Power-Law-Based Synthetic Minority Oversampling Technique on Imbalanced Serum Surface-Enhanced Raman Spectroscopy Data for Cancer Screening
ADVANCED INTELLIGENT SYSTEMS(2023)
摘要
Surface-enhanced Raman spectroscopy (SERS) has shown highly promising for existing cancer screening. However, previous "proof-of-concept" studies ignored the natural imbalance of cancer types in the population, leading the model to be biased toward learning more features in majority class during the learning process at the expense of ignoring minority class. Herein, a power-law-based synthetic minority oversampling technique (PL-SMOTE) method is proposed to guide the resampling of multiclass serum SERS data by analyzing the long-tailed (power-law) distribution of cancer prevalence in the population. The proposed PL-SMOTE method balances the number of minorities to resample and the number of overlaps between classes by introducing modulating factor. Modeling on resampled datasets synthesized by PL-SMOTE verifies the effectiveness of proposed PL-SMOTE method. After further fine-tuning, the parameters of the deep neural network model and PL-SMOTE method, an optimal cancer screening model with an optimal macroaveraged Recall score of 97.24% and an optimal macroaveraged F2-Score of 97.38% is obtained. A new method for multiclass imbalanced resampling is provided, which has significant improvement on model performance in terms of SERS cancer screening. The method also inspires in other multiclass imbalanced scenario, such as biological medicine, abnormal detection, and disaster prediction.
更多查看译文
关键词
data imbalance,deep neural network,multiclass,power-law,SERS,SMOTE
AI 理解论文
溯源树
样例
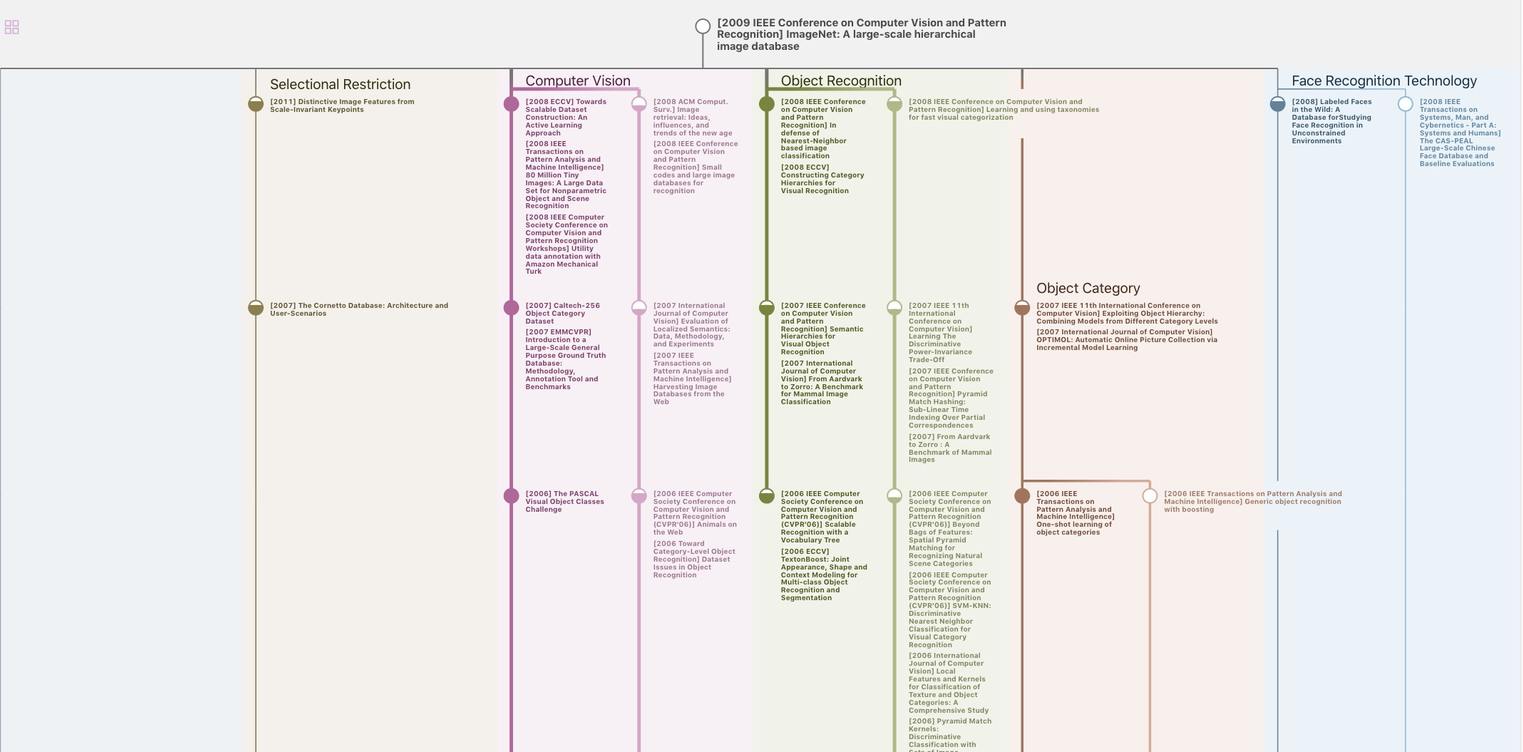
生成溯源树,研究论文发展脉络
Chat Paper
正在生成论文摘要