Evaluating a global soil moisture dataset from a multitask model (GSM3 v1.0)with potential applications for crop threats
GEOSCIENTIFIC MODEL DEVELOPMENT(2023)
Abstract
Climate change threatens our ability to grow food for an ever-increasing population. There is aneed for high-quality soil moisture predictions in under-monitored regionslike Africa. However, it is unclear if soil moisture processes are globallysimilar enough to allow our models trained on available in situ data tomaintain accuracy in unmonitored regions. We present a multitask longshort-term memory (LSTM) model that learns simultaneously from globalsatellite-based data and in situ soil moisture data. This model is evaluated inboth random spatial holdout mode and continental holdout mode (trained onsome continents, tested on a different one). The model compared favorably tocurrent land surface models, satellite products, and a candidate machinelearning model, reaching a global median correlation of 0.792 for the randomspatial holdout test. It behaved surprisingly well in Africa and Australia,showing high correlation even when we excluded their sites from the trainingset, but it performed relatively poorly in Alaska where rapid changes areoccurring. In all but one continent (Asia), the multitask model in theworst-case scenario test performed better than the soil moisture activepassive (SMAP) 9 km product. Factorial analysis has shown that the LSTM model'saccuracy varies with terrain aspect, resulting in lower performance for dryand south-facing slopes or wet and north-facing slopes. This knowledgehelps us apply the model while understanding its limitations. This model isbeing integrated into an operational agricultural assistance applicationwhich currently provides information to 13 million African farmers.
MoreTranslated text
Key words
global soil moisture,multitask model
AI Read Science
Must-Reading Tree
Example
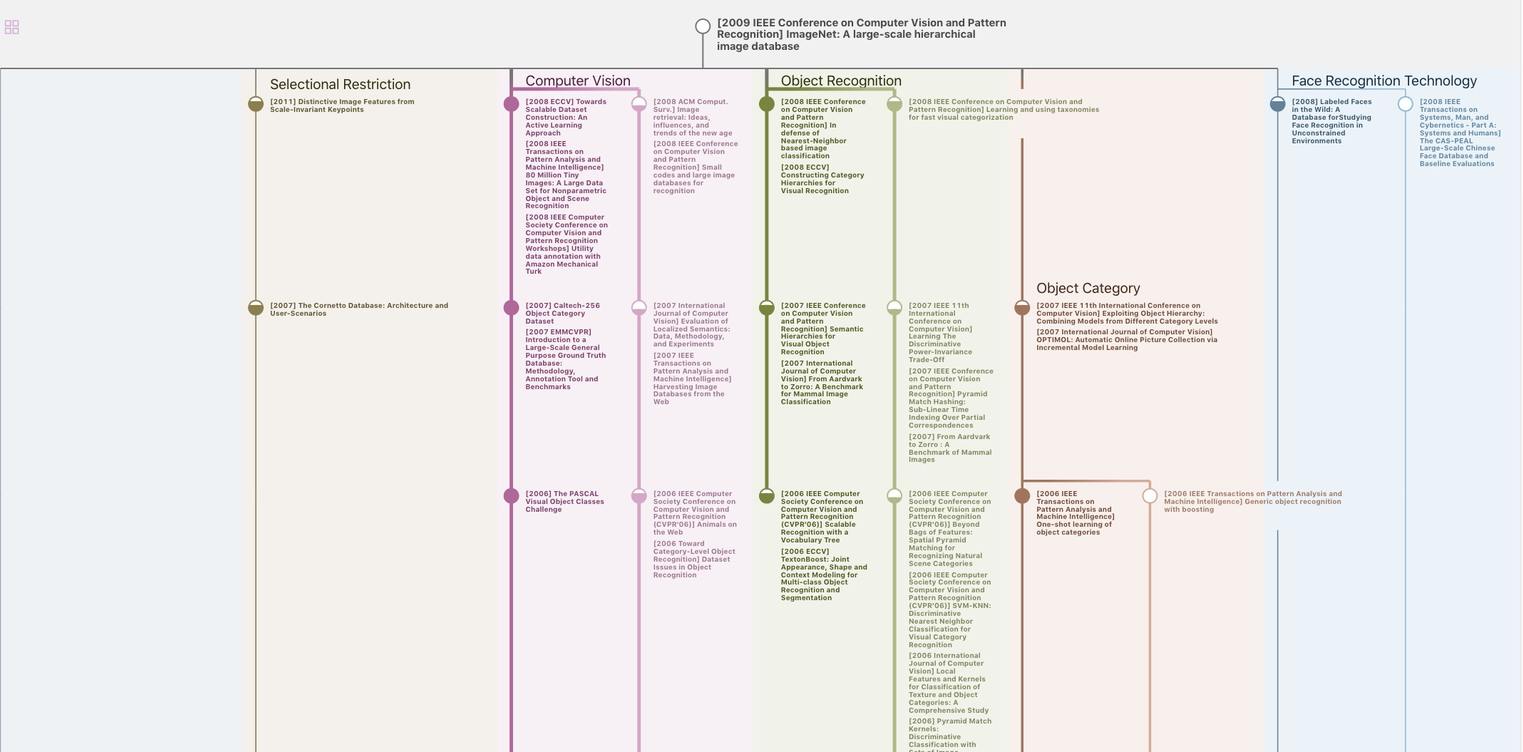
Generate MRT to find the research sequence of this paper
Chat Paper
Summary is being generated by the instructions you defined