Enforcing generalized conditional symmetry in physics-informed neural network for solving the KdV-like equation with Robin initial/boundary conditions
NONLINEAR DYNAMICS(2023)
摘要
In this work, we extend the generalized conditional symmetry enhanced physics-informed neural network (gsPINN) to study the partial differential equations (PDEs) with Robin initial/boundary conditions. The gsPINN incorporates the inherent physical laws, i.e., generalized conditional symmetry of PDEs, into the loss function of PINN and thus learns higher accuracy numerical solutions than PINN with fewer training points and simpler architecture of network. More specifically, we compare the performances of PINN and gsPINN to solve the KdV-like PDEs and show that gsPINN outperforms PINN in terms of the accuracy of learned solutions. Moreover, for the problem of PDEs together with what form of initial/boundary conditions are admitted by the known generalized conditional symmetry, we use the gsPINN method to learn the undetermined functions in Robin initial/boundary conditions and demonstrate the superiorities and robustness of gsPINN over PINN. Our results provide an alternative way for utilizing the deep neural network to study the problems of generalized conditional symmetry of PDEs.
更多查看译文
关键词
Generalized conditional symmetry enhanced PINN,Forward and inverse problems of KdV-like equation,Accuracy of learned solution,Robin initial and boundary problems
AI 理解论文
溯源树
样例
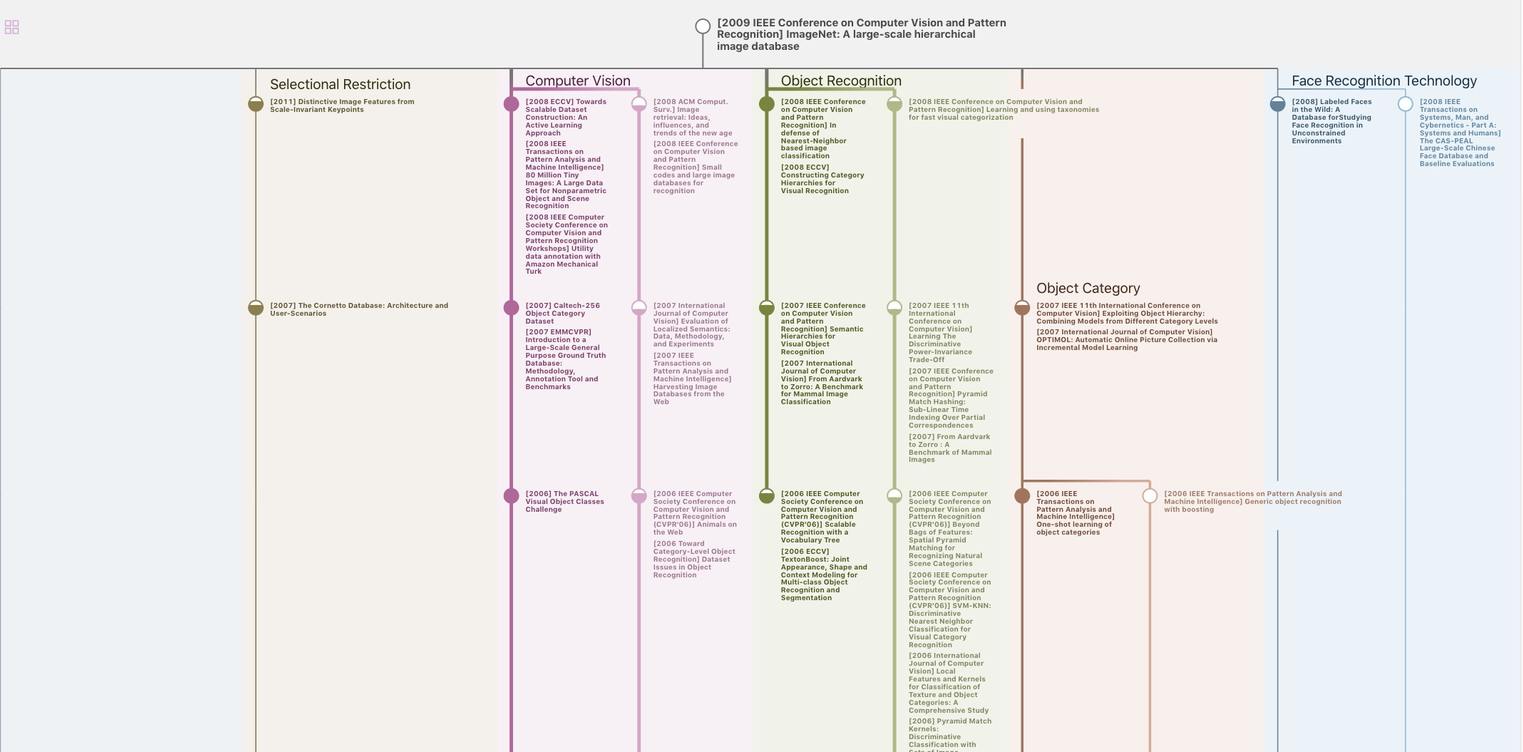
生成溯源树,研究论文发展脉络
Chat Paper
正在生成论文摘要