A foreground-immune CMB-cluster lensing estimator
JOURNAL OF COSMOLOGY AND ASTROPARTICLE PHYSICS(2023)
摘要
Galaxy clusters induce a distinct dipole pattern in the cosmic microwave back-ground (CMB) through the effect of gravitational lensing. Extracting this lensing signal will enable us to constrain cluster masses, even for high redshift clusters (z >= 1) that are expected to be detected by future CMB surveys. However, cluster-correlated foreground signals, like the kinematic and thermal Sunyaev-Zel'dovich (kSZ and tSZ) signals, present a challenge when extracting the lensing signal from CMB temperature data. While CMB polarization -based lensing reconstruction is one way to mitigate these foreground biases, the sensitivity from CMB temperature-based reconstruction is expected to be similar to or higher than po-larization for future surveys. In this work, we extend the cluster lensing estimator developed in [1] to CMB temperature and test its robustness against systematic biases from foreground signals. We find that the kSZ signal only acts as an additional source of variance and provide a simple stacking-based approach to mitigate the bias from the tSZ signal. Additionally, we study the bias induced due to uncertainties in the cluster positions and show that they can be easily mitigated. The estimated signal-to-noise ratio (SNR) of this estimator is comparable to other standard lensing estimators such as the maximum likelihood (MLE) and quadratic (QE) estimators. We predict the cluster mass uncertainties from CMB temperature data for current and future cluster samples to be: 6.6% for SPT-3G with 7,000 clusters, 4.1% for SO and 3.9% for SO + FYST with 25,000 clusters, and 1.8% for CMB-S4 with 100,000 clusters.
更多查看译文
关键词
CMBR experiments,galaxy clusters,weak gravitational lensing
AI 理解论文
溯源树
样例
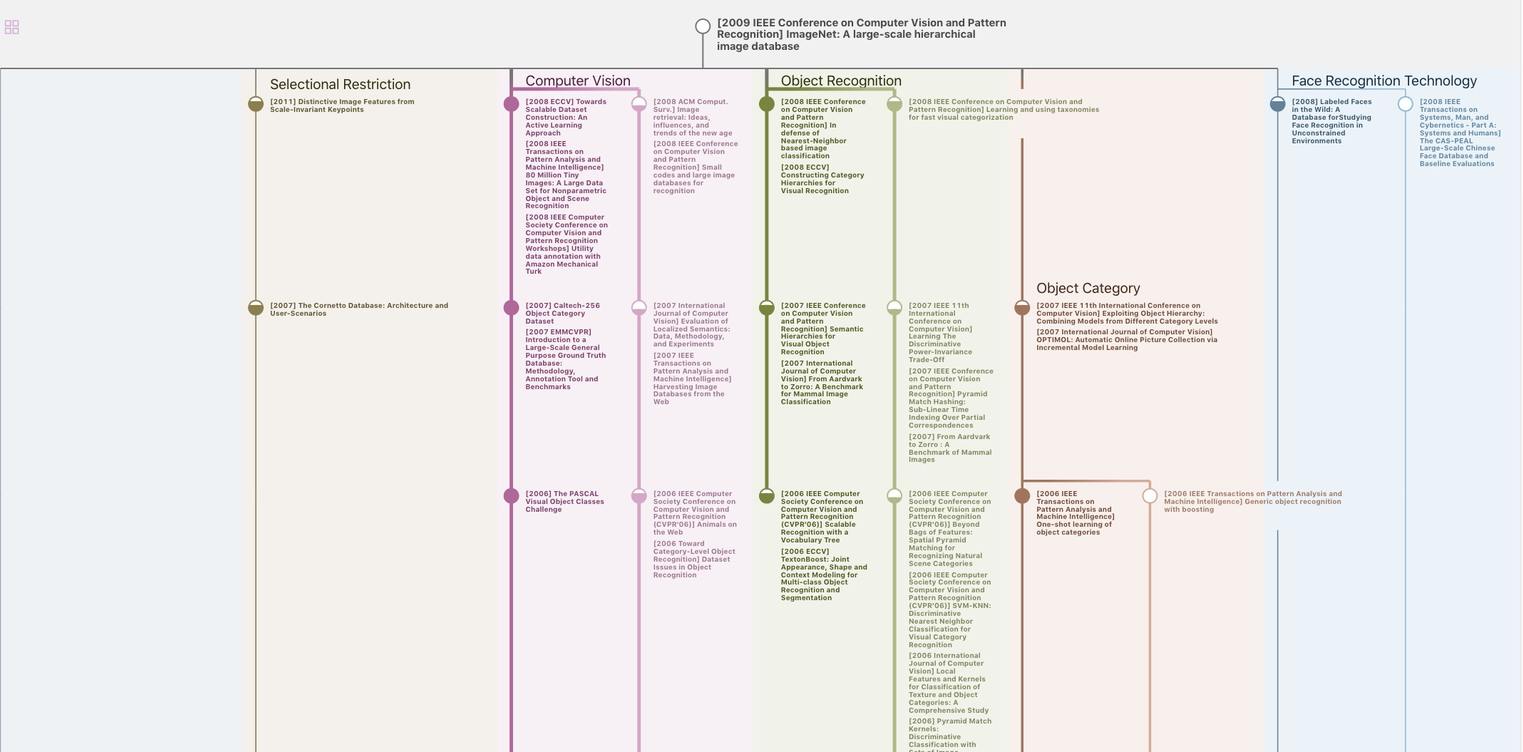
生成溯源树,研究论文发展脉络
Chat Paper
正在生成论文摘要