Predicting pathological highly invasive lung cancer from preoperative [18F]FDG PET/CT with multiple machine learning models
EUROPEAN JOURNAL OF NUCLEAR MEDICINE AND MOLECULAR IMAGING(2023)
摘要
Purpose The efficacy of sublobar resection of primary lung cancer have been proven in recent years. However, sublobar resection for highly invasive lung cancer increases local recurrence. We developed and validated multiple machine learning models predicting pathological invasiveness of lung cancer based on preoperative [F-18]fluorodeoxyglucose (FDG) positron emission tomography (PET) and computed tomography (CT) radiomic features. Methods Overall, 873 patients who underwent lobectomy or segmentectomy for primary lung cancer were enrolled. Radiomics features were extracted from preoperative PET/CT images with the PyRadiomics package. Seven machine learning models and an ensemble of all models (ENS) were evaluated after 100 iterations. In addition, the probability of highly invasive lung cancer was calculated in a nested cross-validation to assess the calibration plot and clinical usefulness and to compare to consolidation tumour ratio (CTR) on CT images, one of the generally used diagnostic criteria. Results In the training set, when PET and CT features were combined, all models achieved an area under the curve (AUC) of >= 0.880. In the test set, ENS showed the highest mean AUC of 0.880 and smallest standard deviation of 0.0165, and when the cutoff was 0.5, accuracy of 0.804, F1 of 0.851, precision of 0.821, and recall of 0.885. In the nested cross-validation, the AUC of 0.882 (95% CI: 0.860-0.905) showed a high discriminative ability, and the calibration plot indicated consistency with a Brier score of 0.131. A decision curve analysis showed that the ENS was valid with a threshold probability ranging from 3 to 98%. Accuracy showed an improvement of more than 8% over the CTR. Conclusion The machine learning model based on preoperative [F-18]FDG PET/CT images was able to predict pathological highly invasive lung cancer with high discriminative ability and stability. The calibration plot showed good consistency, suggesting its usefulness in quantitative risk assessment.
更多查看译文
关键词
Positron emission tomography,computed tomography,Lung cancer,Radiomics,Surgery,Machine learning
AI 理解论文
溯源树
样例
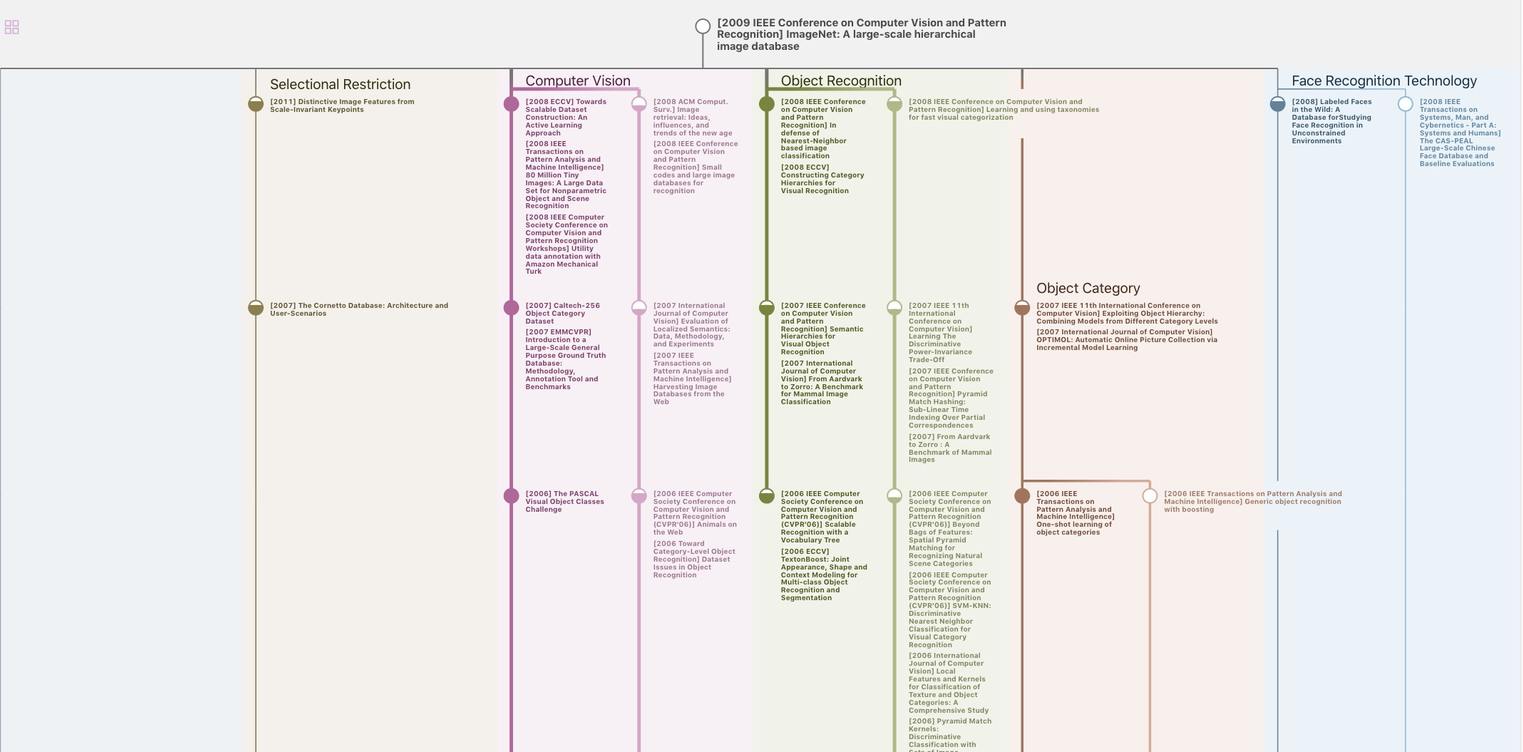
生成溯源树,研究论文发展脉络
Chat Paper
正在生成论文摘要