Estimating individual-level pig growth trajectories from group-level weight time series using machine learning
COMPUTERS AND ELECTRONICS IN AGRICULTURE(2023)
摘要
Recent years have seen an increase in the use of advanced data collection systems to improve management in pig farms, however, the cost of implementing such systems remains a barrier to many. Some of the most useful data for farm management are on an individual pig level, which requires investment of labour and expense of systems such as RFID tagging. In this paper an alternative solution is proposed, where pen-level pig weight measurements (i.e. measurements not linked to a specific pig identity), which are significantly cheaper to obtain, are used to estimate individual-level growth trajectories using machine learning. We evaluated our method on group-level data that were simulated from individual-level weight data, which thus allowed for the verification of the accuracy of the trajectory predictions using a root mean squared error score. The on-average best performing predictor for this task was based on a Random Forest model which achieved a best-case score of 2.00 Kg per pig and a worst-case score of 2.45 Kg per pig depending upon the simulated conditions that were used, thus showing that these predicted trajectories could be accurate enough for commercial usage. The usefulness of the predicted trajectories was then evaluated through a growth prediction downstream task whereby the future weights of pigs two weeks into the future were predicted using a variety of machine learning models. It was found that, on average, the growth prediction models that utilised the predicted trajectories outperformed a method that utilised group-level weights directly by 1.42 Kg root mean squared error but were still outperformed by the models that utilised RFID growth trajectories by 0.83 Kg on average. These results show the potential of this method as an alternative to both RFID and photo-based systems for individual-level data estimation.
更多查看译文
关键词
Machine learning,Precision livestock farming,Sustainable food production
AI 理解论文
溯源树
样例
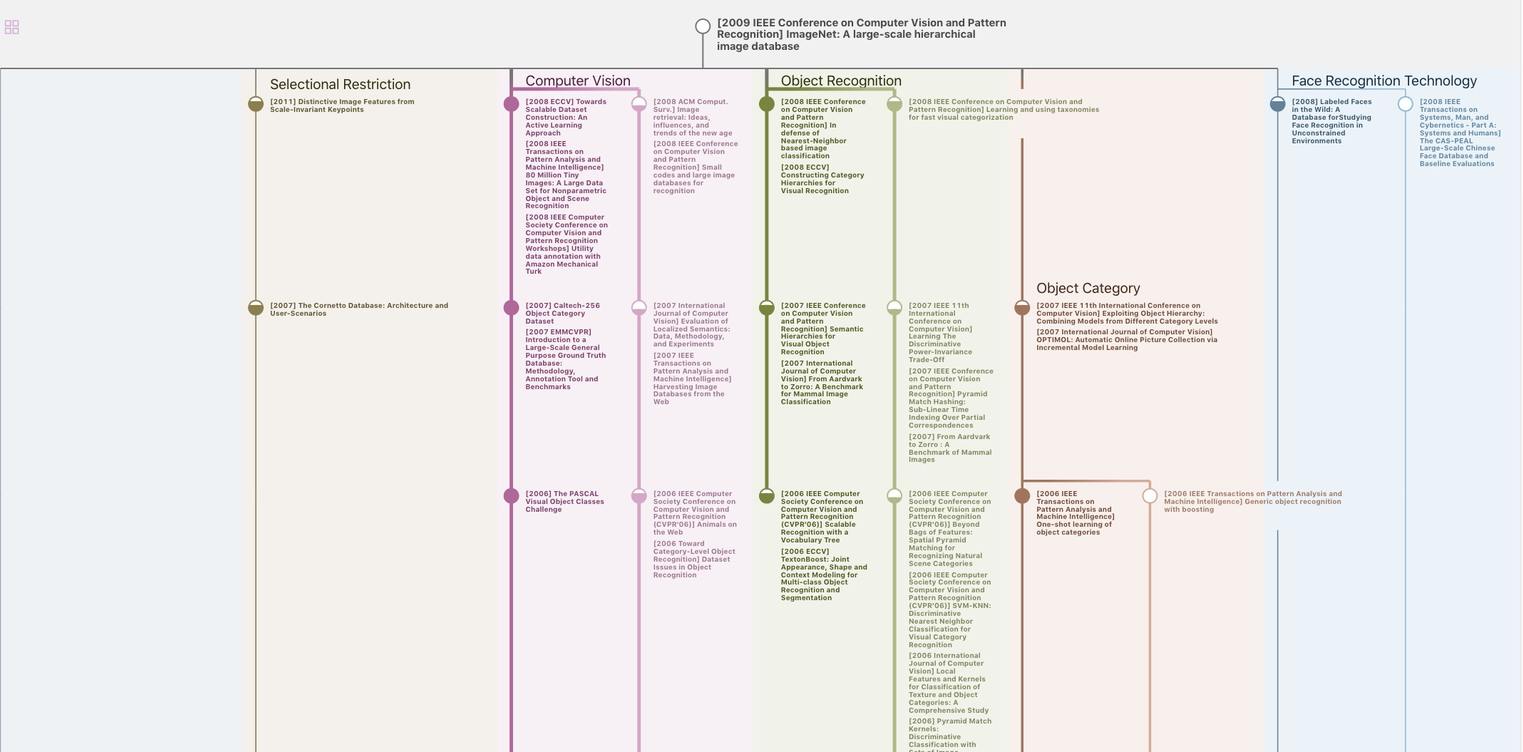
生成溯源树,研究论文发展脉络
Chat Paper
正在生成论文摘要