Adaptive Hybrid Optimization Learning-Based Accurate Motion Planning of Multi-Joint Arm
IEEE TRANSACTIONS ON NEURAL NETWORKS AND LEARNING SYSTEMS(2023)
摘要
Motion planning is important to the automatic operation of the manipulator. It is difficult for traditional motion planning algorithms to achieve efficient online motion planning in a rapidly changing environment and high-dimensional planning space. The neural motion planning (NMP) algorithm based on reinforcement learning provides a new way to solve the above-mentioned task. Aiming to overcome the difficulty of training the neural network in high-accuracy planning tasks, this article proposes to combine the artificial potential field (APF) method and reinforcement learning. The neural motion planner can avoid obstacles in a wide range; meanwhile, the APF method is exploited to adjust the partial position. Considering that the action space of the manipulator is high-dimensional and continuous, the soft-actor-critic (SAC) algorithm is adopted to train the neural motion planner. By training and testing with different accuracy values in a simulation engine, it is verified that, in the high-accuracy planning tasks, the success rate of the proposed hybrid method is better than using the two algorithms alone. Finally, the feasibility of directly transferring the learned neural network to the real manipulator is verified by a dynamic obstacle-avoidance task.
更多查看译文
关键词
Planning,Manipulators,Heuristic algorithms,Training,Reinforcement learning,Manipulator dynamics,Task analysis,Artificial potential field (APF),hybrid dynamic strategy,manipulator motion planning,reinforcement learning
AI 理解论文
溯源树
样例
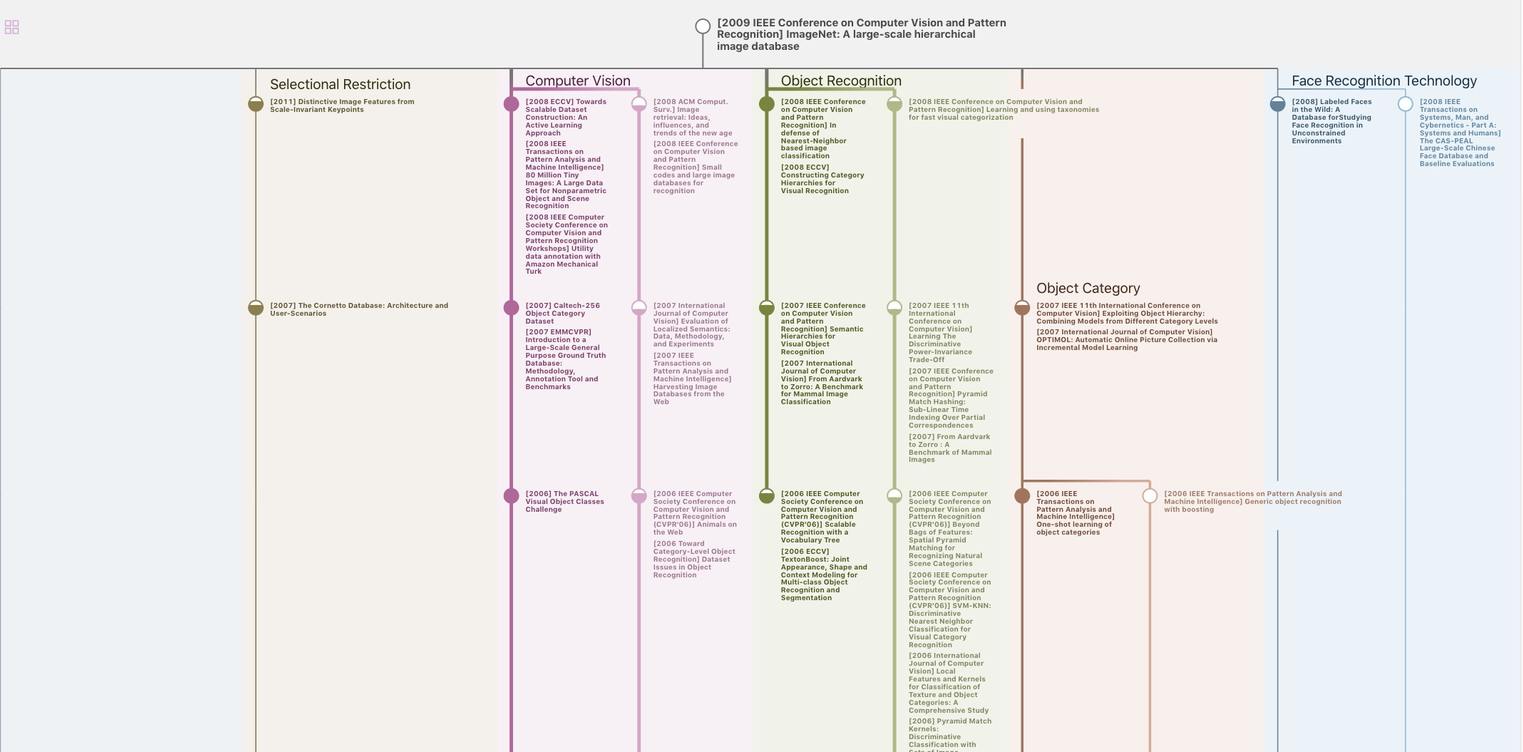
生成溯源树,研究论文发展脉络
Chat Paper
正在生成论文摘要