An accelerated proximal algorithm for regularized nonconvex and nonsmooth bi-level optimization
MACHINE LEARNING(2023)
摘要
Many important machine learning applications involve regularized nonconvex bi-level optimization. However, the existing gradient-based bi-level optimization algorithms cannot handle nonconvex or nonsmooth regularizers, and they suffer from a high computation complexity in nonconvex bi-level optimization. In this work, we study a proximal gradient-type algorithm that adopts the approximate implicit differentiation (AID) scheme for nonconvex bi-level optimization with possibly nonconvex and nonsmooth regularizers. In particular, the algorithm applies the Nesterov’s momentum to accelerate the computation of the implicit gradient involved in AID. We provide a comprehensive analysis of the global convergence properties of this algorithm through identifying its intrinsic potential function. In particular, we formally establish the convergence of the model parameters to a critical point of the bi-level problem, and obtain an improved computation complexity 𝒪(κ ^3.5ϵ ^-2) over the state-of-the-art result. Moreover, we analyze the asymptotic convergence rates of this algorithm under a class of local nonconvex geometries characterized by a Łojasiewicz-type gradient inequality. Experiment on hyper-parameter optimization demonstrates the effectiveness of our algorithm.
更多查看译文
关键词
Bilevel optimization,Nesterov’s momentum,Nonconvex regularization,Proximal algorithm
AI 理解论文
溯源树
样例
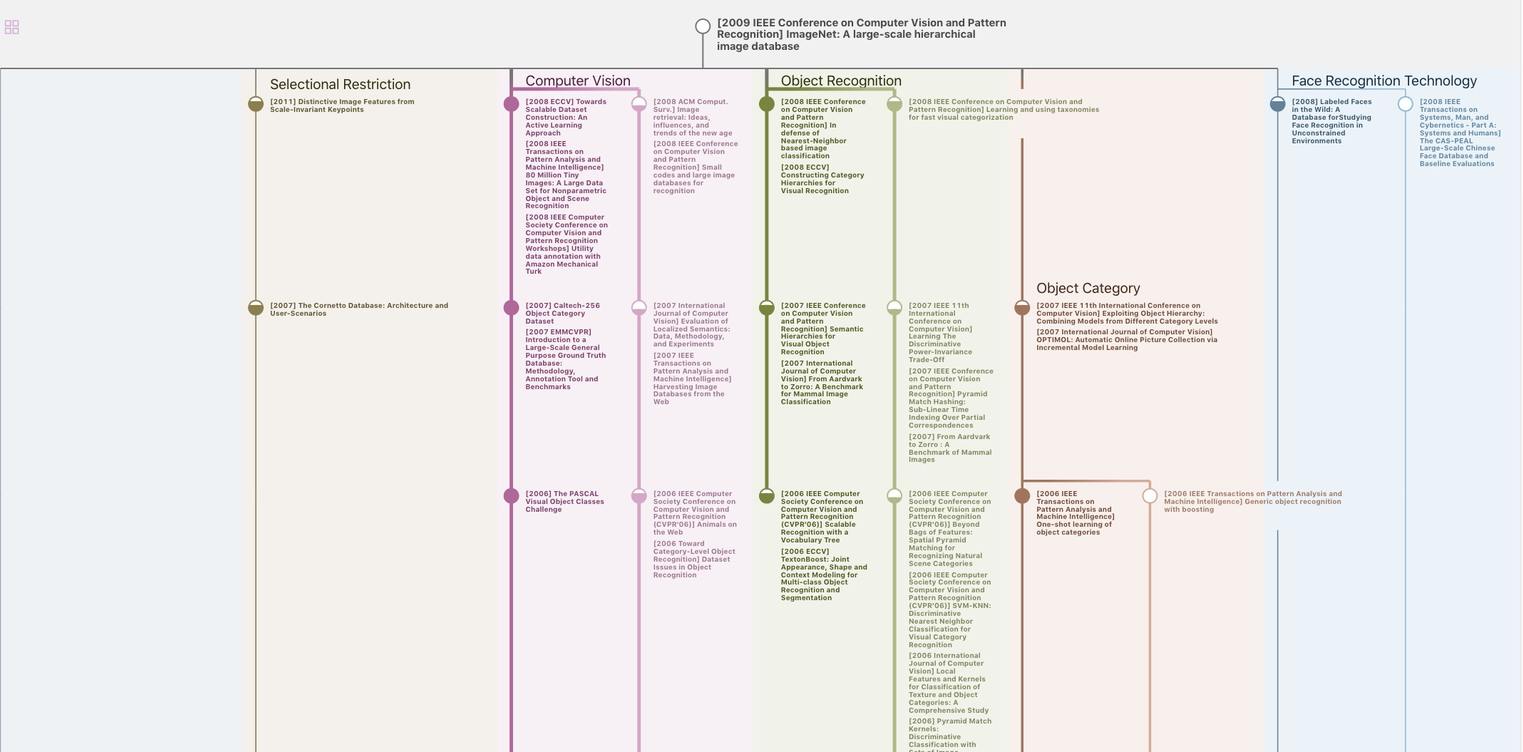
生成溯源树,研究论文发展脉络
Chat Paper
正在生成论文摘要