Analysis of Numerical Integration in RNN-Based Residuals for Fault Diagnosis of Dynamic Systems
IFAC PAPERSONLINE(2023)
摘要
Data-driven modeling and machine learning are widely used to model the behavior of dynamic systems. One application is the residual evaluation of technical systems where model predictions are compared with measurement data to create residuals for fault diagnosis applications. While recurrent neural network models have been shown capable of modeling complex non-linear dynamic systems, they are limited to fixed steps discrete-time simulation. Modeling using neural ordinary differential equations, however, make it possible to evaluate the state variables at specific times, compute gradients when training the model and use standard numerical solvers to explicitly model the underlying dynamic of the time-series data. Here, the effect of solver selection on the performance of neural ordinary differential equation residuals during training and evaluation is investigated. The paper includes a case study of a heavy-duty truck's after-treatment system to highlight the potential of these techniques for improving fault diagnosis performance.
更多查看译文
关键词
Simulation,Recurrent neural networks,Fault diagnosis,Neural ordinary differential,equations,Anomaly classification.
AI 理解论文
溯源树
样例
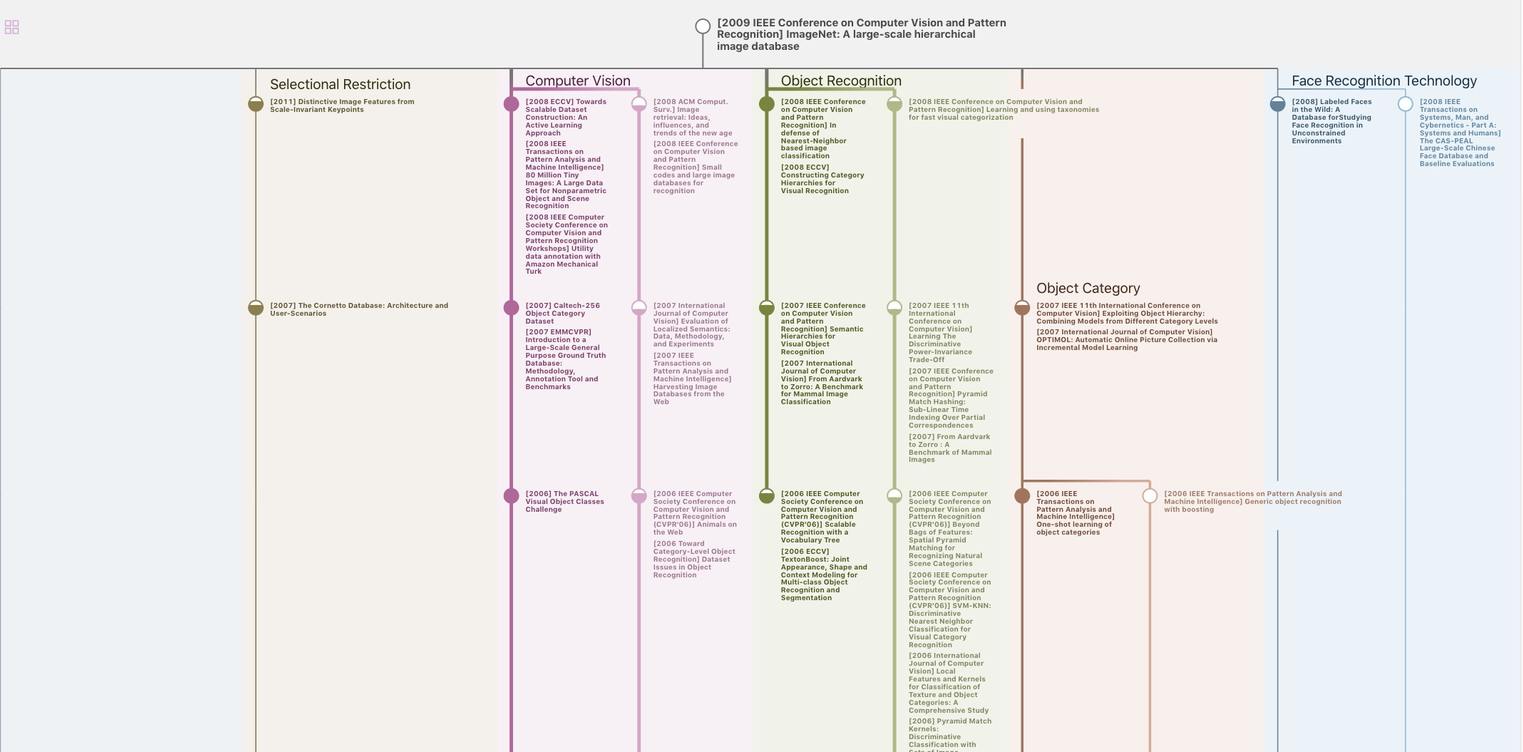
生成溯源树,研究论文发展脉络
Chat Paper
正在生成论文摘要