Process model forecasting and change exploration using time series analysis of event sequence data
DATA & KNOWLEDGE ENGINEERING(2023)
摘要
Process analytics is a collection of data-driven techniques for, among others, making predictions for individual process instances or overall process models. At the instance level, various novel techniques have been recently devised, tackling analytical tasks such as next activity, remaining time, or outcome prediction. However, there is a notable void regarding predictions at the process model level. It is the ambition of this article to fill this gap. More specifically, we develop a technique to forecast the entire process model from historical event data. A forecasted model is a will-be process model representing a probable description of the overall process for a given period in the future. Such a forecast helps, for instance, to anticipate and prepare for the consequences of upcoming process drifts and emerging bottlenecks. Our technique builds on a representation of event data as multiple time series, each capturing the evolution of a behavioural aspect of the process model, such that corresponding time series forecasting techniques can be applied. Our implementation demonstrates the feasibility of process model forecasting using real-world event data. A user study using our Process Change Exploration tool confirms the usefulness and ease of use of the produced process model forecasts.
更多查看译文
关键词
Process model forecasting,Predictive process modelling,Process mining,Time series analysis,User study
AI 理解论文
溯源树
样例
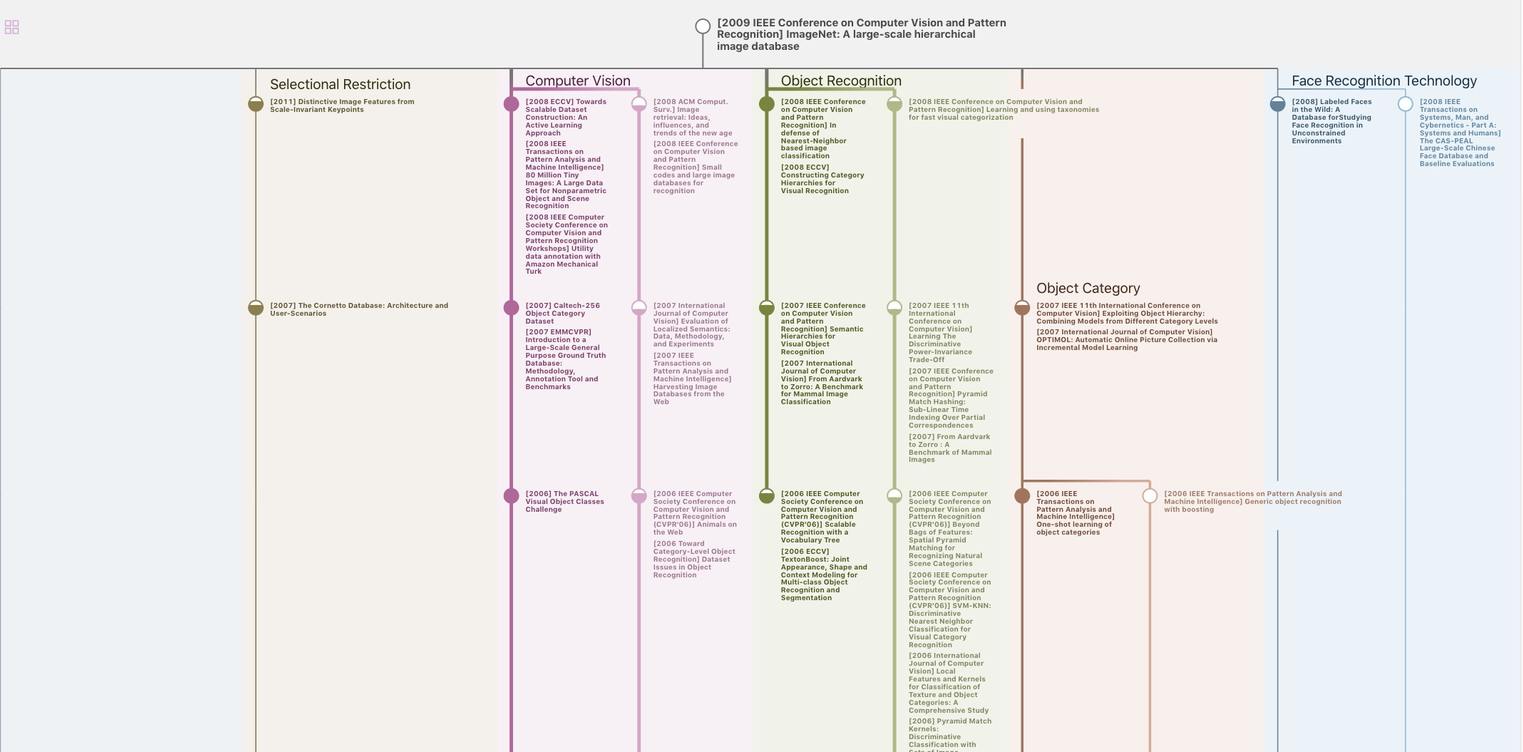
生成溯源树,研究论文发展脉络
Chat Paper
正在生成论文摘要