Accelerated Stochastic Optimization Methods under Quasar-convexity
ICML 2023(2023)
摘要
Non-convex optimization plays a key role in a growing number of machine learning applications. This motivates the identification of specialized structure that enables sharper theoretical analysis. One such identified structure is quasar-convexity, a non-convex generalization of convexity that subsumes convex functions. Existing algorithms for minimizing quasar-convex functions in the stochastic setting have either high complexity or slow convergence, which prompts us to derive a new class of stochastic methods for optimizing smooth quasar-convex functions. We demonstrate that our algorithms have fast convergence and outperform existing algorithms on several examples, including the classical problem of learning linear dynamical systems. We also present a unified analysis of our newly proposed algorithms and a previously studied deterministic algorithm.
更多查看译文
关键词
accelerated stochastic optimization methods,optimization methods,quasar-convexity
AI 理解论文
溯源树
样例
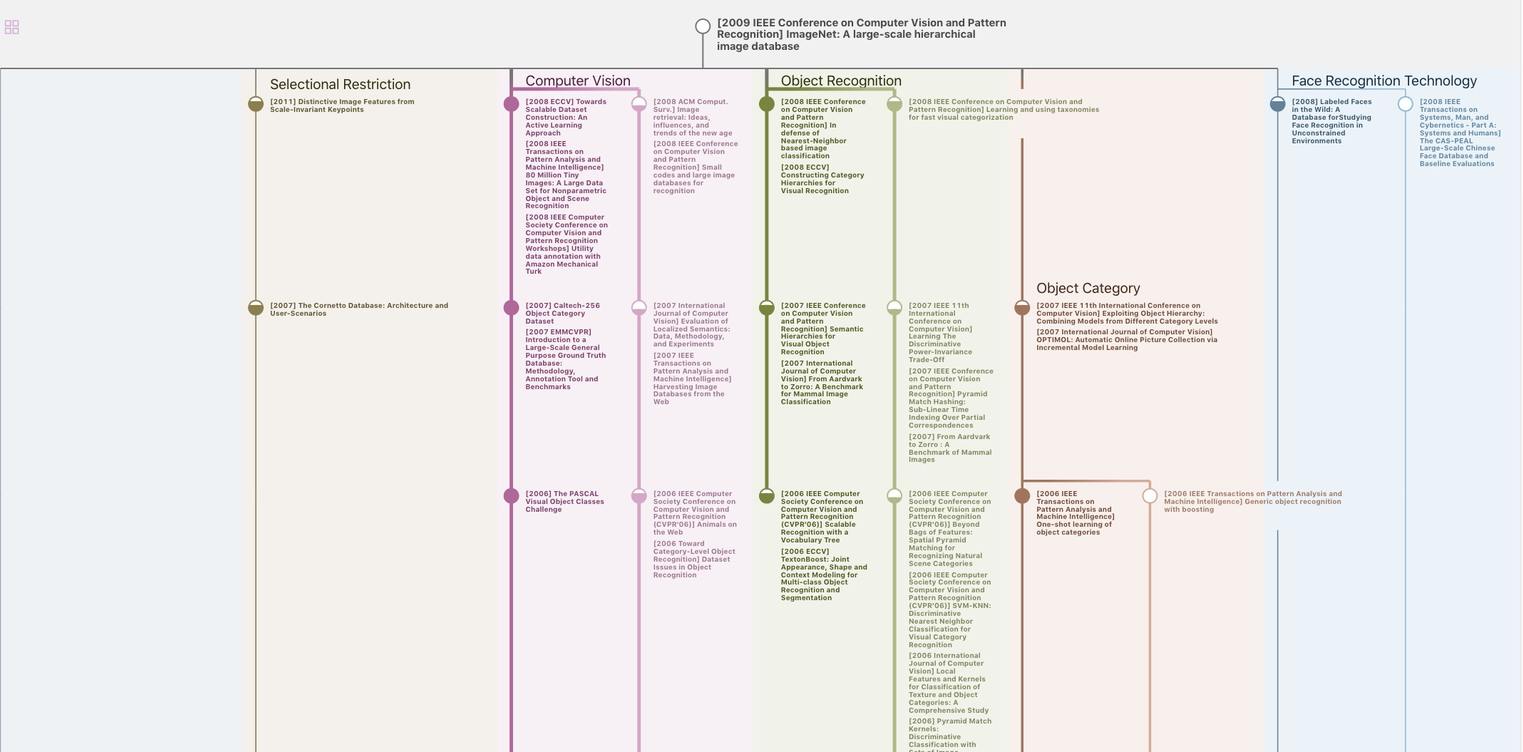
生成溯源树,研究论文发展脉络
Chat Paper
正在生成论文摘要