A Holistic Evaluation of Multivariate Statistical Process Monitoring in a Biological and Membrane Treatment System
ACS ES&T WATER(2023)
摘要
Unsupervised process monitoring for fault detection and data cleaning is underdeveloped for municipal wastewater treatment plants (WWTPs) due to the complexity and volume of data produced by sensors, equipment, and control systems. The goal of this work is to extensively test and tune an unsupervised process monitoring method that can promptly identify faults in a full-scale decentralized WWTP prior to significant system changes. Adaptive dynamic principal component analysis (AD-PCA) is a dimension reduction method modified to address autocorrelation and nonstationarity in multivariate processes and is evaluated in this work for its ability to continuously detect drift, shift, and spike faults. For spike faults, univariate drift faults, and multivariate shift faults, implementing AD-PCA on data that are subset by treatment processes and operating states with significant differences in covariates and whose model parameters use week-long training windows, moderate cumulative variance, and a high threshold for detection was found to detect faults prior to existing operational thresholds. To improve the consistency with which the AD-PCA method detects out-of-control conditions in real time, additional work is needed to remove outliers prior to model fitting and to detect multivariate drift faults in which the covariates change slowly.
更多查看译文
关键词
wastewater treatment,fault detection,sequencing-batch reactor,membrane bioreactor,statistical learning
AI 理解论文
溯源树
样例
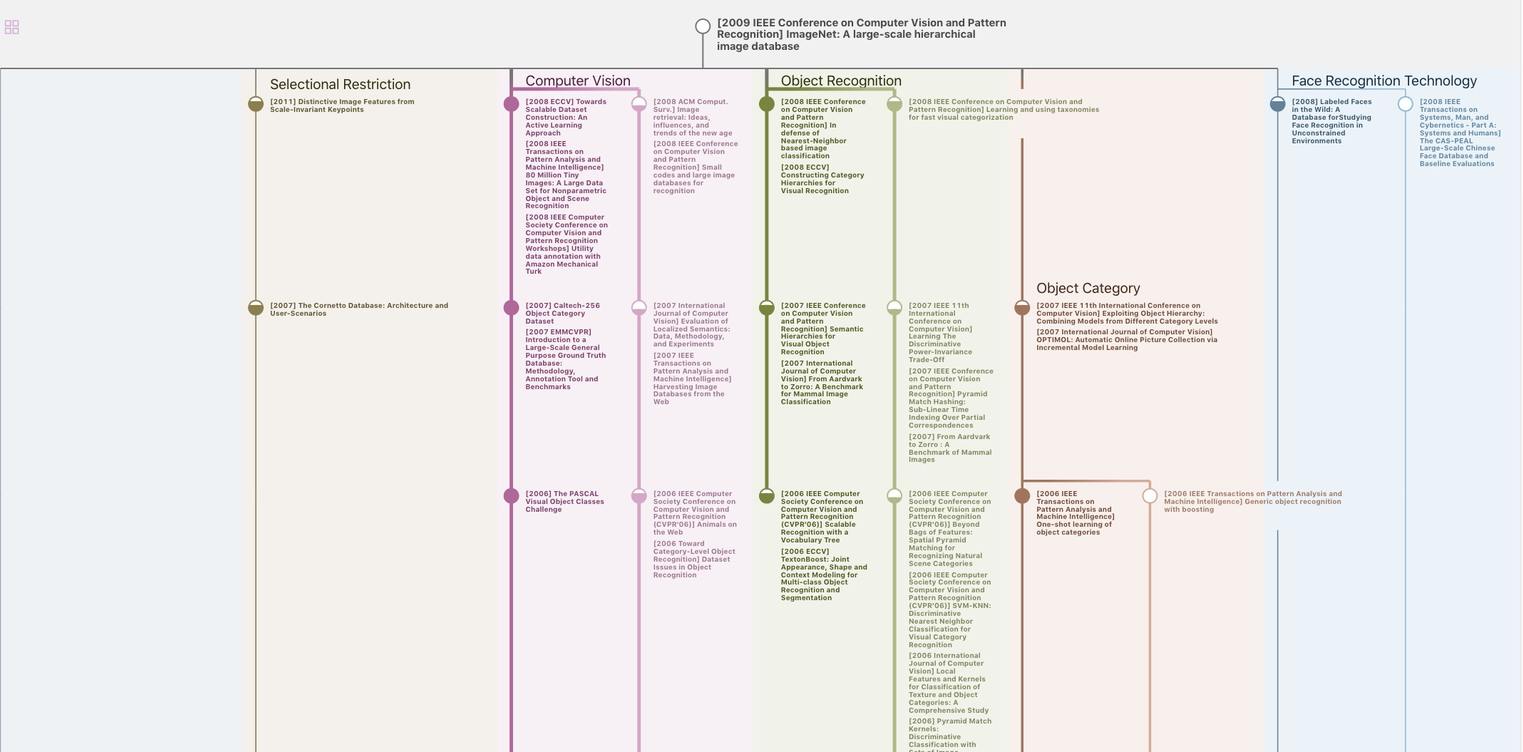
生成溯源树,研究论文发展脉络
Chat Paper
正在生成论文摘要