Adapt and Align to Improve Zero-Shot Sketch-Based Image Retrieval
arXivorg(2023)
摘要
Zero-shot sketch-based image retrieval (ZS-SBIR) is challenging due to the cross-domain nature of sketches and photos, as well as the semantic gap between seen and unseen image distributions. Previous methods fine-tune pre-trained models with various side information and learning strategies to learn a compact feature space that is shared between the sketch and photo domains and bridges seen and unseen classes. However, these efforts are inadequate in adapting domains and transferring knowledge from seen to unseen classes. In this paper, we present an effective “Adapt and Align” approach to address the key challenges. Specifically, we insert simple and lightweight domain adapters to learn new abstract concepts of the sketch domain and improve cross-domain representation capabilities. Inspired by recent advances in image-text foundation models (e.g., CLIP) on zero-shot scenarios, we explicitly align the learned image embedding with a more semantic text embedding to achieve the desired knowledge transfer from seen to unseen classes. Extensive experiments on three benchmark datasets and two popular backbones demonstrate the superiority of our method in terms of retrieval accuracy and flexibility.
更多查看译文
关键词
Domain Adaptation,Feature Matching,Image Retrieval,Semi-Supervised Learning,Visual Question Answering
AI 理解论文
溯源树
样例
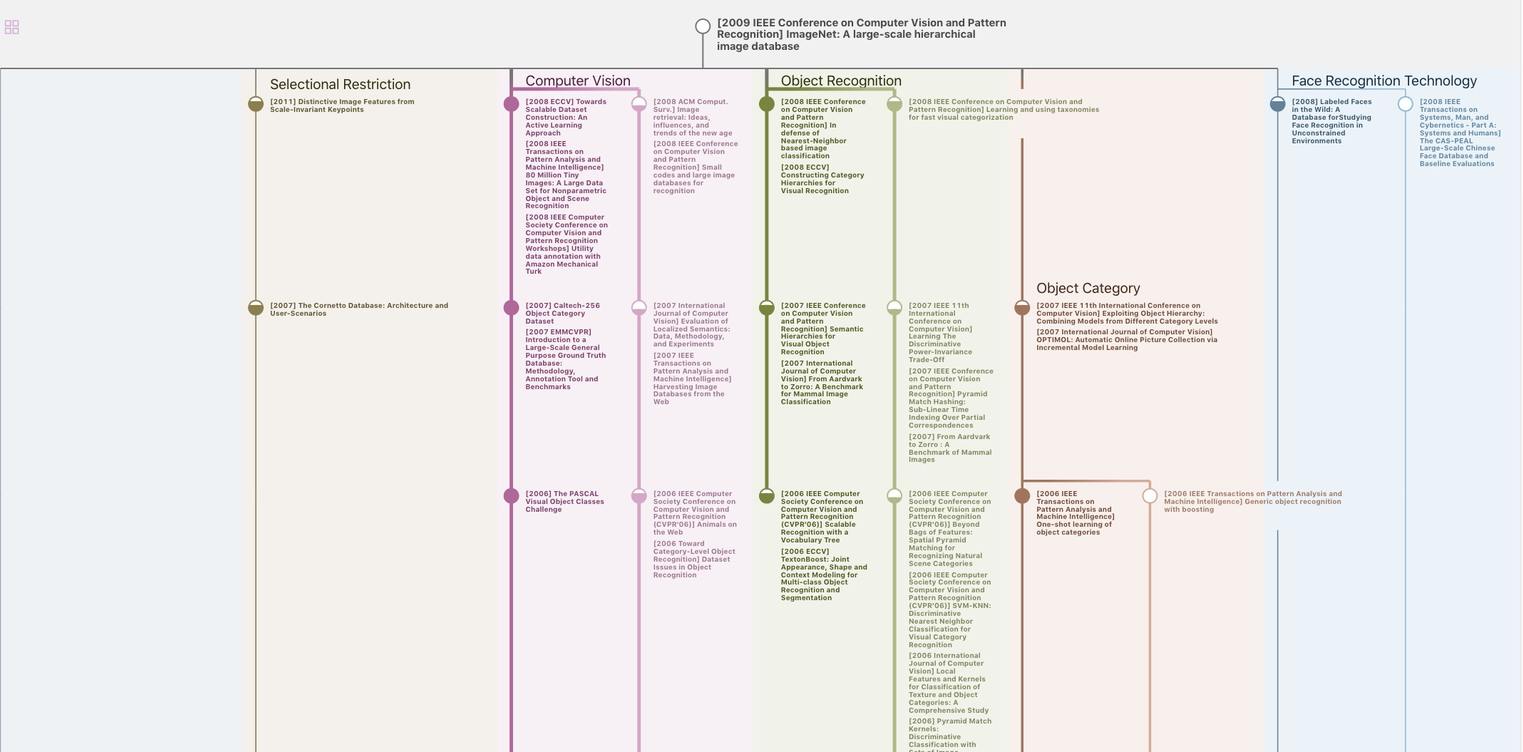
生成溯源树,研究论文发展脉络
Chat Paper
正在生成论文摘要