Low-carbon optimal learning scheduling of the power system based on carbon capture system and carbon emission flow theory
ELECTRIC POWER SYSTEMS RESEARCH(2023)
摘要
In the context of the current low-carbon environment initiatives and the construction of modern power systems, how to achieve an energy-saving and low-carbon system while ensuring rational scheduling is an urgent issue to be addressed. In view of the fact that few studies has integrated carbon emission flows with power flow to conduct study on power dispatch and the intelligence of the solution algorithm needs to be further explored, this study proposed a low-carbon optimal learning and scheduling method for the power system that takes into account the carbon capture system and the carbon emission flow theory. Firstly, the carbon emission flow model of the power system was constructed at the equipment level and the system level respectively. Secondly, a bi-level alternating optimal scheduling model, which includes system day-ahead scheduling and load demand response adjustment, was established with all aspects of the power system (source, grid, load and storage) considered, and this model was solved by a deep deterministic policy gradient algorithm based on the deep reinforcement learning actor-critic framework. The simulations of actual grid structures shows that the theo-retical scheduling method proposed in this study is effective in improving overall operation economic efficiency, and the deep reinforcement learning method adopted demonstrates certain advantages in the iterative conver-gence process and accurate determination of solution space.
更多查看译文
关键词
Carbon capture,Carbon flow,Operational scheduling,Deep reinforcement learning,Demand response
AI 理解论文
溯源树
样例
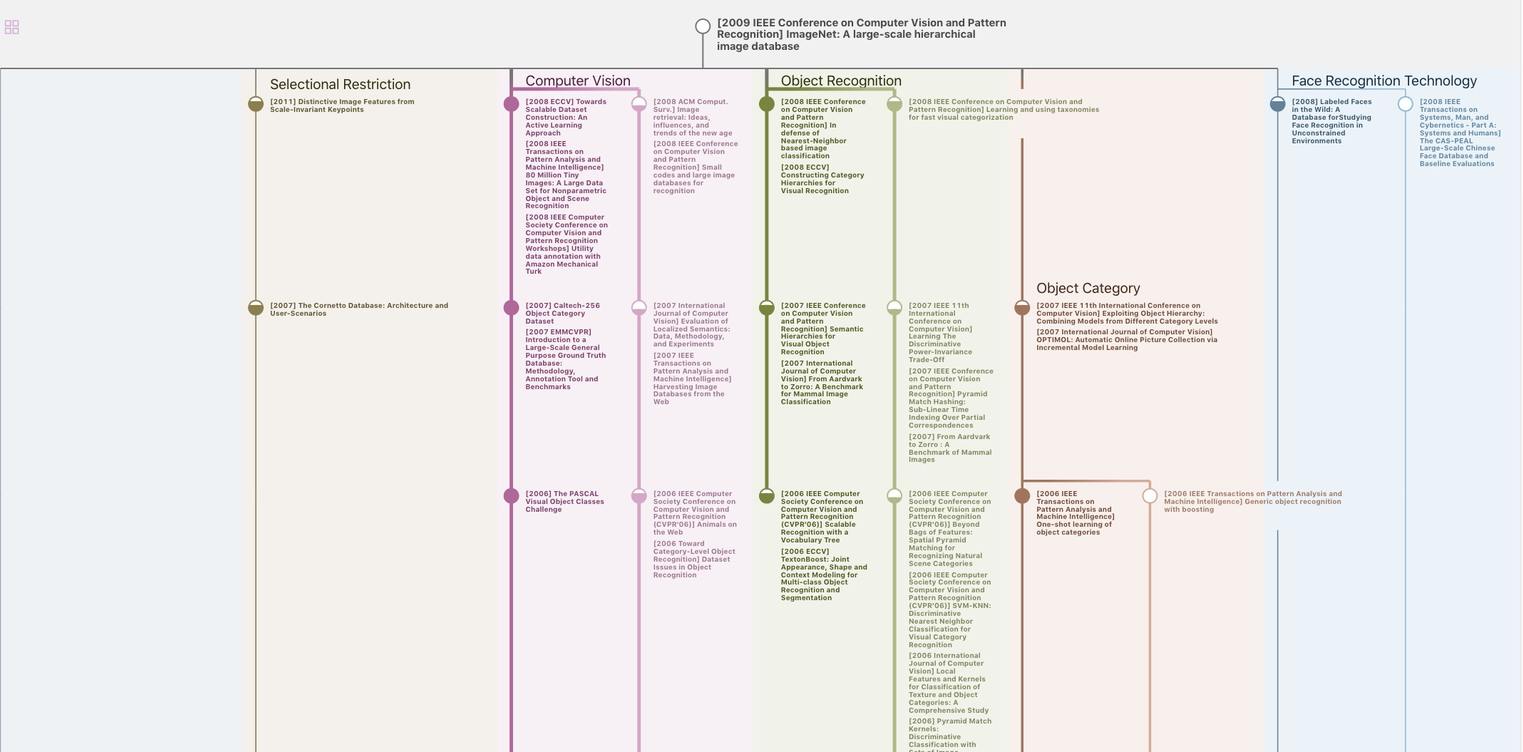
生成溯源树,研究论文发展脉络
Chat Paper
正在生成论文摘要