ARWLS-AFEKE: SOC Estimation and Capacity Correction of Lithium Batteries Based on a Fusion Algorithm
PROCESSES(2023)
Abstract
Accuracy of battery charge status (SOC) estimation plays a significant role in the management of electric vehicle power batteries. However, recently, abrupt changes from SOC data often occurs in the actual operation of electric vehicles and some errors appear in the establishment of battery models and noise models, which give rise to the poorly adaptive and robust performance of traditional algorithms in the process of SOC estimation. The fusion algorithm proposed in this paper can effectively improve the accuracy of models and SOC estimation of lithium-ion batteries. Based on the second-order R-C network model, this method optimizes the accuracy of parameter identification by adopting the adaptive recursive weighted least square algorithm (ARWLS). In addition, the adaptive fading extended Kalman filter algorithm (AFEKF) is applied to estimate the SOC of lithium-ion batteries. Additionally, via introducing a fading factor, the optimal Kalman gain is updated in real-time, which can reduce the impact of data mutation on battery modeling. Compared with the offline AEKF algorithm and the EKF algorithm, the adaptive recursive weighted least square-adaptive fading extended Kalman filter (ARWLS-AFEKF) fusion algorithm had higher accuracy and adaptability, which can be adapted to the variable noise environment.
MoreTranslated text
Key words
SOC,capacity correction,AFEKF,ARWLS,battery equivalent model
AI Read Science
Must-Reading Tree
Example
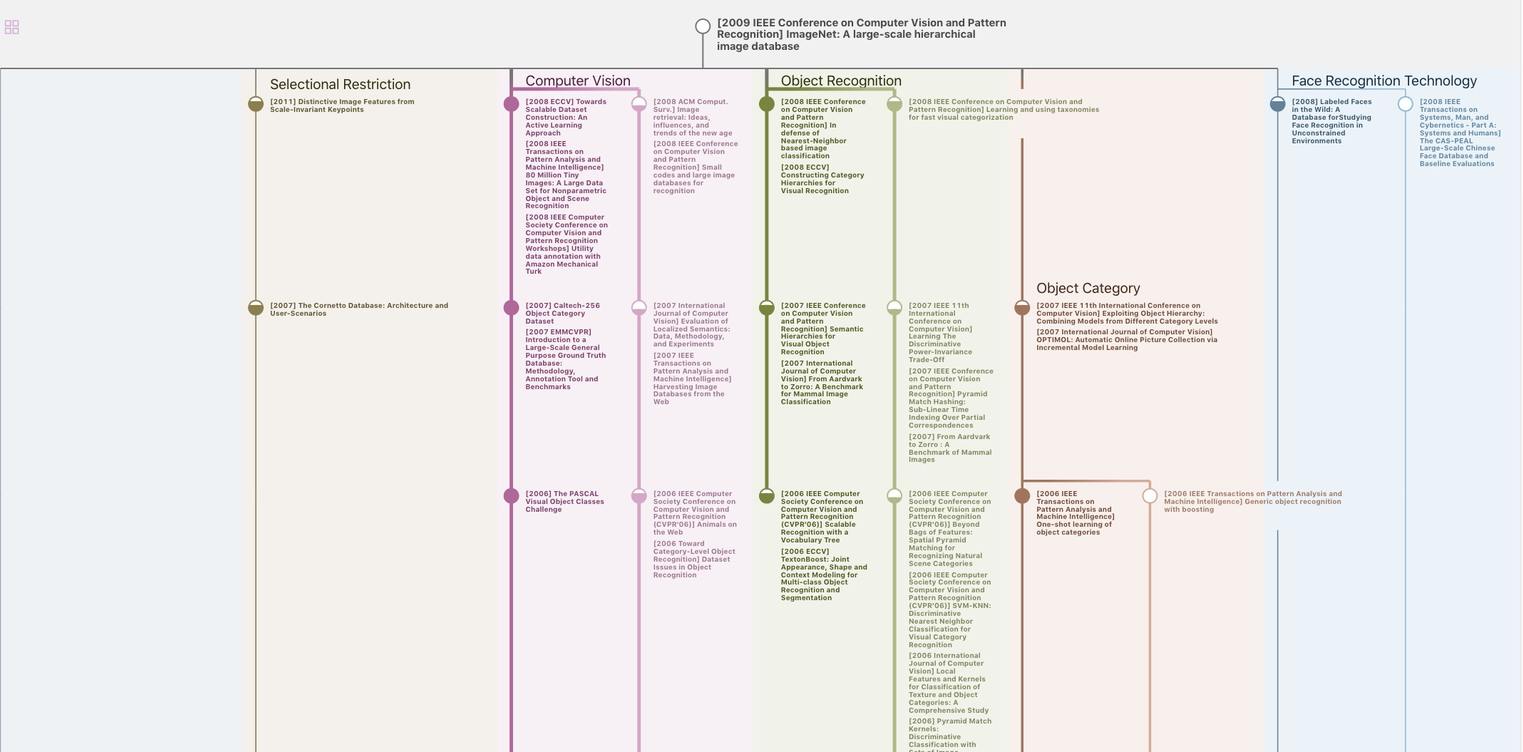
Generate MRT to find the research sequence of this paper
Chat Paper
Summary is being generated by the instructions you defined