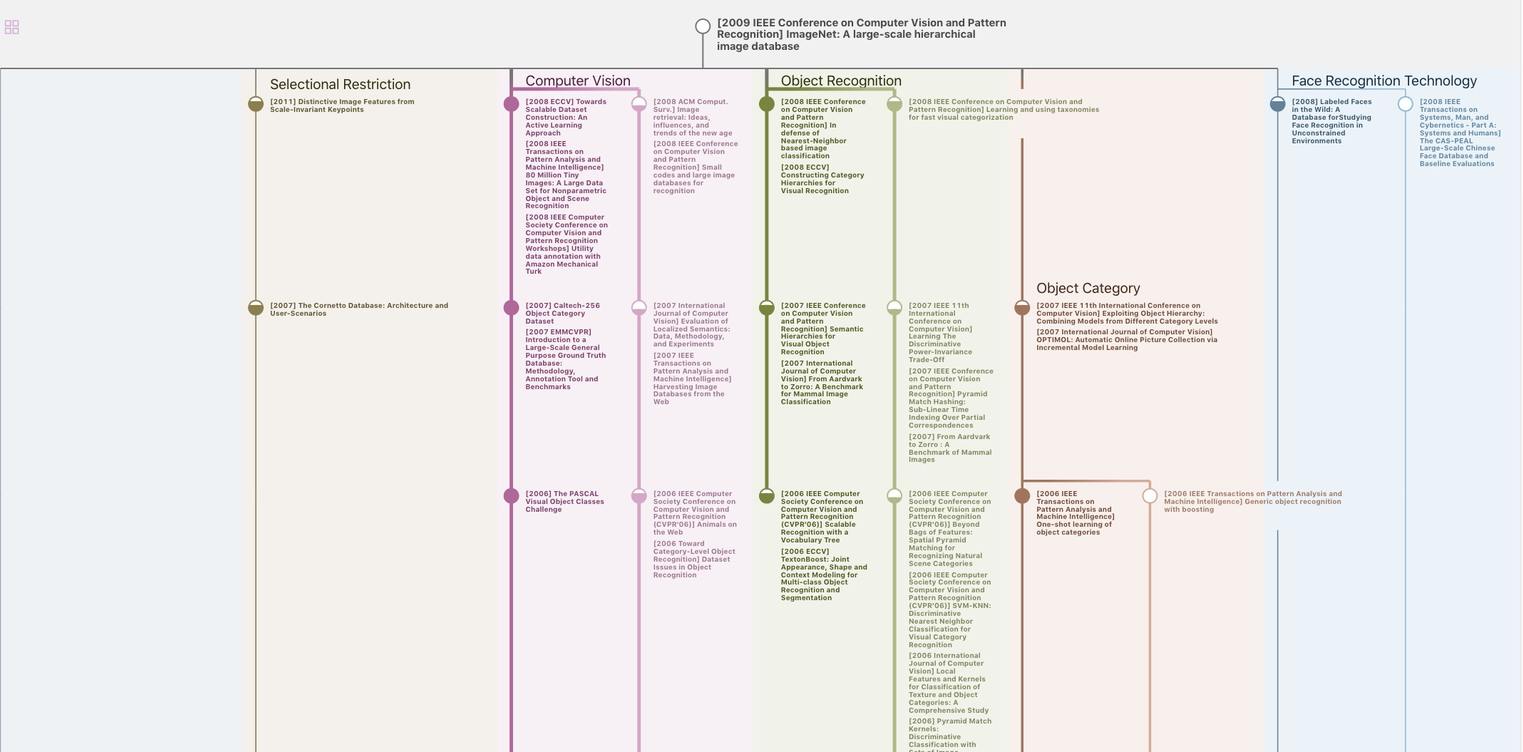
生成溯源树,研究论文发展脉络
Chat Paper
正在生成论文摘要