A two stage approach to electricity theft detection in AMI using deep learning
INTERNATIONAL JOURNAL OF ELECTRICAL POWER & ENERGY SYSTEMS(2023)
摘要
Electricity fraud as a noteworthy portion of non-technical losses has continuously been a worldwide concern. On the other hand, once a fraud is detected, on-site inspection is necessary for final verification. Since inspecting all metering data is laborious and expensive, utilities constantly try to narrow the inspection to instances with a higher odds of fraud. The rise of smart meters has introduced AI-based methods to reduce the inspection's scope by leveraging consumption patterns. However, their performances are limited due to the insufficiency of mali-cious samples, known as data imbalance. In the following investigation, a two-stage deep-learning-based model is provided for detecting energy fraud using metering data. first, a deep architecture is developed to model sus-picious behaviour of fraudulent customers; which is used to handle the problem of data imbalance by predicting possible theft scenarios for normal consumers. then, A DNN network is developed to distinguishes normal and suspicious consumers. Consideration use of clustering and deep learning, as well as concurrent use of con-sumption and livelihood data, has enabled the algorithm to understand customer's behaviour and not fall against non-malicious changes in consumption patterns. Evaluation of the following method on a repository with 6000 real-world metering data demonstrates its high performance.
更多查看译文
关键词
Advanced metering infrastructure (AMI),Deep learning,Energy theft,Non-technical losses (NTL),Smart grid
AI 理解论文
溯源树
样例
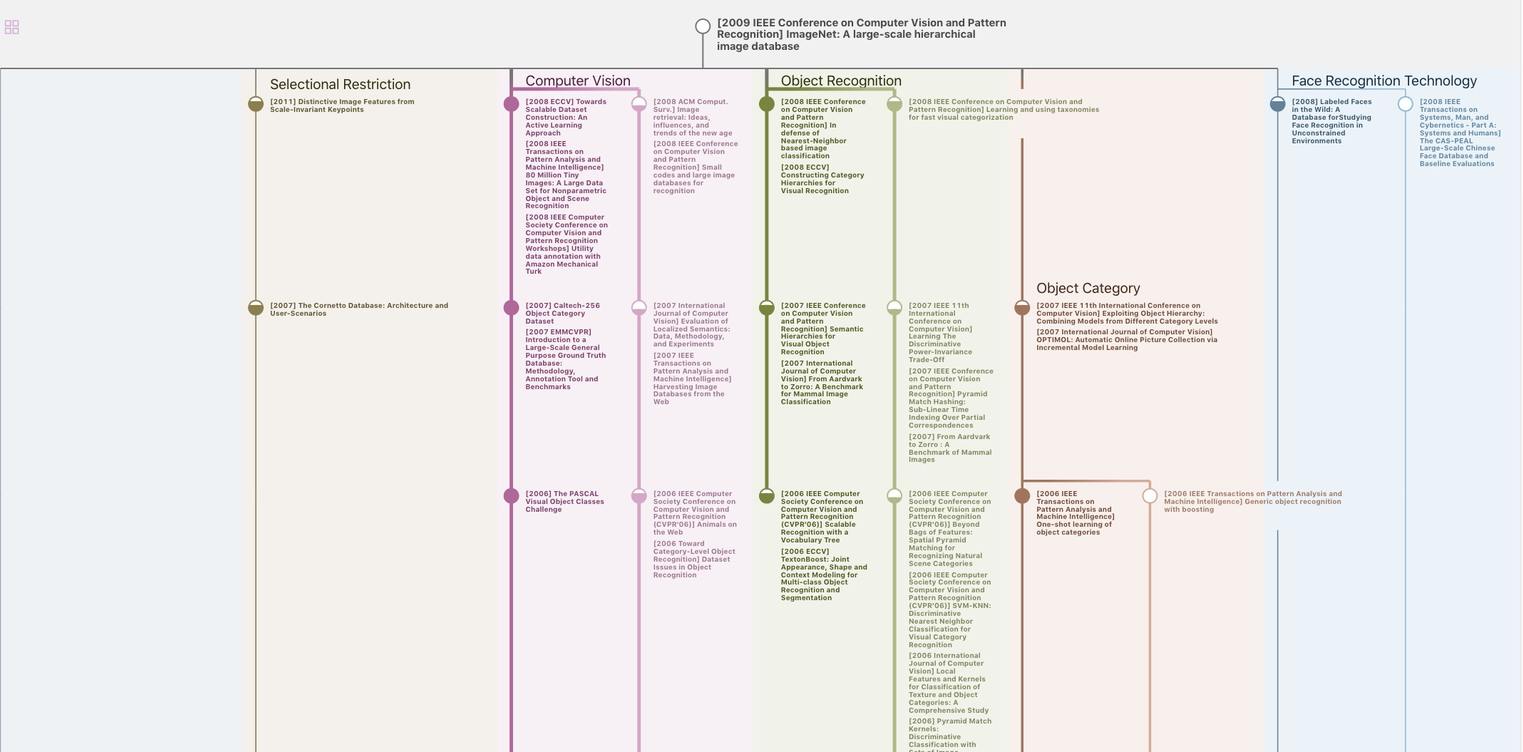
生成溯源树,研究论文发展脉络
Chat Paper
正在生成论文摘要