Rapid Synthesis of Cryo-ET Data for Training Deep Learning Models
bioRxiv : the preprint server for biology(2023)
Abstract
Deep learning excels at cryo-tomographic image restoration and segmentation tasks but is hindered by a lack of training data. Here we introduce cryo-TomoSim (CTS), a MATLAB-based software package that builds coarse-grained models of macromolecular complexes embedded in vitreous ice and then simulates transmitted electron tilt series for tomographic reconstruction. We then demonstrate the effectiveness of these simulated datasets in training different deep learning models for use on real cryotomographic reconstructions. Computer-generated ground truth datasets provide the means for training models with voxel-level precision, allowing for unprecedented denoising and precise molecular segmentation of datasets. By modeling phenomena such as a three-dimensional contrast transfer function, probabilistic detection events, and radiation-induced damage, the simulated cryo-electron tomograms can cover a large range of imaging content and conditions to optimize training sets. When paired with small amounts of training data from real tomograms, networks become incredibly accurate at segmenting in situ macromolecular assemblies across a wide range of biological contexts.
Summary By pairing rapidly synthesized Cryo-ET data with computed ground truths, deep learning models can be trained to accurately restore and segment real tomograms of biological structures both in vitro and in situ .
### Competing Interest Statement
The authors have declared no competing interest.
MoreTranslated text
Key words
training deep learning models,deep learning,rapid synthesis
AI Read Science
Must-Reading Tree
Example
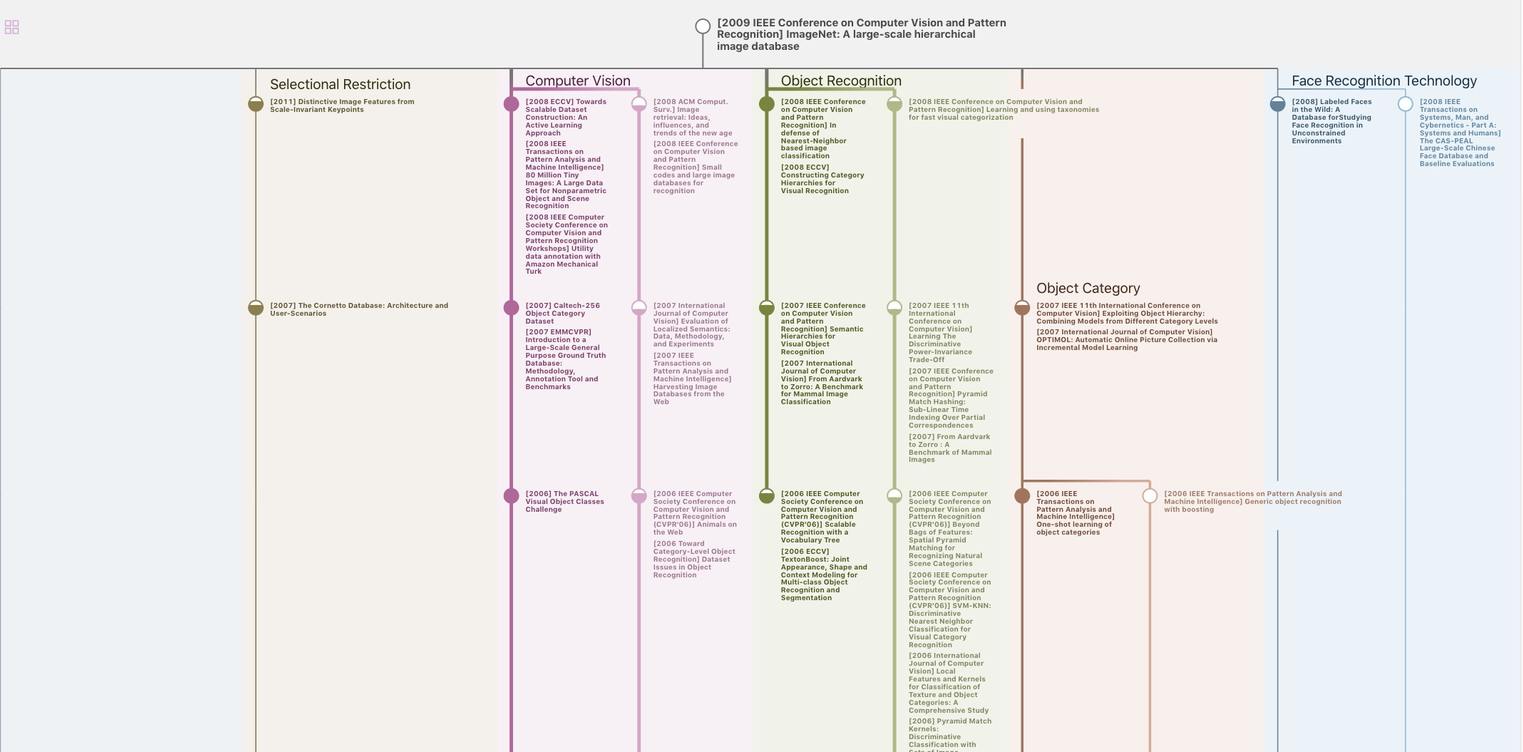
Generate MRT to find the research sequence of this paper
Chat Paper
Summary is being generated by the instructions you defined