Estimating high-spatial-resolution daily PM2.5 mass concentration from satellite top-of-atmosphere reflectance based on an improved random forest model
ATMOSPHERIC ENVIRONMENT(2023)
Abstract
The aerosol optical depth (AOD) has been widely used to estimate ground PM2.5 mass concentration in the field of remote sensing, but the current AOD retrieval results always contained invalid AOD values due to the limi-tations of different algorithms, which is not conducive to obtaining daily estimations of PM2.5 mass concentra-tion. This study used the top-of-atmosphere (TOA) reflectance to establish a relationship with ground PM2.5 mass concentration instead of using traditional AOD product data. We developed an improved multiple-factor random forest model (IMFRFM) by integrating TOA reflectance, meteorological data, and land-use data to estimate high -spatial-resolution (1-km x 1-km) daily PM2.5 mass concentration in the Pearl River Delta (PRD) region. The simulation results showed that our model had excellent performance (test-R2 = 0.88, RMSE = 9.20 mu g/m3, and MAE = 2.48 mu g/m3) with city-based and time-based cross-validation (CV) test-R2 values of 0.82 and 0.88, respectively. The four-year (2017-2020) seasonal results showed that the PM2.5 mass concentration of the PRD region exhibited obvious seasonality, with the highest values in winter and lowest values in summer. The four-year annual results showed that the PM2.5 mass concentration in the PRD region decreased year by year. The annual average PM2.5 mass concentration in most cities in the PRD region were below 40 mu g/m3, indicating that the atmospheric environment of the PRD region was gradually improving.
MoreTranslated text
Key words
PM 2,5 mass concentration,TOA reflectance,Random forest model (RFM),Pearl River Delta (PRD)
AI Read Science
Must-Reading Tree
Example
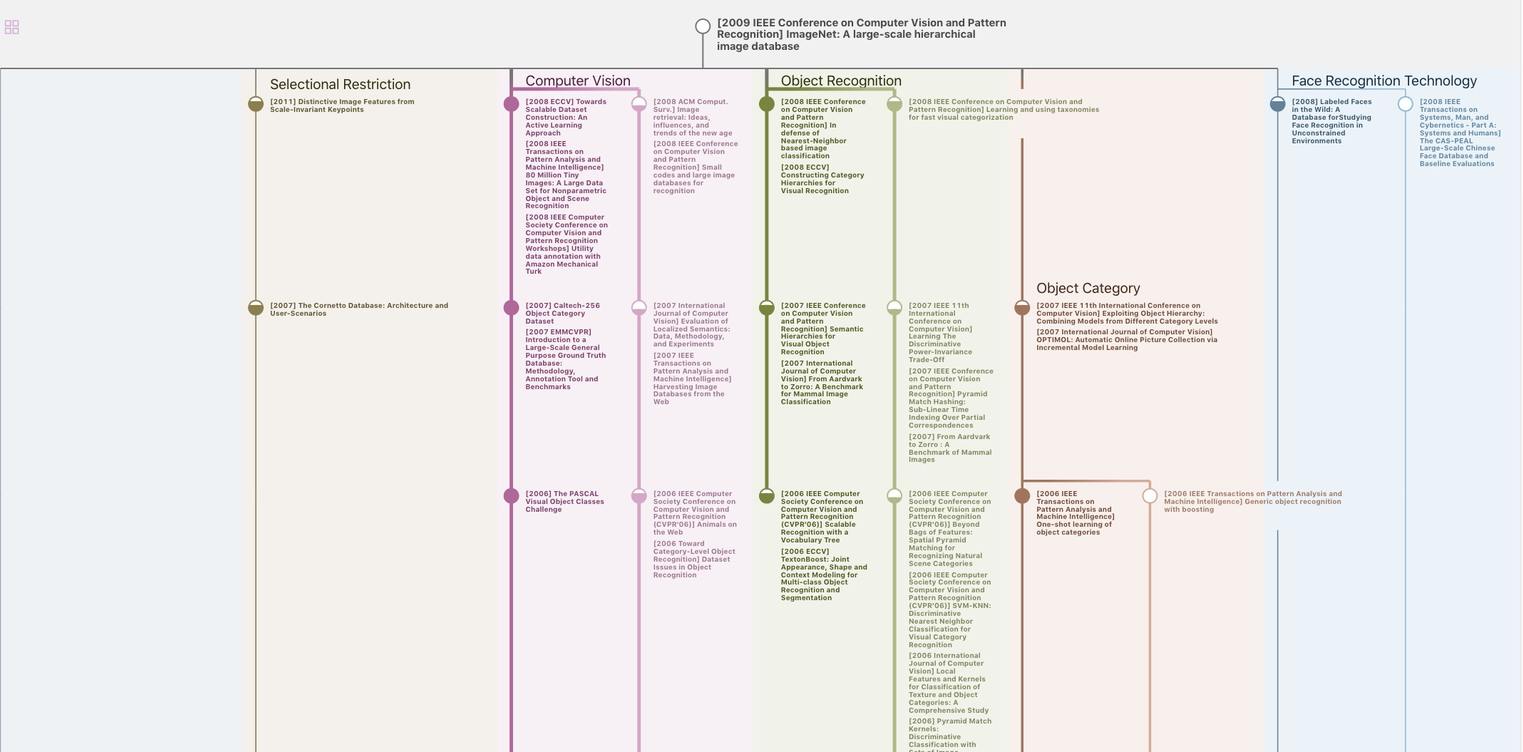
Generate MRT to find the research sequence of this paper
Chat Paper
Summary is being generated by the instructions you defined