iEdit: Localised Text-guided Image Editing with Weak Supervision
CoRR(2023)
摘要
Diffusion models (DMs) can generate realistic images with text guidance using large-scale datasets. However, they demonstrate limited controllability in the output space of the generated images. We propose a novel learning method for text-guided image editing, namely \texttt{iEdit}, that generates images conditioned on a source image and a textual edit prompt. As a fully-annotated dataset with target images does not exist, previous approaches perform subject-specific fine-tuning at test time or adopt contrastive learning without a target image, leading to issues on preserving the fidelity of the source image. We propose to automatically construct a dataset derived from LAION-5B, containing pseudo-target images with their descriptive edit prompts given input image-caption pairs. This dataset gives us the flexibility of introducing a weakly-supervised loss function to generate the pseudo-target image from the latent noise of the source image conditioned on the edit prompt. To encourage localised editing and preserve or modify spatial structures in the image, we propose a loss function that uses segmentation masks to guide the editing during training and optionally at inference. Our model is trained on the constructed dataset with 200K samples and constrained GPU resources. It shows favourable results against its counterparts in terms of image fidelity, CLIP alignment score and qualitatively for editing both generated and real images.
更多查看译文
关键词
image editing,supervision,text-guided
AI 理解论文
溯源树
样例
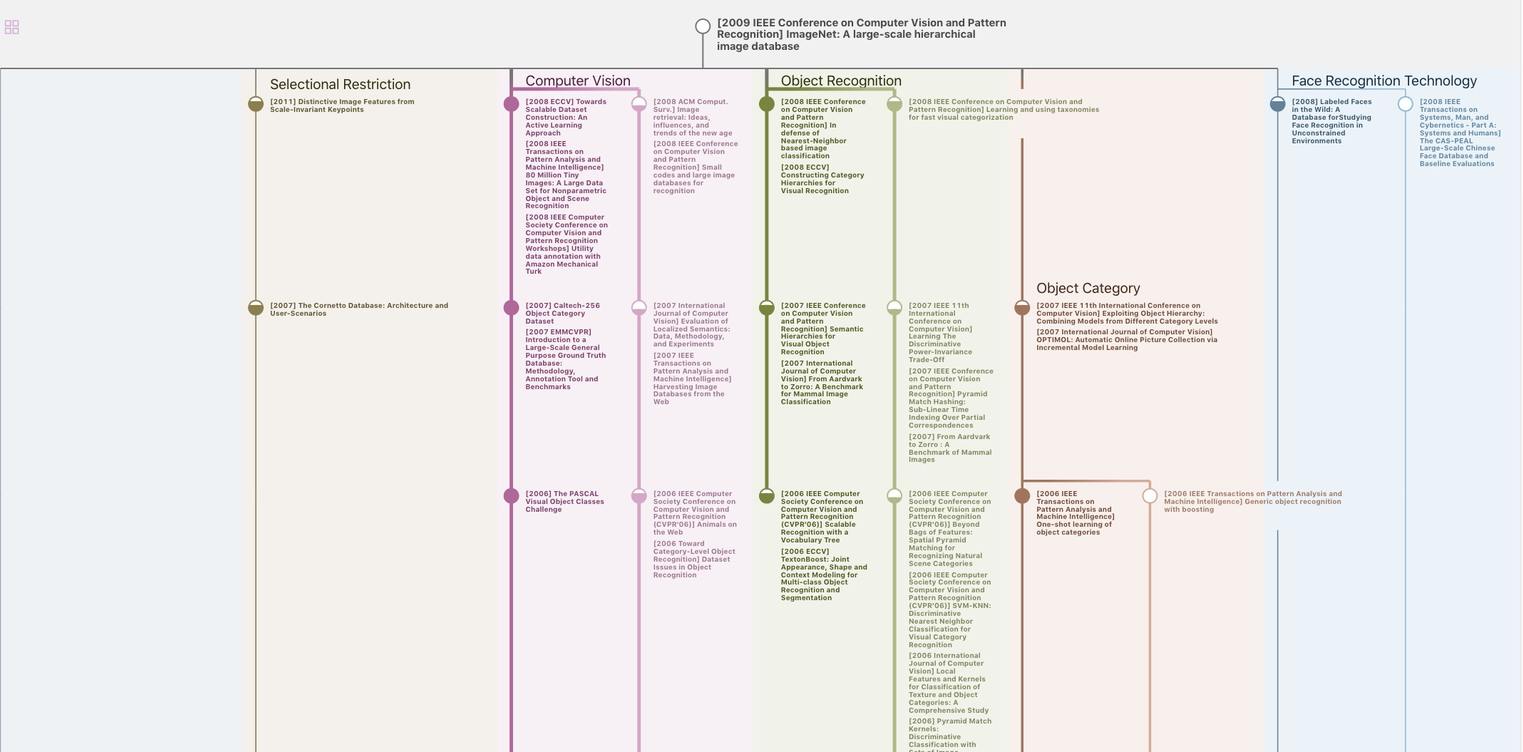
生成溯源树,研究论文发展脉络
Chat Paper
正在生成论文摘要