Can Point Cloud Networks Learn Statistical Shape Models of Anatomies?
MEDICAL IMAGE COMPUTING AND COMPUTER ASSISTED INTERVENTION, MICCAI 2023, PT I(2023)
摘要
Statistical Shape Modeling (SSM) is a valuable tool for investigating and quantifying anatomical variations within populations of anatomies. However, traditional correspondence-based SSM generation methods have a prohibitive inference process and require complete geometric proxies (e.g., high-resolution binary volumes or surface meshes) as input shapes to construct the SSM. Unordered 3D point cloud representations of shapes are more easily acquired from various medical imaging practices (e.g., thresholded images and surface scanning). Point cloud deep networks have recently achieved remarkable success in learning permutation-invariant features for different point cloud tasks (e.g., completion, semantic segmentation, classification). However, their application to learning SSM from point clouds is to-date unexplored. In this work, we demonstrate that existing point cloud encoder-decoder-based completion networks can provide an untapped potential for SSM, capturing population-level statistical representations of shapes while reducing the inference burden and relaxing the input requirement. We discuss the limitations of these techniques to the SSM application and suggest future improvements. Our work paves the way for further exploration of point cloud deep learning for SSM, a promising avenue for advancing shape analysis literature and broadening SSM to diverse use cases.
更多查看译文
关键词
Statistical Shape Modeling,Point Cloud Deep Networks,Morphometrics
AI 理解论文
溯源树
样例
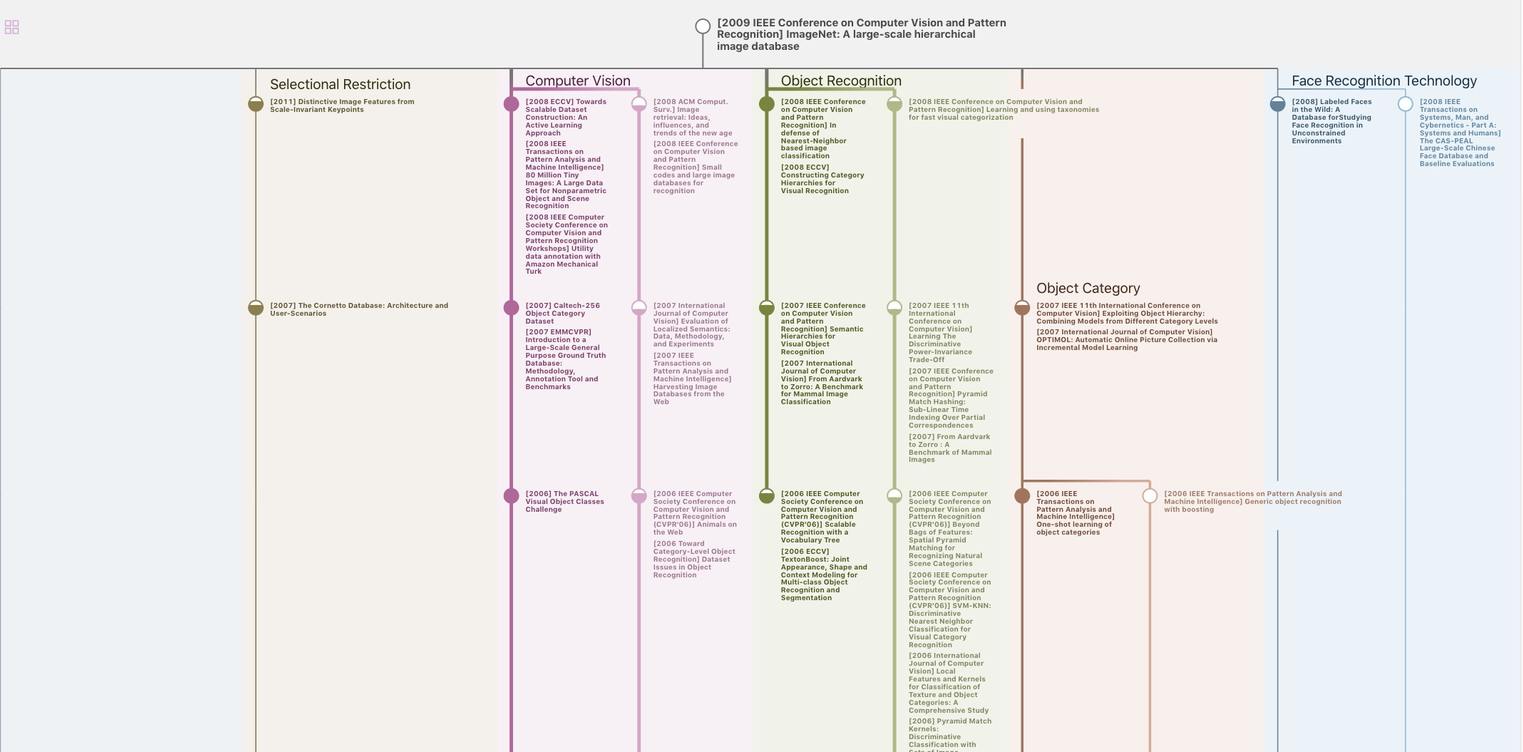
生成溯源树,研究论文发展脉络
Chat Paper
正在生成论文摘要