Long-Lived Particles Anomaly Detection with Parametrized Quantum Circuits
PARTICLES(2023)
摘要
We investigate the possibility to apply quantum machine learning techniques for data analysis, with particular regard to an interesting use-case in high-energy physics. We propose an anomaly detection algorithm based on a parametrized quantum circuit. This algorithm was trained on a classical computer and tested with simulations as well as on real quantum hardware. Tests on NISQ devices were performed with IBM quantum computers. For the execution on quantum hardware, specific hardware-driven adaptations were devised and implemented. The quantum anomaly detection algorithm was able to detect simple anomalies such as different characters in handwritten digits as well as more complex structures such as anomalous patterns in the particle detectors produced by the decay products of long-lived particles produced at a collider experiment. For the high-energy physics application, the performance was estimated in simulation only, as the quantum circuit was not simple enough to be executed on the available quantum hardware platform. This work demonstrates that it is possible to perform anomaly detection with quantum algorithms; however, as an amplitude encoding of classical data is required for the task, due to the noise level in the available quantum hardware platform, the current implementation cannot outperform classic anomaly detection algorithms based on deep neural networks.
更多查看译文
关键词
particle physics,long-lived particles,anomaly detection,quantum computing,machine learning,quantum machine learning,parametrized quantum circuits
AI 理解论文
溯源树
样例
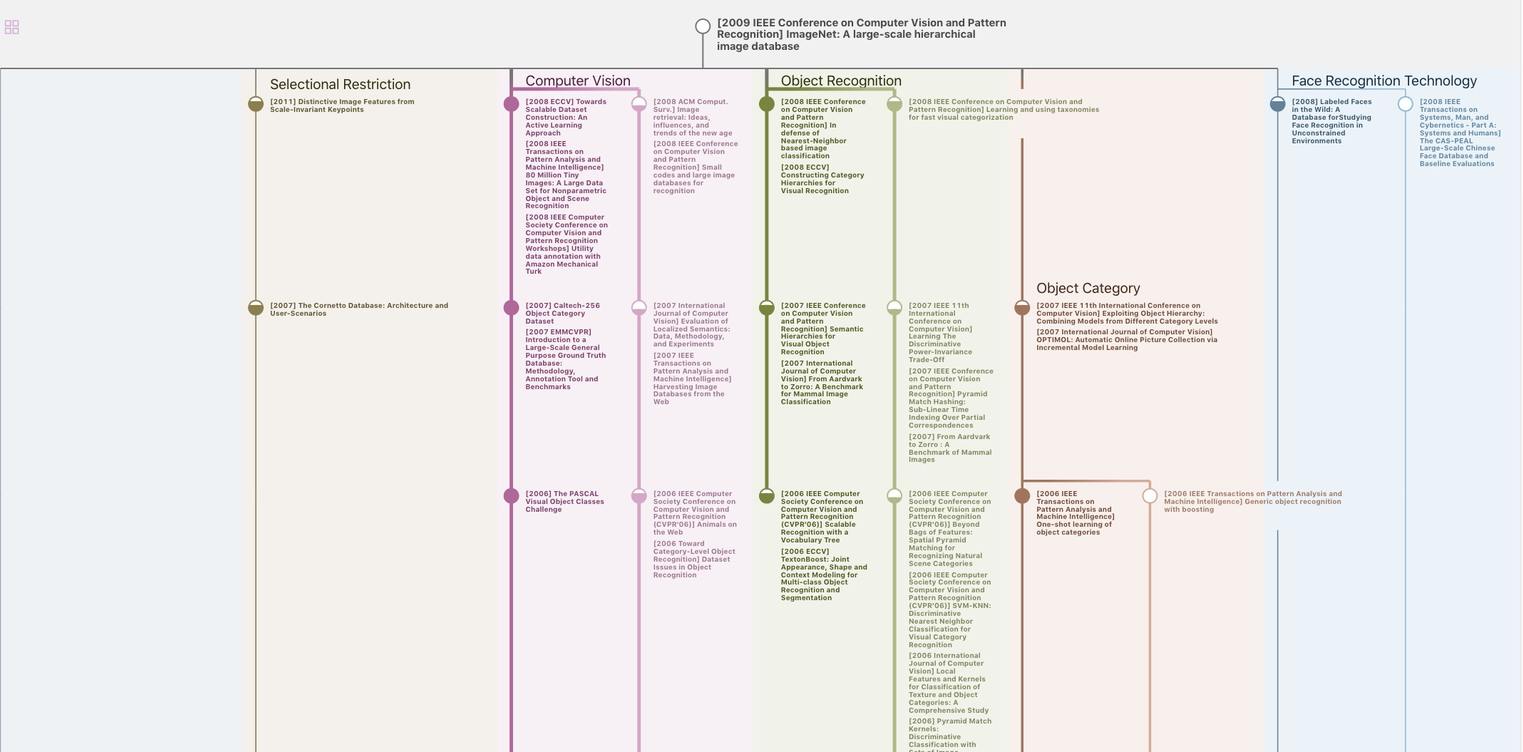
生成溯源树,研究论文发展脉络
Chat Paper
正在生成论文摘要