Structural Hawkes processes for learning causal structure from discrete-time event sequences
IJCAI 2023(2023)
摘要
Learning causal structure among event types from discrete-time event sequences is a particularly important but challenging task. Existing methods, such as the multivariate Hawkes processes based methods, mostly boil down to learning the so-called Granger causality which assumes that the cause event happens strictly prior to its effect event. Such an assumption is often untenable beyond applications, especially when dealing with discrete-time event sequences in low-resolution; and typical discrete Hawkes processes mainly suffer from identifiability issues raised by the instantaneous effect, i.e., the causal relationship that occurred simultaneously due to the low-resolution data will not be captured by Granger causality. In this work, we propose Structure Hawkes Processes (SHPs) that leverage the instantaneous effect for learning the causal structure among events type in discrete-time event sequence. The proposed method is featured with the minorization-maximization of the likelihood function and a sparse optimization scheme. Theoretical results show that the instantaneous effect is a blessing rather than a curse, and the causal structure is identifiable under the existence of the instantaneous effect. Experiments on synthetic and real-world data verify the effectiveness of the proposed method.
更多查看译文
关键词
structural hawkes processes,learning causal structure,discrete-time
AI 理解论文
溯源树
样例
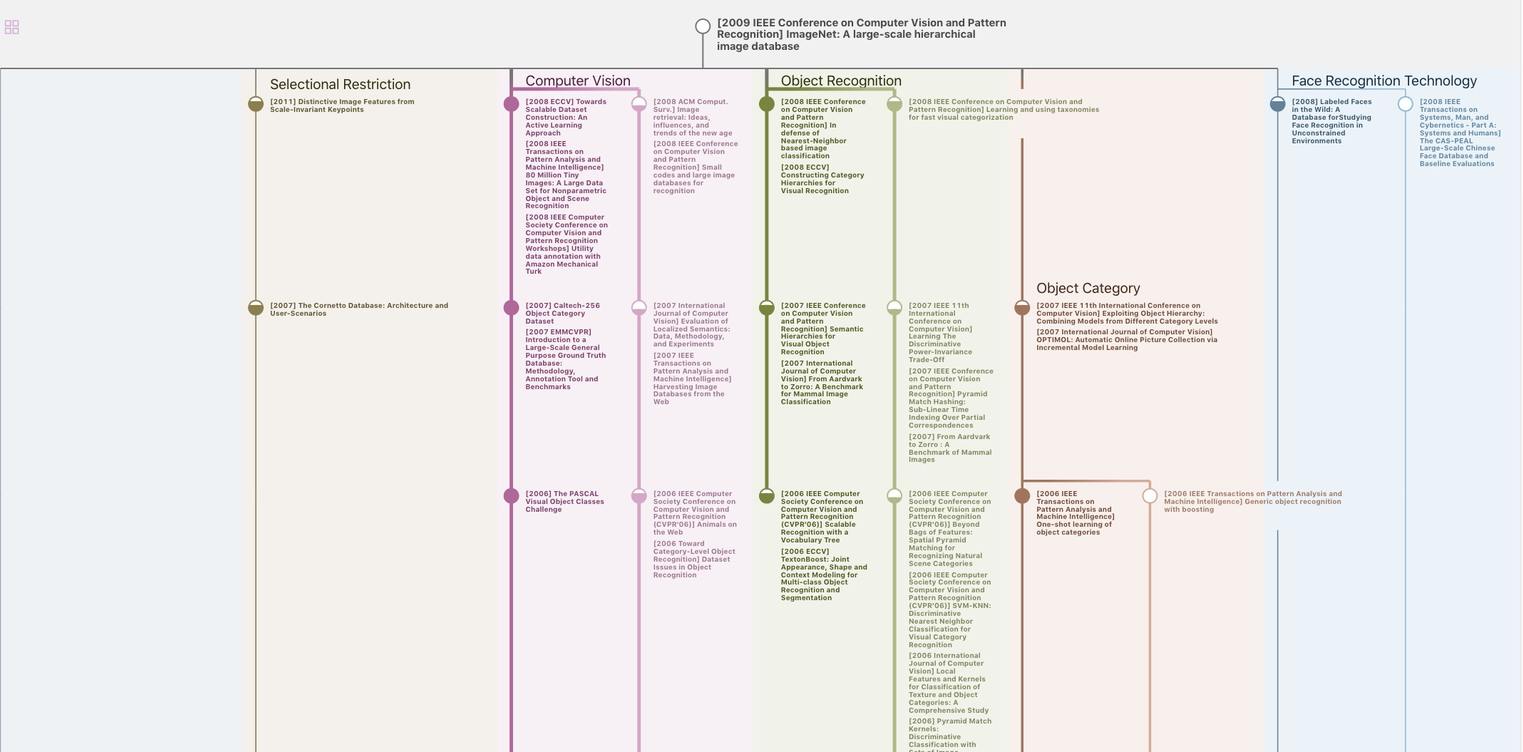
生成溯源树,研究论文发展脉络
Chat Paper
正在生成论文摘要