Dynamic Multi-Objective Optimization Framework With Interactive Evolution for Sequential Recommendation
IEEE TRANSACTIONS ON EMERGING TOPICS IN COMPUTATIONAL INTELLIGENCE(2023)
摘要
In contrast to traditional recommender systems which usually pay attention to users' general and long-term preferences, sequential recommendation (SR) can model users' dynamic intents based on their behaviour sequences and suggest the next item(s) to them. However, most of existing sequential models learn the ranking score of an item only based on its relevance property, and the personalized user demands in terms of different learning objectives, such as diversity, tail novelty or recency, which have been played essential roles in multi-objective recommendation (MOR), are often neglected in SR. In this paper, we first discuss the importance of considering multiple different objectives within a learning model for recommender system. Next, to capture users' objective-level preferences by utilizing interactive information in the sequential context, we propose a novel Dynamic Multi-objective Recommendation (DMORec) framework with interactive evolution for SR. In particular, DMORec formulates a dynamic multi-objective optimization task to simultaneously optimize more than two varying objectives in an interactive recommendation process. Moreover, to resolve this optimization task in SR, we develop an evolutionary algorithm with supervised learning approach to obtain the Pareto-optimal solutions of the formulated problem. Comprehensive experiments on four real-world datasets demonstrate the effectiveness of the proposed DMORec for dynamic multi-objective recommendation in sequential recommender systems.
更多查看译文
关键词
Optimization,Behavioral sciences,Task analysis,Tail,Training,Recommender systems,Tuning,Dynamic multi-objective optimization,multi-objective recommendation,sequential recommendation
AI 理解论文
溯源树
样例
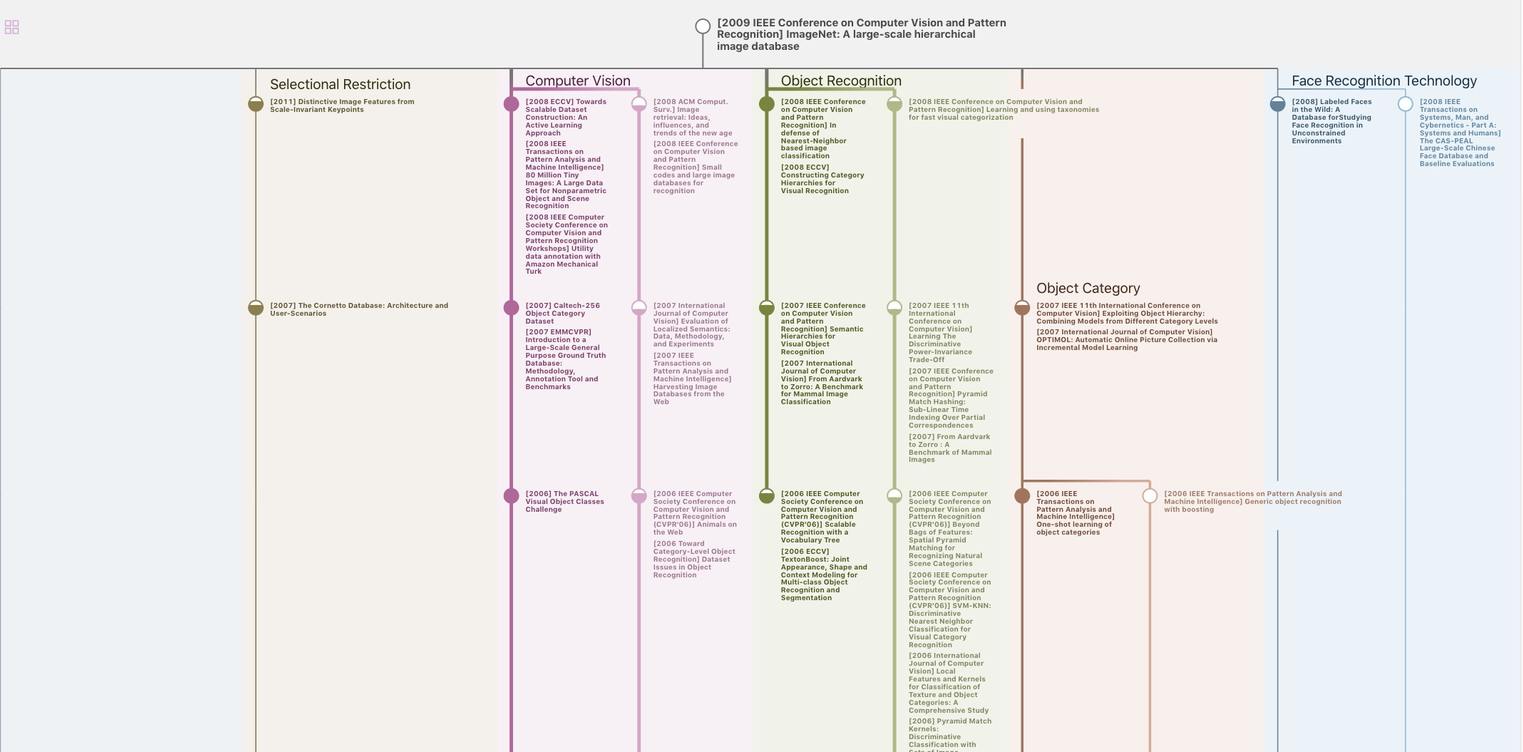
生成溯源树,研究论文发展脉络
Chat Paper
正在生成论文摘要