A Cross-Scale and Illumination Invariance-Based Model for Robust Object Detection in Traffic Surveillance Scenarios
IEEE TRANSACTIONS ON INTELLIGENT TRANSPORTATION SYSTEMS(2023)
摘要
Robust object detection methods in traffic surveillance scenarios often encounters challenges due to large-scale deformations and illumination variations in outdoor scenes. To enhance the tolerance of such methods against these variations, we design a cross-scale and illumination-invariant detection model (CSIM) based on the You Only Look Once (YOLO) architecture. A main cause of false detection in large-scale detection tasks is the inconsistency between various feature scales. To address this issue, we introduce an adaptive cross-scale feature fusion model to ensure the consistency of the constructed feature pyramid. To overcome the influence of uneven light, we build an illumination-invariant chromaticity space on the CSIM model, which is independent of the correlated color temperature. In addition, we adopt spatial attention modules, K-means clustering and the Mish activation function for further model optimization. The obtained experimental results show that the proposed CSIM produces excellent detection results for addressing the challenges derived from large-scale deformations and the illumination changes encountered during traffic surveillance. Compared with state-of-the-art object detection methods on public datasets, our proposed model has achieved competitive results in robust object detection tasks in traffic surveillance scenarios.
更多查看译文
关键词
Feature extraction,Object detection,Lighting,Traffic control,Adaptation models,Task analysis,Robustness,Traffic detection,surveillance scenarios,spatial feature fusion,illumination invariance
AI 理解论文
溯源树
样例
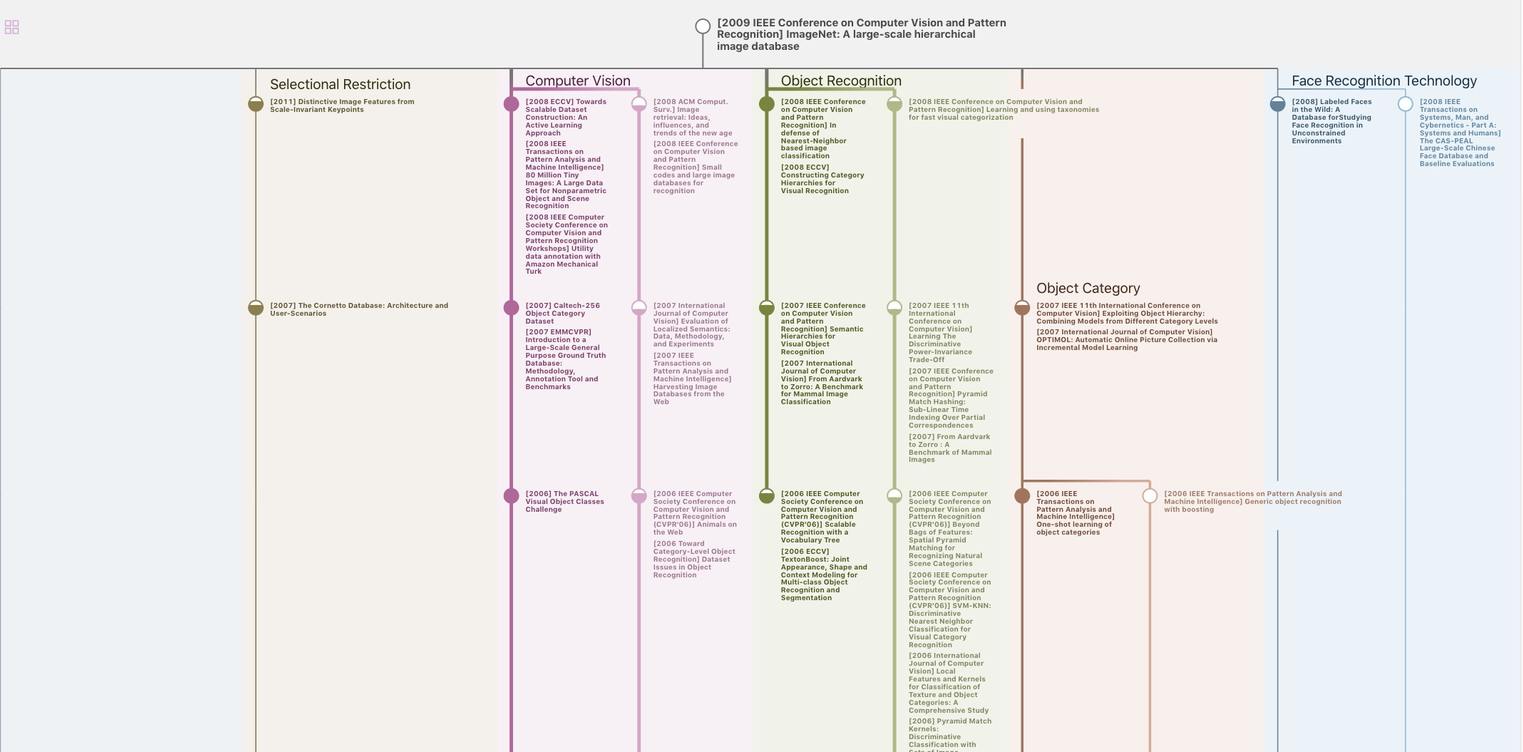
生成溯源树,研究论文发展脉络
Chat Paper
正在生成论文摘要