CaseEncoder: A Knowledge-enhanced Pre-trained Model for Legal Case Encoding.
CoRR(2023)
摘要
Legal case retrieval is a critical process for modern legal information systems. While recent studies have utilized pre-trained language models (PLMs) based on the general domain self-supervised pre-training paradigm to build models for legal case retrieval, there are limitations in using general domain PLMs as backbones. Specifically, these models may not fully capture the underlying legal features in legal case documents. To address this issue, we propose CaseEncoder, a legal document encoder that leverages fine-grained legal knowledge in both the data sampling and pre-training phases. In the data sampling phase, we enhance the quality of the training data by utilizing fine-grained law article information to guide the selection of positive and negative examples. In the pre-training phase, we design legal-specific pre-training tasks that align with the judging criteria of relevant legal cases. Based on these tasks, we introduce an innovative loss function called Biased Circle Loss to enhance the model's ability to recognize case relevance in fine grains. Experimental results on multiple benchmarks demonstrate that CaseEncoder significantly outperforms both existing general pre-training models and legal-specific pre-training models in zero-shot legal case retrieval.
更多查看译文
关键词
legal caseencoder,knowledge-enhanced,pre-trained
AI 理解论文
溯源树
样例
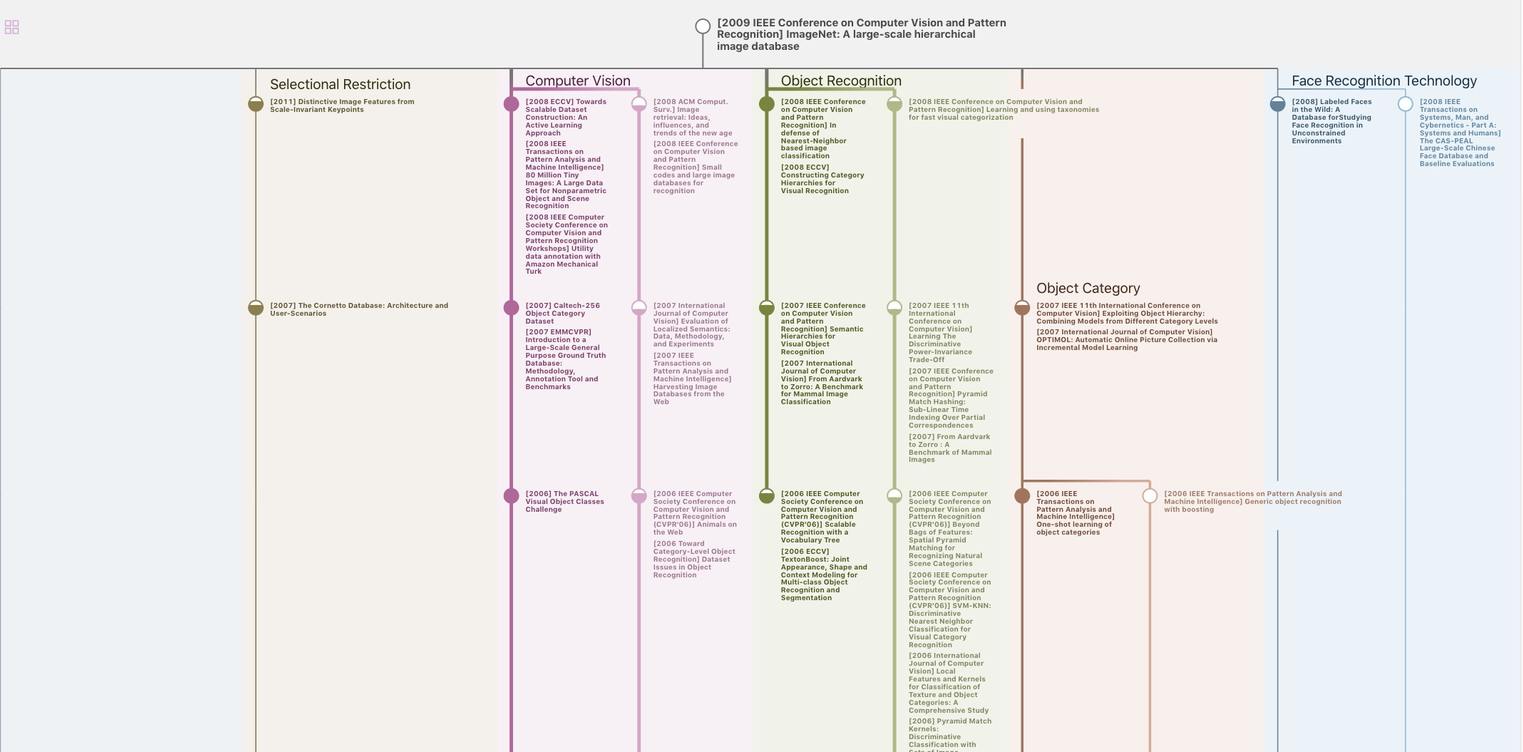
生成溯源树,研究论文发展脉络
Chat Paper
正在生成论文摘要