CAM-CAN: Class activation map-based categorical adversarial network
EXPERT SYSTEMS WITH APPLICATIONS(2023)
Abstract
Numerous studies have investigated image classification. In particular, recent methods based on deep learning have exhibited high accuracies. However, various existing state-of-the-art methods based on deep learning show different accuracies depending on the database and environment. Accordingly, different deep learning models need to be used in image classification studies according to the database, environment, and research field. This study investigated a technique to increase the accuracy of the existing deep learning-based models. The proposed method was applied to various existing state-of-the-art methods. In the proposed method, a convolution neural network (CNN) is trained using the classification activation map (CAM) to focus on specific areas in the input image. The CAM image is used as the ground-truth image. Furthermore, the concept of the CAM-based cate-gorical adversarial network (CAM-CAN), in which the CNN is trained based on a generative adversarial network, is proposed in this paper. An action recognition experiment was performed using the self-collected Dongguk thermal image database (DTh-DB) and open database, and the results revealed that the accuracies of the existing state-of-the-art methods significantly increased after applying the proposed method. For instance, the accuracies obtained using the DTh-DB, TPR, PPV, ACC, and F1 with the conventional DenseNet201 model were 80.14%, 75.28%, 96.0%, and 75.91%, respectively. After applying the proposed method, the accuracies increased to 86.53%, 89.90%, 97.64%, and 85.84%, respectively.
MoreTranslated text
Key words
Deep learning,CNN,CAM-CAN,GAN,Action recognition
AI Read Science
Must-Reading Tree
Example
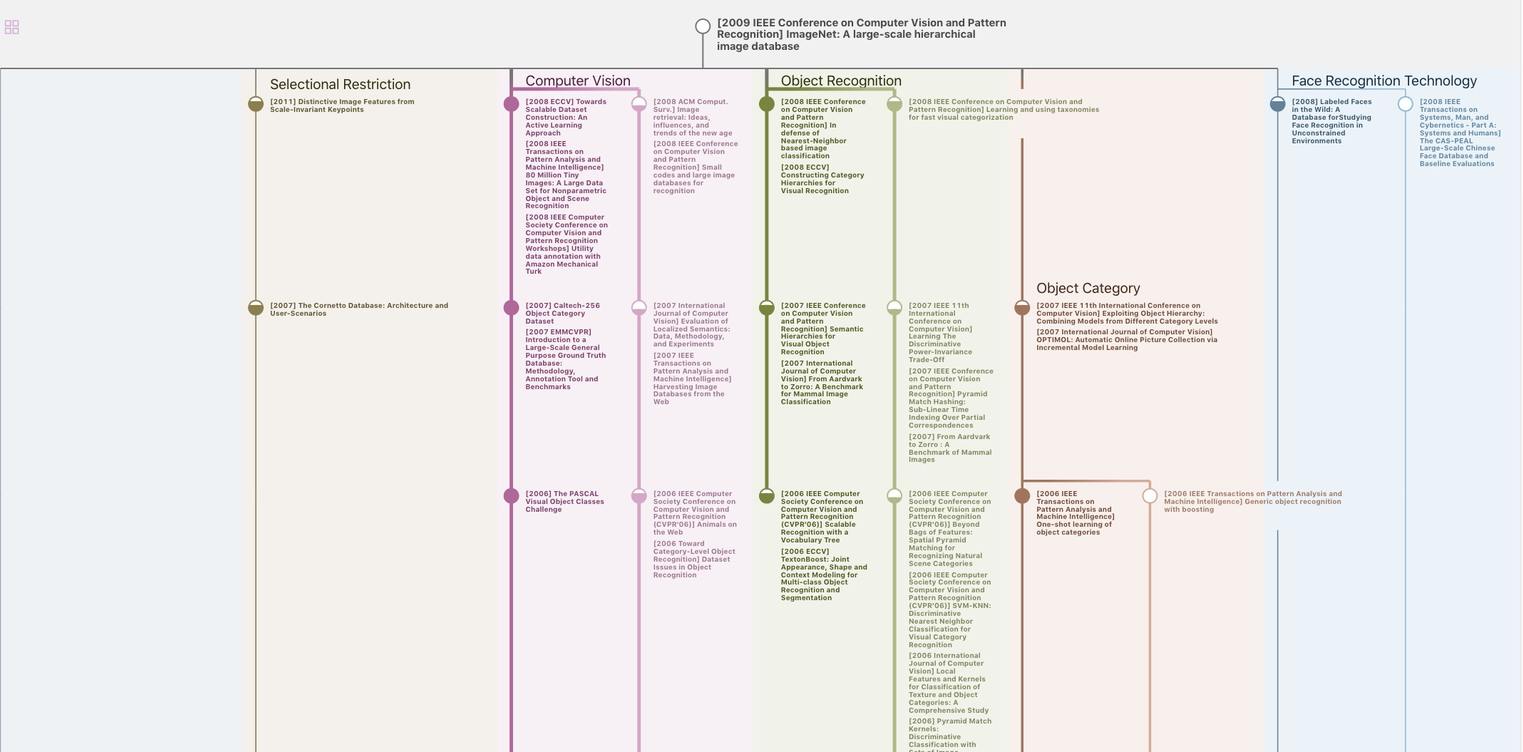
Generate MRT to find the research sequence of this paper
Chat Paper
Summary is being generated by the instructions you defined