Self-Supervised Instance Segmentation by Grasping
2023 IEEE/RSJ INTERNATIONAL CONFERENCE ON INTELLIGENT ROBOTS AND SYSTEMS, IROS(2023)
Abstract
Instance segmentation is a fundamental skill for many robotic applications. We propose a self-supervised method that uses grasp interactions to collect segmentation supervision for an instance segmentation model. When a robot grasps an item, the mask of that grasped item can be inferred from the images of the scene before and after the grasp. Leveraging this insight, we learn a grasp segmentation model from a small dataset of labelled images to segment the grasped object from before and after grasp images. Such a model can segment grasped objects from thousands of grasp interactions without costly human annotation. Using the segmented grasped objects, we can "cut" objects from their original scenes and "paste" them into new scenes to generate instance supervision. We show that our grasp segmentation model provides a 5x error reduction when segmenting grasped objects compared with traditional image subtraction approaches. Combined with our "cut-and-paste" generation method, instance segmentation models trained with our method achieve better performance than a model trained with 10x the amount of labeled data. On a real robotic grasping system, our instance segmentation model reduces the rate of grasp errors by over 3x compared to an image subtraction baseline.
MoreTranslated text
Key words
segmentation,grasping,instance,self-supervised
AI Read Science
Must-Reading Tree
Example
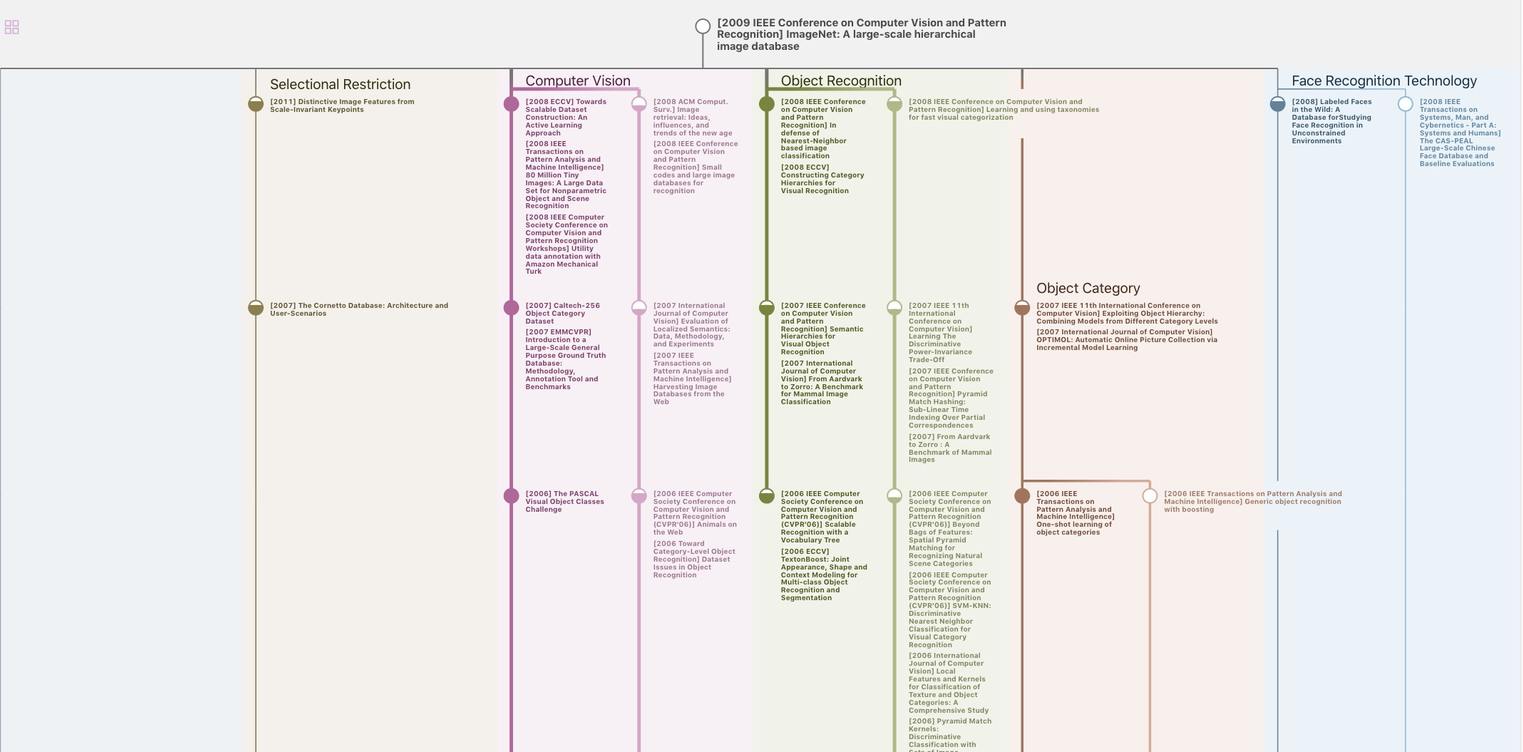
Generate MRT to find the research sequence of this paper
Chat Paper
Summary is being generated by the instructions you defined