Multiregional radiomic model for breast cancer diagnosis: value of ultrasound-based peritumoral and parenchymal radiomics
QUANTITATIVE IMAGING IN MEDICINE AND SURGERY(2023)
Abstract
Background: Breast cancer consists not only of neoplastic cells but also of significant changes in the surrounding and parenchymal stroma, which can be reflected in radiomics. This study aimed to perform breast lesion classification through an ultrasound-based multiregional (intratumoral, peritumoral, and parenchymal) radiomic model. Methods: We retrospectively reviewed ultrasound images of breast lesions from institution #1 (n=485) and institution #2 (n=106). Radiomic features were extracted from different regions (intratumoral, peritumoral, and ipsilateral breast parenchymal) and selected to train the random forest classifier with the training cohort (n=339, a subset of the institution #1 dataset). Then, the intratumoral, peritumoral, and parenchymal, intratumoral & peritumoral (In&Peri), intratumoral & parenchymal (In&P), and intratumoral & peritumoral & parenchymal (In&Peri&P) models were developed and validated on the internal (n=146, another subset of institution 1) and external (n=106, institution #2 dataset) test cohorts. Discrimination was evaluated using the area under the curve (AUC). Calibration curve and Hosmer-Lemeshow test assessed calibration. Integrated discrimination improvement (IDI) was used to assess performance improvement. Results: The performance of the In&Peri (AUC values 0.892 and 0.866), In&P (0.866 and 0.863), and In&Peri&P (0.929 and 0.911) models was significantly better than that of the intratumoral model (0.849 and 0.838) in the internal and external test cohorts (IDI test, all P<0.05). The intratumoral, In&Peri and In& Peri& P models showed good calibration (Hosmer-Lemeshow test, all P> 0.05). The multiregional (In&Peri&P) model had the highest discrimination among the 6 radiomic models in the test cohorts, respectively. Conclusions: The multiregional model combining radiomic information of intratumoral, peritumoral, and ipsilateral parenchymal regions yielded better performance than the intratumoral model in distinguishing malignant breast lesions from benign lesions.
MoreTranslated text
Key words
Breast cancer,radiomics,ultrasound,machine learning
AI Read Science
Must-Reading Tree
Example
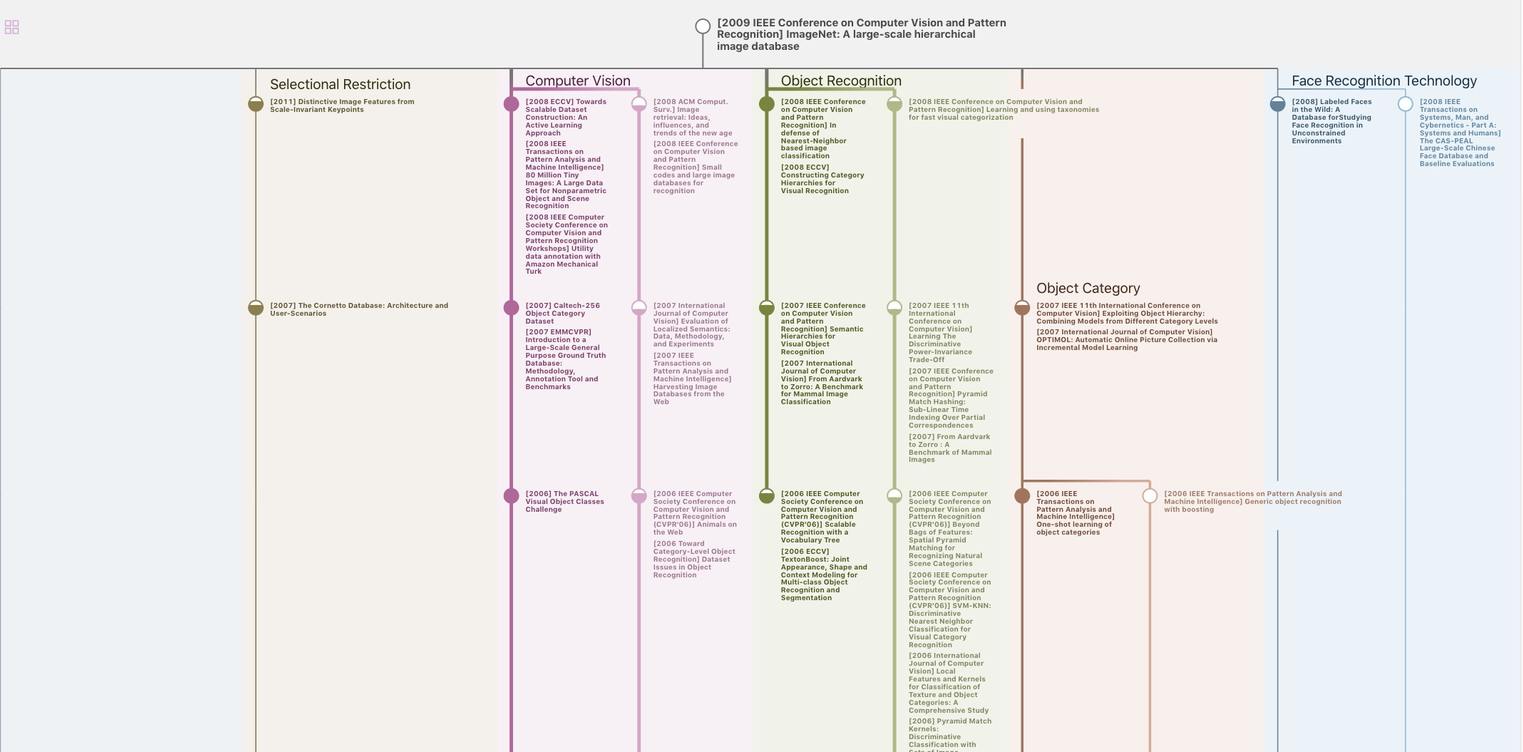
Generate MRT to find the research sequence of this paper
Chat Paper
Summary is being generated by the instructions you defined