Compiler Optimization Prediction with New Self-Improved Optimization Model
INTERNATIONAL JOURNAL OF ADVANCED COMPUTER SCIENCE AND APPLICATIONS(2023)
摘要
Users may now choose from a vast range of compiler optimizations. These optimizations interact in a variety of sophisticated ways with one another and with the source code. The order in which optimization steps are applied can have a considerable influence on the performance obtained. As a result, we created a revolutionary compiler optimization prediction model. Our model comprises three operational phases: model training, feature extraction, as well as model exploitation. The model training step includes initialization as well as the formation of candidate sample sets. The inputs were then sent to the feature extraction phase, which retrieved static, dynamic, and improved entropy features. These extracted features were then optimized by the feature exploitation phase, which employs an improved hunger games search algorithm to choose the best features. In this work, we used a Convolutional Neural Network to predict compiler optimization based on these selected characteristics, and the findings show that our innovative compiler optimization model surpasses previous approaches.
更多查看译文
关键词
Compiler optimization prediction,feature extraction,feature exploitation,improved hunger games search algorithm,convolutional neural network
AI 理解论文
溯源树
样例
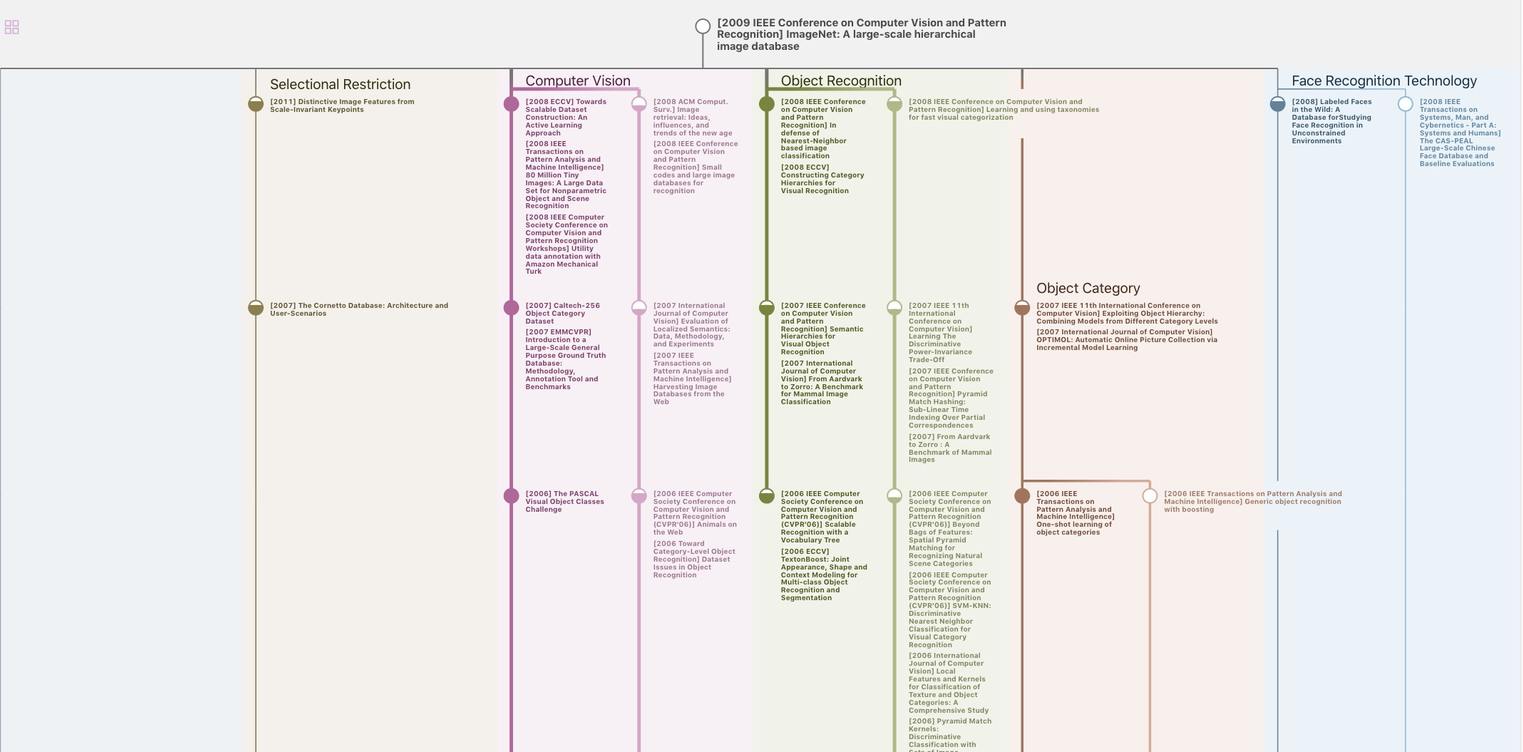
生成溯源树,研究论文发展脉络
Chat Paper
正在生成论文摘要