Machine Learning Identifies Plasma Proteomic Signatures of Descending Thoracic Aortic Disease
bioRxiv : the preprint server for biology(2023)
摘要
Descending thoracic aortic aneurysms may go undetected until severe and catastrophic complications such as rupture or dissection occur. Few clinical indicators exist to screen for aneurysms or predict risk of dissection or rupture. This study generated a plasma proteomic dataset from 150 patients with descending thoracic aortic disease and 52 controls to identify proteomic signatures capable of differentiating descending thoracic aortic disease from controls, as well as aneurysm from descending aortic dissections. Of the 1,468 peptides and 195 proteins quantified, 853 peptides and 99 proteins were significantly different between disease and controls. Using machine learning to classify disease from control, the highest precision-recall area under the curve (PR AUC) was achieved using significantly different proteins (PR AUC 0.99), followed by significant peptides (PR AUC 0.96). Despite no statistically significant protein signatures between aneurysm and dissection, use of all proteins was able to modestly classify between the disease states (PR AUC 0.77). To address correlation between proteins, a disease versus control classifier was optimized using only seven unique protein clusters, which achieved comparable performance to models trained on all/significant proteins (PR AUC 0.90). Model interpretation with permutation importance revealed that proteins differentiating disease and control function in coagulation, protein-lipid complex remodeling, and acute inflammatory response.
### Competing Interest Statement
The authors have declared no competing interest.
更多查看译文
AI 理解论文
溯源树
样例
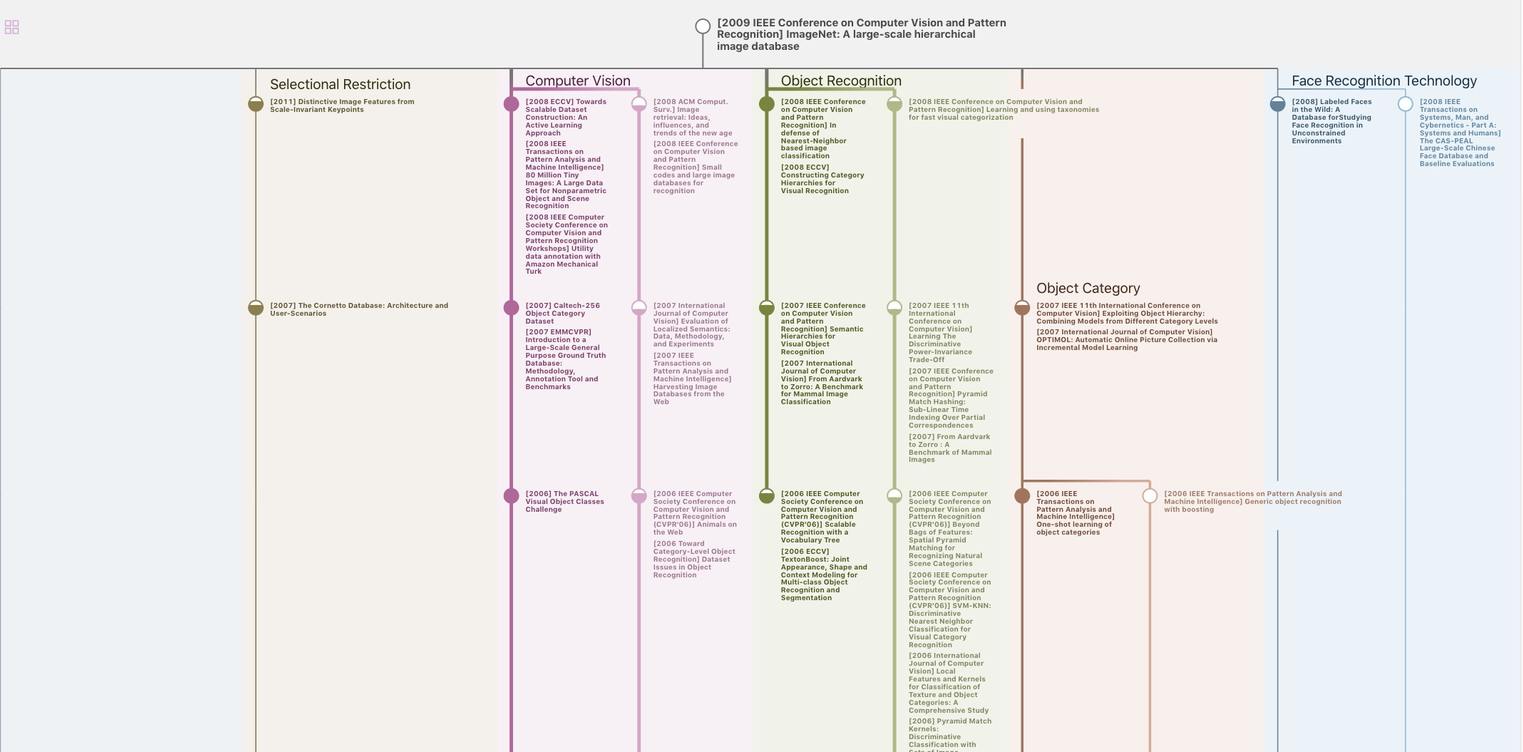
生成溯源树,研究论文发展脉络
Chat Paper
正在生成论文摘要