DietCNN: Multiplication-free Inference for Quantized CNNs
2023 INTERNATIONAL JOINT CONFERENCE ON NEURAL NETWORKS, IJCNN(2023)
摘要
The rising demand for networked embedded systems with machine intelligence has been a catalyst for sustained attempts by the research community to implement Convolutional Neural Networks (CNN) based inferencing on embedded resource-limited devices. Redesigning a CNN by removing costly multiplication operations has already shown promising results in terms of reducing inference energy usage. This paper proposes a new method for replacing multiplications in a CNN by table lookups. Unlike existing methods that completely modify the CNN operations, the proposed methodology preserves the semantics of the major CNN operations. Conforming to the existing mechanism of the CNN layer operations ensures that the reliability of a standard CNN is preserved. It is shown that the proposed multiplication-free CNN, based on a single activation codebook, can achieve 4.7x, 5.6x, and 3.5x reduction in energy per inference in an FPGA implementation of MNIST-LeNet-5, CIFAR10-VGG11, and Tiny ImageNet-ResNet-18 respectively. Our results show that the DietCNN approach significantly improves the resource consumption and latency of deep inference for smaller models, often used in embedded systems. Our code is available at: https://github.com/swadeykgp/DietCNN
更多查看译文
关键词
dietcnn,multiplication-free
AI 理解论文
溯源树
样例
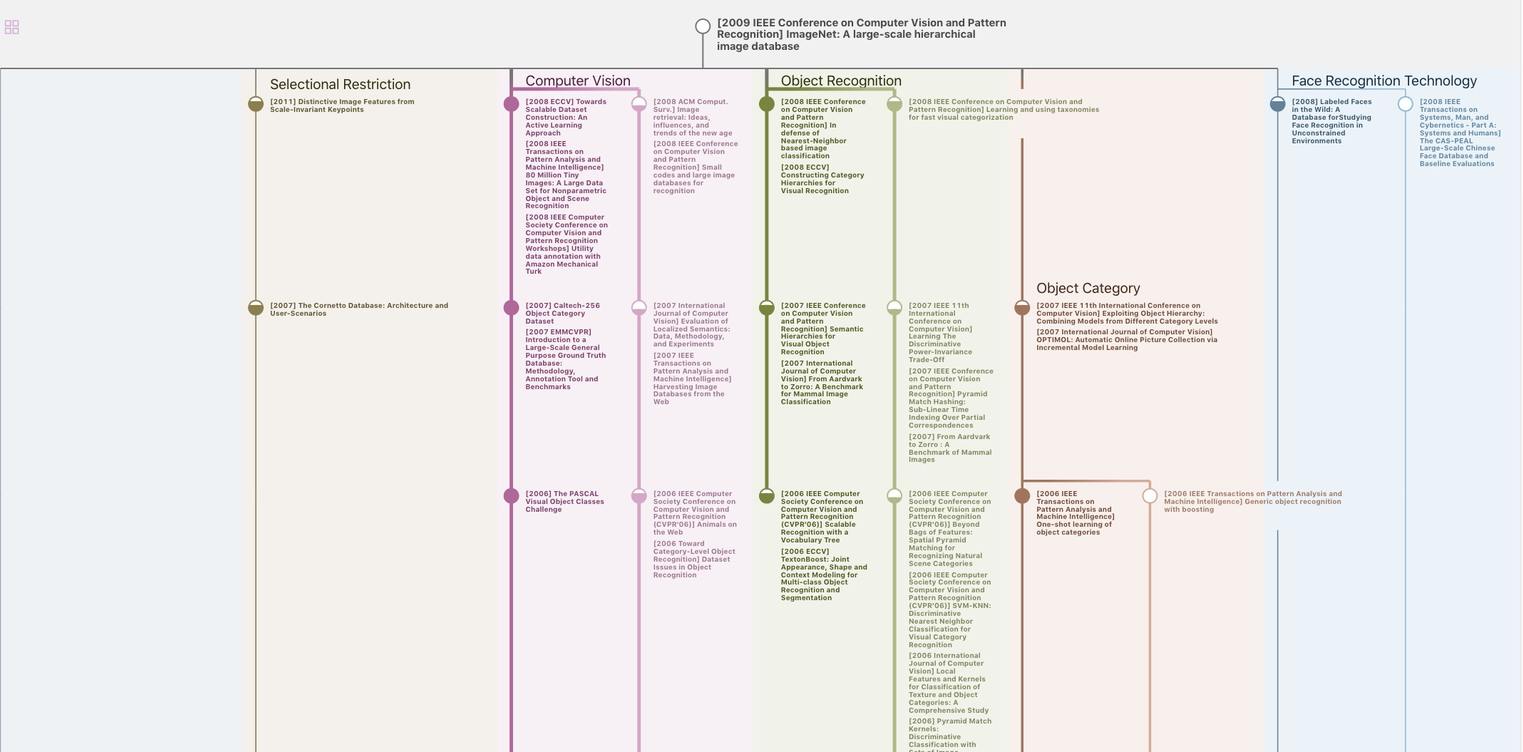
生成溯源树,研究论文发展脉络
Chat Paper
正在生成论文摘要