Direct Poisson neural networks: learning non-symplectic mechanical systems
JOURNAL OF PHYSICS A-MATHEMATICAL AND THEORETICAL(2023)
摘要
In this paper, we present neural networks learning mechanical systems that are both symplectic (for instance particle mechanics) and non-symplectic (for instance rotating rigid body). Mechanical systems have Hamiltonian evolution, which consists of two building blocks: a Poisson bracket and an energy functional. We feed a set of snapshots of a Hamiltonian system to our neural network models which then find both the two building blocks. In particular, the models distinguish between symplectic systems (with non-degenerate Poisson brackets) and non-symplectic systems (degenerate brackets). In contrast with earlier works, our approach does not assume any further a priori information about the dynamics except its Hamiltonianity, and it returns Poisson brackets that satisfy Jacobi identity. Finally, the models indicate whether a system of equations is Hamiltonian or not.
更多查看译文
关键词
machine learning,Hamiltonian mechanics,non-symplectic,neural networks,Poisson
AI 理解论文
溯源树
样例
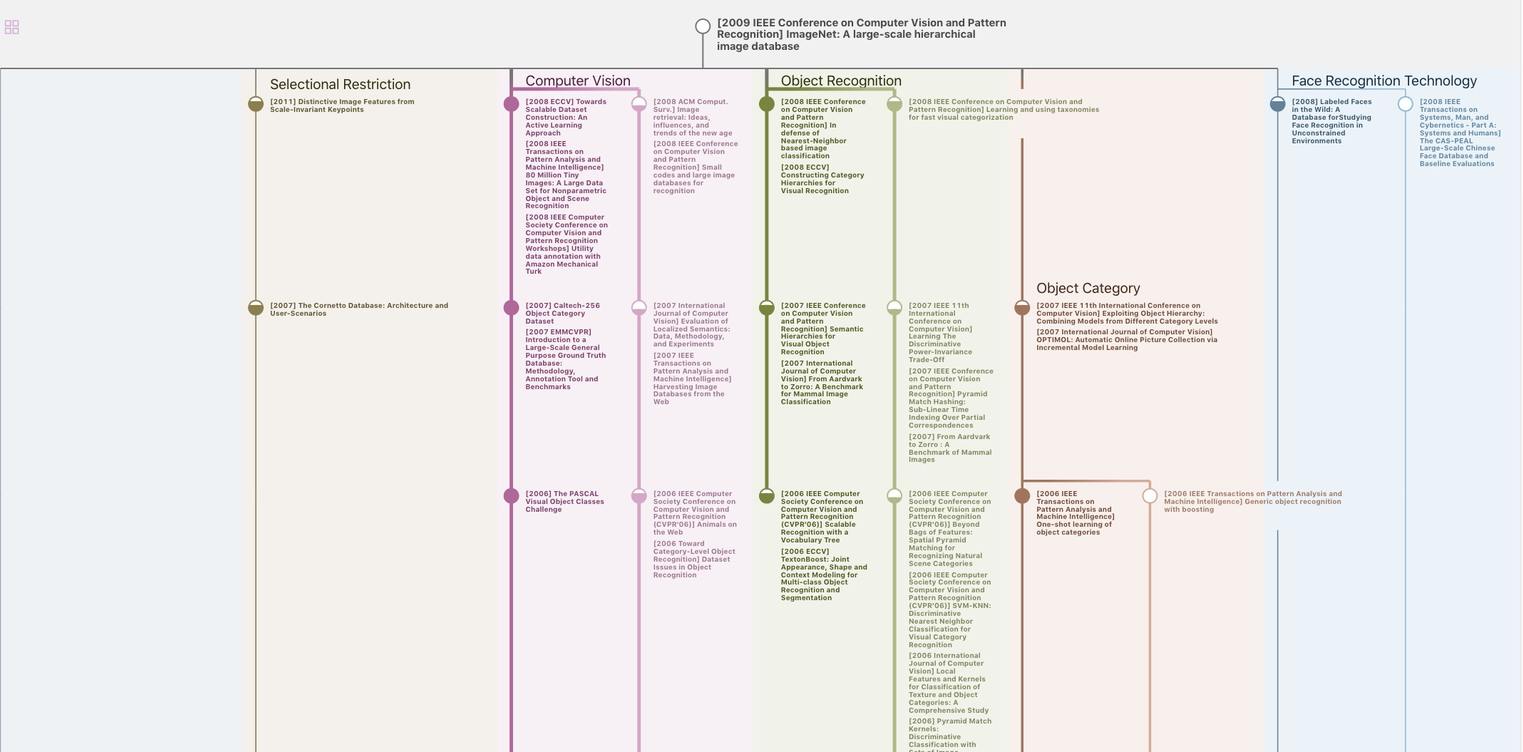
生成溯源树,研究论文发展脉络
Chat Paper
正在生成论文摘要