BarrierNet: Differentiable Control Barrier Functions for Learning of Safe Robot Control
IEEE TRANSACTIONS ON ROBOTICS(2023)
摘要
Many safety-critical applications of neural networks, such as robotic control, require safety guarantees. This article introduces a method for ensuring the safety of learned models for control using differentiable control barrier functions (dCBFs). dCBFs are end-to-end trainable and guarantee safety. They improve over classical control barrier functions (CBFs), which are usually overly conservative. Our dCBF solution relaxes the CBF definitions by: 1) using environmental dependencies; 2) embedding them into differentiable quadratic programs. These novel safety layers are called a BarrierNet. They can be used in conjunction with any neural network-based controller. They are trained by gradient descent. With BarrierNet, the safety constraints of a neural controller become adaptable to changing environments. We evaluate BarrierNet on the following several problems: 1) robot traffic merging; 2) robot navigation in 2-D and 3-D spaces; 3) end-to-end vision-based autonomous driving in a sim-to-real environment and in physical experiments; 4) demonstrate their effectiveness compared to state-of-the-art approaches.
更多查看译文
关键词
Safety,Robots,Autonomous vehicles,Neural networks,Vehicle dynamics,Uncertainty,Robot sensing systems,Control barrier function (CBF),neural networks,robot learning,safety guarantees
AI 理解论文
溯源树
样例
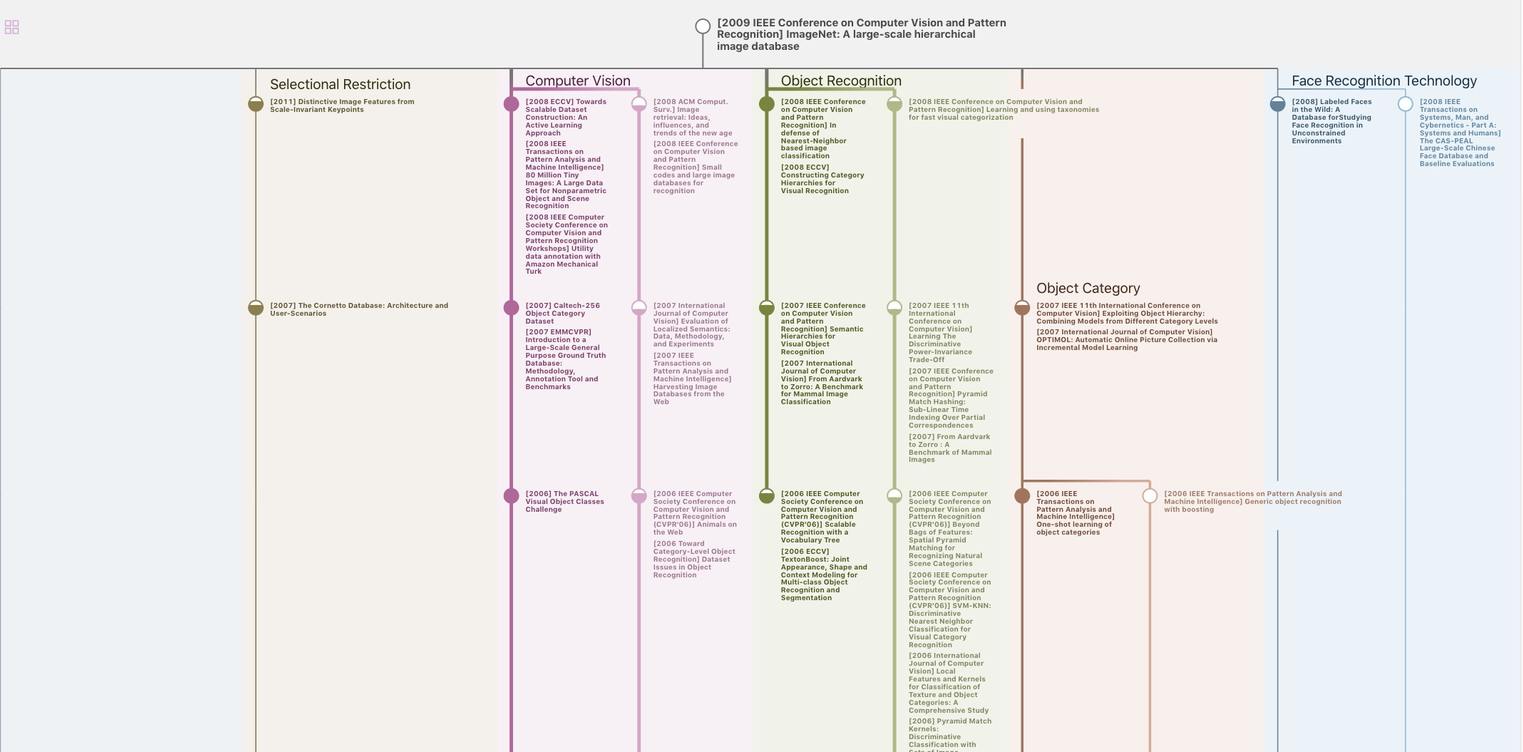
生成溯源树,研究论文发展脉络
Chat Paper
正在生成论文摘要