Optimized Hierarchical Tree Deep Convolutional Neural Network of a Tree-Based Workload Prediction Scheme for Enhancing Power Efficiency in Cloud Computing
ENERGIES(2023)
Abstract
Workload prediction is essential in cloud data centers (CDCs) for establishing scalability and resource elasticity. However, the workload prediction accuracy in the cloud data center could be better due to noise, redundancy, and low performance for workload prediction. This paper designs a hierarchical tree-based deep convolutional neural network (T-CNN) model with sheep flock optimization (SFO) to enhance CDCs' power efficiency and workload prediction. The kernel method is used to preprocess historical information from the CDCs. Additionally, T-CNN model weight parameters are optimized using SFO. The suggested TCNN-SFO technology has successfully reduced excessive power consumption while correctly forecasting the incoming demand. Further, the proposed model is assessed using two benchmark datasets: Saskatchewan HTTP traces and NASA. The developed model is executed in a Java tool. Therefore, associated with existing methods, the developed technique has achieved higher accuracy of 20.75%, 19.06%, 29.09%, 23.8%, and 20.5%, as well as lower energy consumption of 20.84%, 18.03%, 28.64%, 30.72%, and 33.74% when validating the Saskatchewan HTTP traces dataset. It has also achieved higher accuracy of 32.95%, 12.05%, 32.65%, and 26.54%.
MoreTranslated text
Key words
cloud data center,cloud computing,convolutional neural network,sheep flock optimization,workload prediction,kernel correlation
AI Read Science
Must-Reading Tree
Example
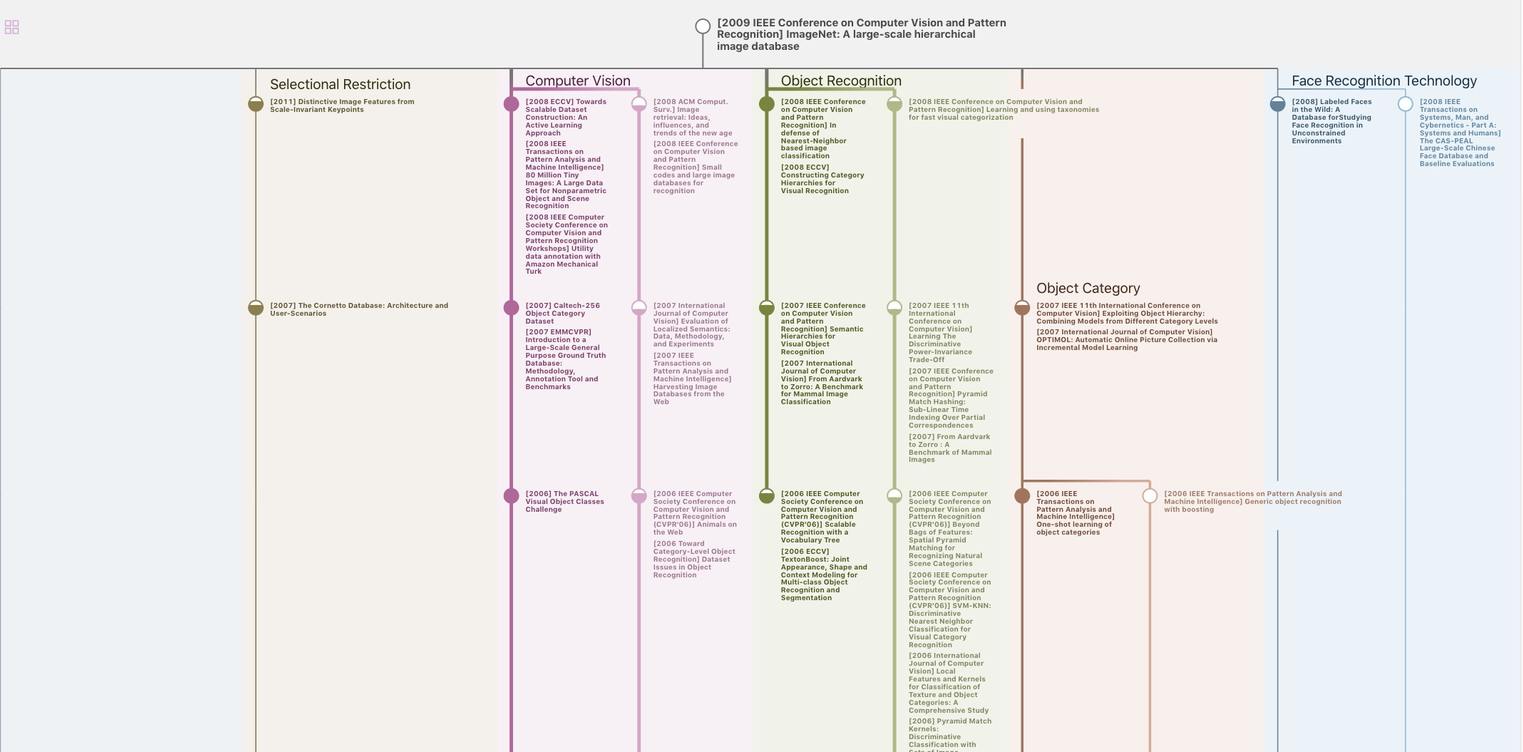
Generate MRT to find the research sequence of this paper
Chat Paper
Summary is being generated by the instructions you defined