Modeling Bilingualism as a Dynamic Phenomenon in Healthy and Neurologically Affected Speakers Across the Lifespan: A Commentary on "Computational Modeling of Bilingual Language Learning: Current Models and Future Directions"
LANGUAGE LEARNING(2023)
Abstract
In their review article, Li and Xu offered an insightful overview of the contributions and limitations of computational models of bilingual language learning and processing to our current understanding of the bilingual mind. They further proposed joining cross-disciplinary efforts toward building a computational account that links cognitive theory and neurobiological accounts of bilingualism as part of their suggested future research agenda. We agree with Li and Xu's suggestions and further propose that (a) the scope of computational models of bilingual language learning and processing should be expanded to include other perspectives: language learning context, maintenance, and decay of linguistic competence and bilingual language breakdown and that (b) existing modeling efforts already work toward addressing these areas, answering the proposed desiderata for good computational models. As reviewed by Li and Xu, developmental computational models have helped researchers understand how language representation emerges as a function of a speaker's bilingual experience. However, language learning context must be better accounted for. Specifically, bilingual language learning poses additional challenges for behavioral research when studies seek to address more naturalistic learning contexts such as second language (L2) acquisition via immersion in a foreign language context versus L2 acquisition in the classroom, and the involvement of implicit and explicit learning mechanisms constitutes an important axis of differentiation in this regard. Thus, while computational models include data-driven learning mechanisms to discover and organize linguistic representations as indicated by Li and Xu, future models should incorporate testable theory-driven implicit and explicit mechanisms for language learning (Peñaloza et al., 2022). Existing computational models of bilingual lexical access (Peñaloza et al., 2019) could incorporate such mechanisms to help test the contributions of these mechanisms to bilingual language learning. In addition, in modeling bilingual learning, both maintenance of the acquired linguistic knowledge and the reverse decay process are equally important in the lifespan timeline. For example, the extant literature makes it clear that contextual changes may reduce bilingual exposure and use that affect young and older bilinguals, yet bilingual processing decay in older adults can be further confounded with age-related language and cognitive decline (Goral et al., 2008). In supporting Li and Xu's proposal for cross-disciplinary work, we argue that computational modeling could implement and test assumptions from closely related fields including memory theory (Mickan et al., 2019) to gain understanding on how speakers’ language processing abilities in their first language (L1) and their L2 change with contextual experience and over the lifespan. Cognitive control is a domain-general ability worth incorporating in an expanded scope for computational models of bilingualism. Although it is well known that different brain regions contribute to language control in bilinguals, their unique contributions to helping bilinguals overcome cross-language interference across different learning environments and language processing contexts with high versus low cognitive control demands remain an open question. We propose that the study of bilingual language breakdown following damage to critical brain regions offers a unique window into the modeling of lexical access and cognitive control. At the broadest level, lesion studies and patient behavioral data can inform computational models of bilingual language learning and processing to achieve the cognitive and neurobiological plausibility proposed by Li and Xu. At the most specific level, the computational simulation of control, learning, and processing mechanisms in bilingual patients with brain damage could help specify causal links between specific brain regions and bilingual behavior while controlling for relevant variables difficult to manipulate in behavioral research. For instance, using the BiLex computational model (Peñaloza et al., 2019), we demonstrated that applying damage to the semantic and L1 and L2 phonetic components of individual prestroke models reproduced L1 and L2 lexical retrieval deficits in bilingual aphasia patients (Grasemann et al., 2021). In turn, applying semantic but not other lesion types could best reproduce the pattern of language decline in semantic dementia patients (Fidelman et al., 2022). These simulation findings align with theories of lexical access deficits versus semantic storage deficits put forth for these patient groups, respectively (Mirman & Britt, 2014). Notably, BiLex could offer prognostic value in predicting rates of language decline in bilingual dementia and in identifying the language to target in treatment to achieve optimal recovery across the two languages in bilingual aphasia, an approach currently being tested in a clinical trial (Peñaloza et al., 2020). Models that expand the scope outlined above go a long way toward the desiderata for good computational models proposed by Li and Xu. One example is the BiLex model (Peñaloza et al., 2019): While based on the same principles of self-organizing maps and Hebbian learning reviewed by Li and Xu, it focuses on modeling individual proficiency, impairment, and treatment-induced recovery in stroke and dementia patients. The model is valid in that its initial training parameters map to actual L2 age of acquisition and language exposure of bilingual speakers, its performance is measured via clinical tests used with patients, and its rehabilitation training parameters map to actual treatment items, intensity, and duration. It makes good contact with real language in that the semantic representations encode the semantic dimensions identified through crowdsourcing (Mechanical Turk) experiments, and its phonetic representations encode the International Phonetic Alphabet principles of the languages modeled. BiLex is interpretable in that the maps and connectivity patterns explain the behavioral patterns, and specific damage leads to specific impairments; it is also predictive because it can be used to identify the treatment options leading to the best possible recovery. In summary, we agree with Li and Xu in that an integrative computational neuroscience of bilingualism is needed, but its scope needs to be expanded to include language learning context, maintenance and decay of linguistic competence, and bilingual language breakdown to account for a larger range of phenomena in bilingual language learning and processing. Building upon work addressing the desiderata for good models proposed by Li and Xu, computational modeling can achieve cognitive and neurobiological plausibility by incorporating evidence from lesion studies and behavioral patient data to provide a vehicle for contrasting bilingualism theories and a bridge between computational science and real-world clinical needs.
MoreTranslated text
Key words
bilingualism,bilingual language learning,bilingual language processing,computational modeling
AI Read Science
Must-Reading Tree
Example
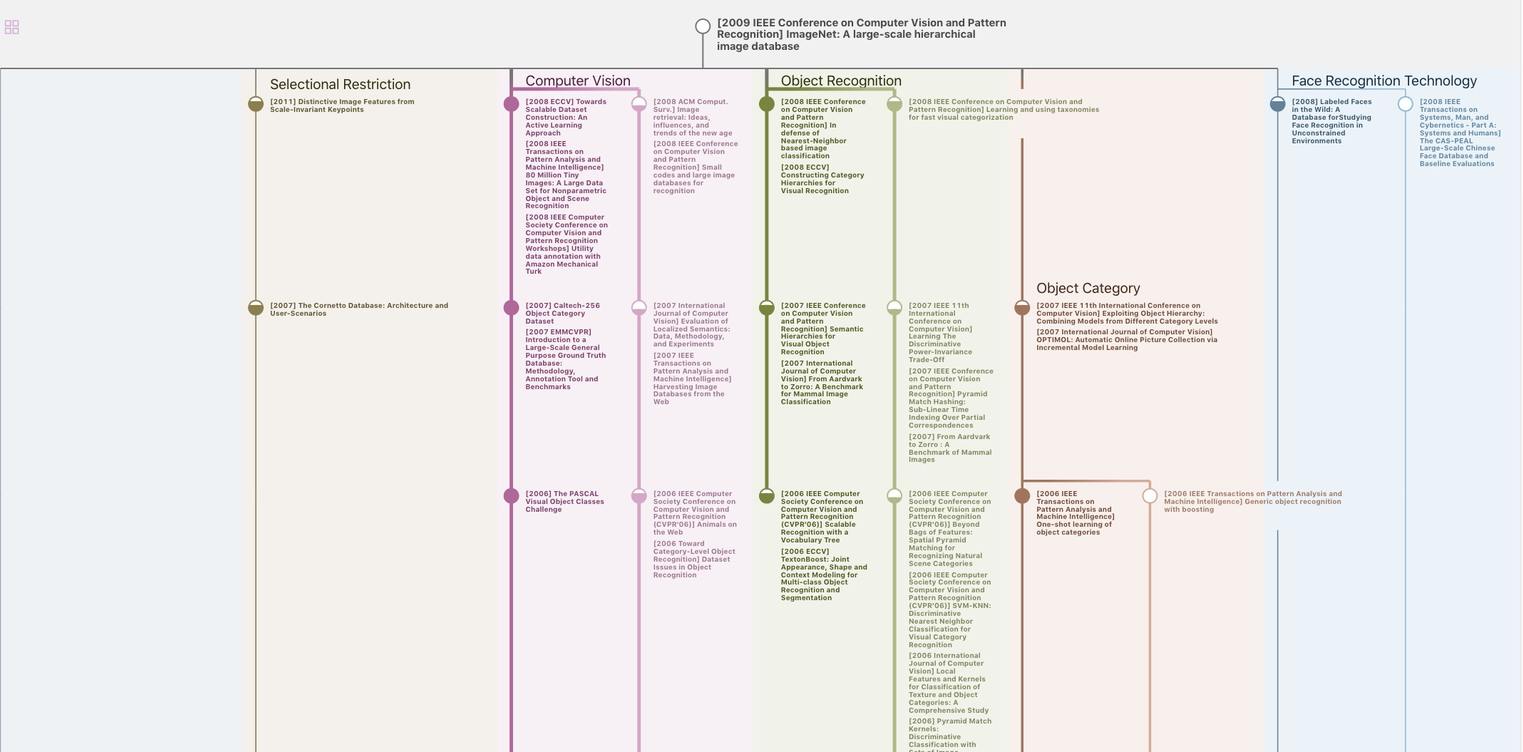
Generate MRT to find the research sequence of this paper
Chat Paper
Summary is being generated by the instructions you defined