Knowledge-enhanced Agents for Interactive Text Games
CoRR(2023)
摘要
Communication via natural language is a key aspect of machine intelligence, and it requires computational models to learn and reason about world concepts, with varying levels of supervision. Significant progress has been made on fully-supervised non-interactive tasks, such as question-answering and procedural text understanding. Yet, various sequential interactive tasks, as in text-based games, have revealed limitations of existing approaches in terms of coherence, contextual awareness, and their ability to learn effectively from the environment. In this paper, we propose a knowledge-injection framework for improved functional grounding of agents in text-based games. Specifically, we consider two forms of domain knowledge that we inject into learning-based agents: memory of previous correct actions and affordances of relevant objects in the environment. Our framework supports two representative model classes: reinforcement learning agents and language model agents. Furthermore, we devise multiple injection strategies for the above domain knowledge types and agent architectures, including injection via knowledge graphs and augmentation of the existing input encoding strategies. We experiment with four models on the 10 tasks in the ScienceWorld text-based game environment, to illustrate the impact of knowledge injection on various model configurations and challenging task settings. Our findings provide crucial insights into the interplay between task properties, model architectures, and domain knowledge for interactive contexts.
更多查看译文
关键词
interactive text games,agents,knowledge-enhanced
AI 理解论文
溯源树
样例
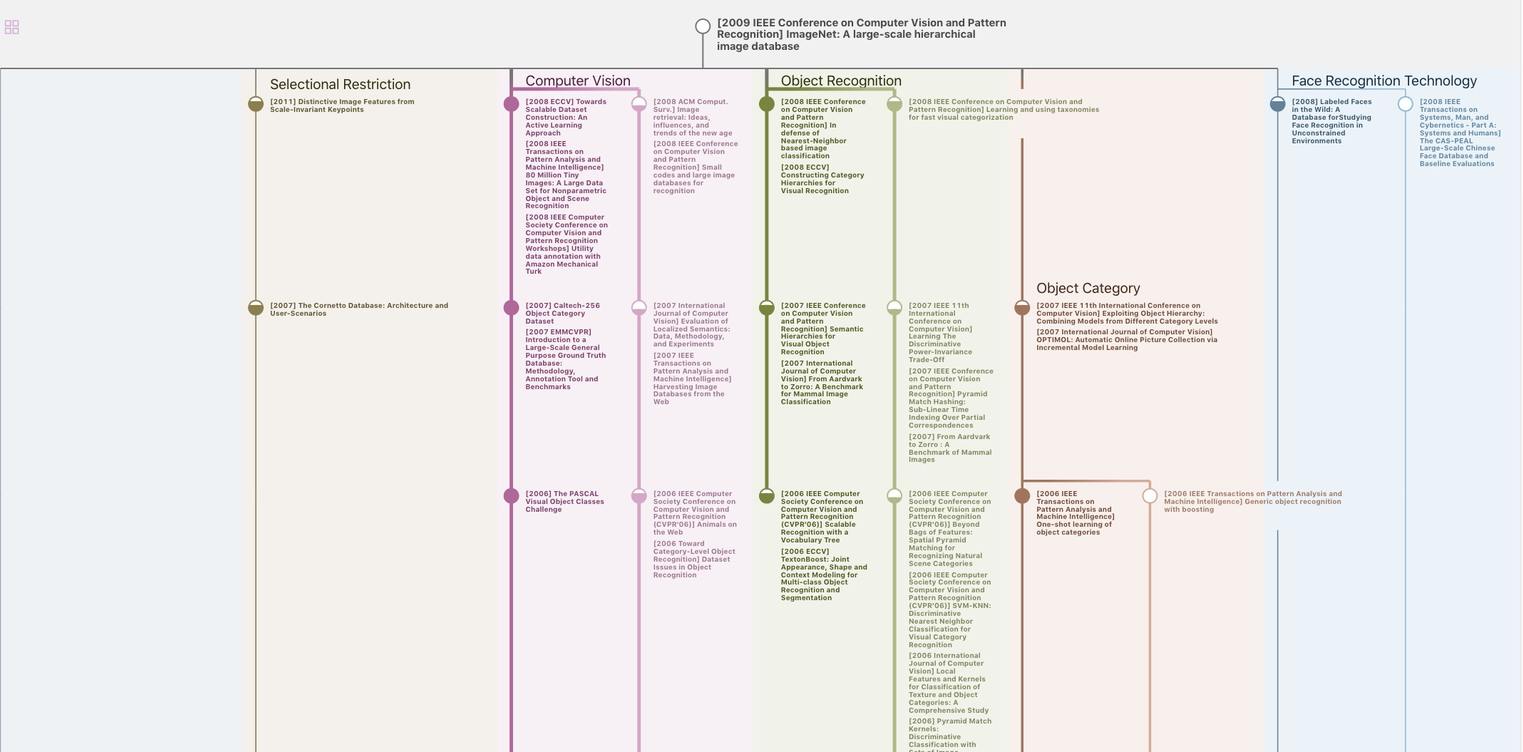
生成溯源树,研究论文发展脉络
Chat Paper
正在生成论文摘要