Privacy and Accuracy for Cloud-Fog-Edge Collaborative Driver-Vehicle-Road Relation Graphs
IEEE TRANSACTIONS ON INTELLIGENT TRANSPORTATION SYSTEMS(2023)
摘要
There are three key roles in Intelligent Transportation Systems (ITS): driver, vehicle and road. However, existing static interactions among Driver-Vehicle-Road (DVR) are too passive to reflect the change of driver preferences, vehicle conditions, road conditions, etc. Therefore, we provide a data-driven Cloud-Fog-Edge Collaborative Driver-Vehicle-Road (CFEC-DVR) framework. The framework could self-adaptively evolves through continuous iteration to provide better ITS services for humans. The collaboration among DVR creates a lot of relation data that construct our relation graphs. Cloud brings some privacy risks. Relation graphs have great analytic value. As DVR collaboration, privacy quality and analytic accuracy are three key issues in the framework, we propose a Relation Graph Privacy-Preserving scheme with High Accuracy in our framework, which is named as RGPP-HA. Based on machine learning, our method nearly maximizes the difficulty for attackers to know exactly how many other roles are connected to the attacked role, which enhances the privacy quality. Meanwhile, we find as much valuable information as possible from roles' encrypted relations for more accurately analytic performance. Based on the experiments, we compare the proposed scheme RGPP-HA with existing classic and relevant schemes. The experimental results show that our scheme has the best privacy quality and analytic accuracy. This further verifies the feasibility of CFEC-DVR framework.
更多查看译文
关键词
Vehicles,Privacy,Roads,Collaboration,Data privacy,Computer science,Monitoring,Cloud-fog-edge collaborative driver-vehicle-road framework,relation graphs,privacy quality,analytic accuracy,self-adaptive evolution
AI 理解论文
溯源树
样例
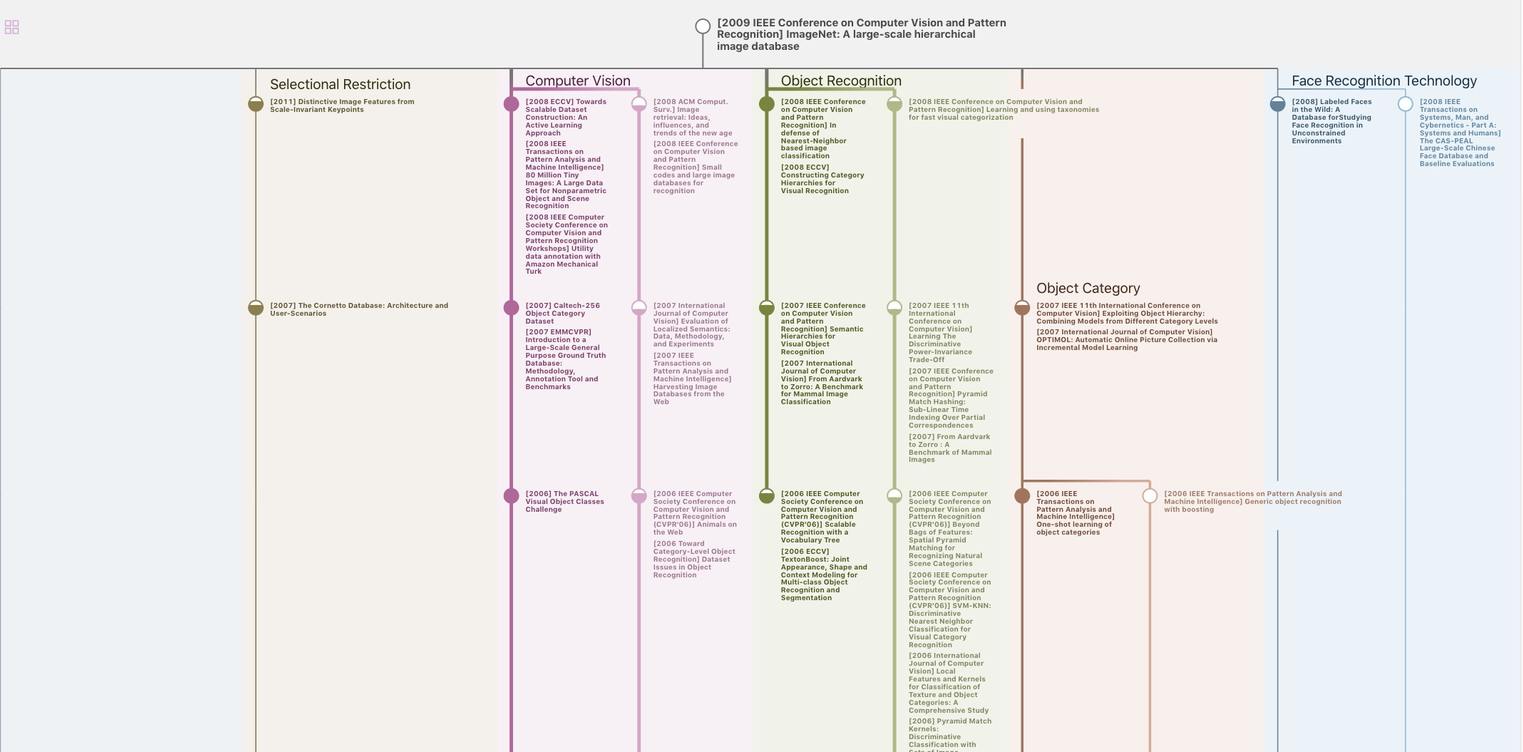
生成溯源树,研究论文发展脉络
Chat Paper
正在生成论文摘要