Sharp bound on the truncated metric dimension of trees
DISCRETE MATHEMATICS(2023)
Abstract
A k-truncated resolving set of a graph is a subset S c V of its vertex set such that the vector (dk(s, v))sES is distinct for each vertex v E V where dk(x, y) = min{d(x, y), k + 1} is the graph distance truncated at k + 1. We think of elements of a k-truncated resolving set as sensors that can measure up to distance k. The k-truncated metric dimension (Tmdk) of a graph G is the minimum cardinality of a k-truncated resolving set of G. We give a sharp lower bound on Tmdk for any tree T in terms of its number of vertices | T | and the measuring radius k. Our result is that Tmdk(T) >= |T| center dot 3/(k2 +4k + 3 +1{k = 1 (mod 3)}) + ck, disproving earlier conjectures by Frongillo et al. that suspected |T|/(lk2/4j +2k) +c'k as general lower bound, where ck, c'k are k-dependent constants. We provide a construction for trees with the largest number of vertices with a given Tmdk value. The proof that our optimal construction cannot be improved relies on edge-rewiring procedures of arbitrary (suboptimal) trees with arbitrary resolving sets, which reveal the structure of how small subsets of sensors measure and resolve certain areas in the tree that we call the attraction of those sensors. The notion of 'attraction of sensors' might be useful in other contexts beyond trees to solve related problems. We also provide an improved lower bound on Tmdk of arbitrary trees that takes into account the structural properties of the tree, in particular, the number and length of simple paths of degree-two vertices terminating in leaf vertices. This bound complements the result of the above-mentioned work of Frongillo et al., where only trees without degree-two vertices were considered, except the simple case of a single path.(c) 2023 The Authors. Published by Elsevier B.V. This is an open access article under the CC BY license (http://creativecommons .org /licenses /by /4 .0/).
MoreTranslated text
Key words
Metric dimension,k-Truncated metric dimension,Source detection
AI Read Science
Must-Reading Tree
Example
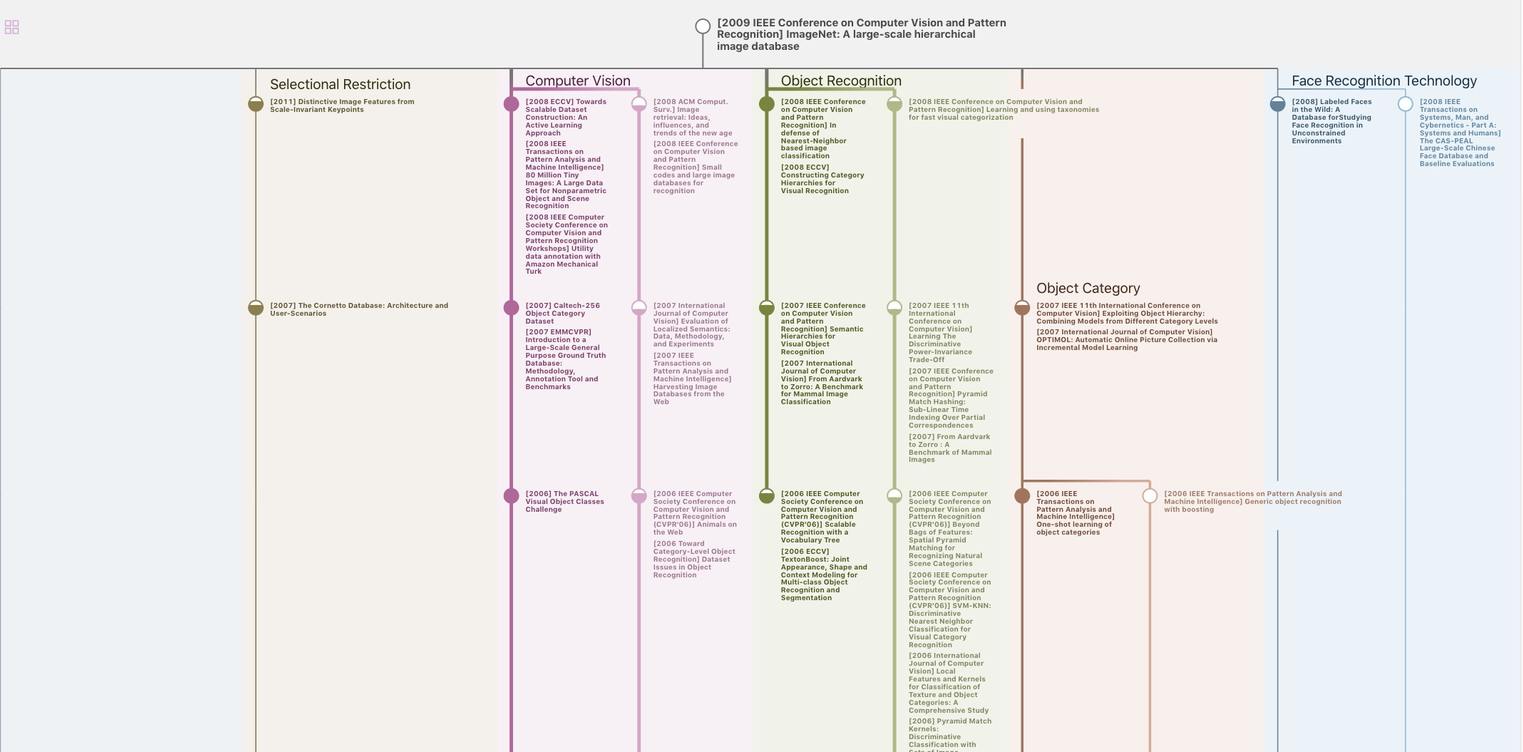
Generate MRT to find the research sequence of this paper
Chat Paper
Summary is being generated by the instructions you defined