Global spatio-temporal synergistic topology learning for skeleton-based action recognition
PATTERN RECOGNITION(2023)
摘要
Compared to RGB video-based action recognition, skeleton-based action recognition algorithm has attracted much more attention due to being more lightweight, better generalization and robustness. The extraction of temporal and spatial features is a crucial factor for skeleton-based action recognition. However, existing feature extraction methods suffer from two limitations: (1) the isolated extraction of temporal and spatial feature cannot capture temporal feature connections among non-adjacent joints and (2) convolution-limited perceptual fields cannot capture global temporal features of joints effectively. In this work, we propose a global spatio-temporal synergistic feature learning module (GSTL), which generates global spatio-temporal synergistic topology of joints by spatio-temporal feature fusion. By further combining the GSTL with a temporal modeling unit, we develop a powerful global spatio-temporal synergistic topology learning network (GSTLN), and it achieves competitive performance with fewer parameters on three challenge datasets: NTU RGB + D, NTU RGB + D 120, and NW-UCLA.(c) 2023 Elsevier Ltd. All rights reserved.
更多查看译文
关键词
Action recognition,Spatio-temporal synergistic,Skeleton,Topology learning
AI 理解论文
溯源树
样例
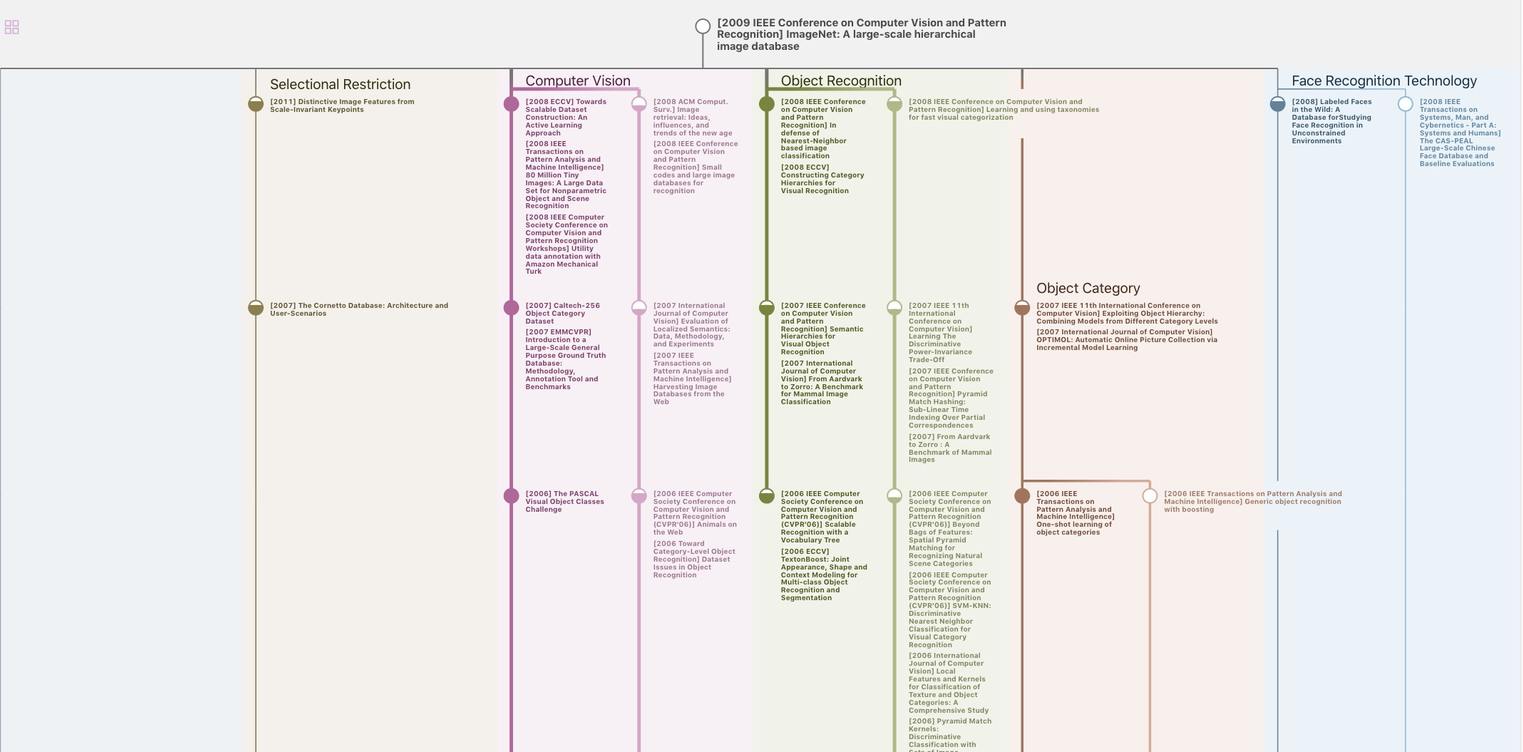
生成溯源树,研究论文发展脉络
Chat Paper
正在生成论文摘要