Diffusion Theory as a Scalpel: Detecting and Purifying Poisonous Dimensions in Pre-trained Language Models Caused by Backdoor or Bias
conf_acl(2023)
摘要
Pre-trained Language Models (PLMs) may be poisonous with backdoors or bias injected by the suspicious attacker during the fine-tuning process. A core challenge of purifying potentially poisonous PLMs is precisely finding poisonous dimensions. To settle this issue, we propose the Fine-purifying approach, which utilizes the diffusion theory to study the dynamic process of fine-tuning for finding potentially poisonous dimensions. According to the relationship between parameter drifts and Hessians of different dimensions, we can detect poisonous dimensions with abnormal dynamics, purify them by resetting them to clean pre-trained weights, and then fine-tune the purified weights on a small clean dataset. To the best of our knowledge, we are the first to study the dynamics guided by the diffusion theory for safety or defense purposes. Experimental results validate the effectiveness of Fine-purifying even with a small clean dataset.
更多查看译文
关键词
language models,bias,diffusion,purifying poisonous,pre-trained
AI 理解论文
溯源树
样例
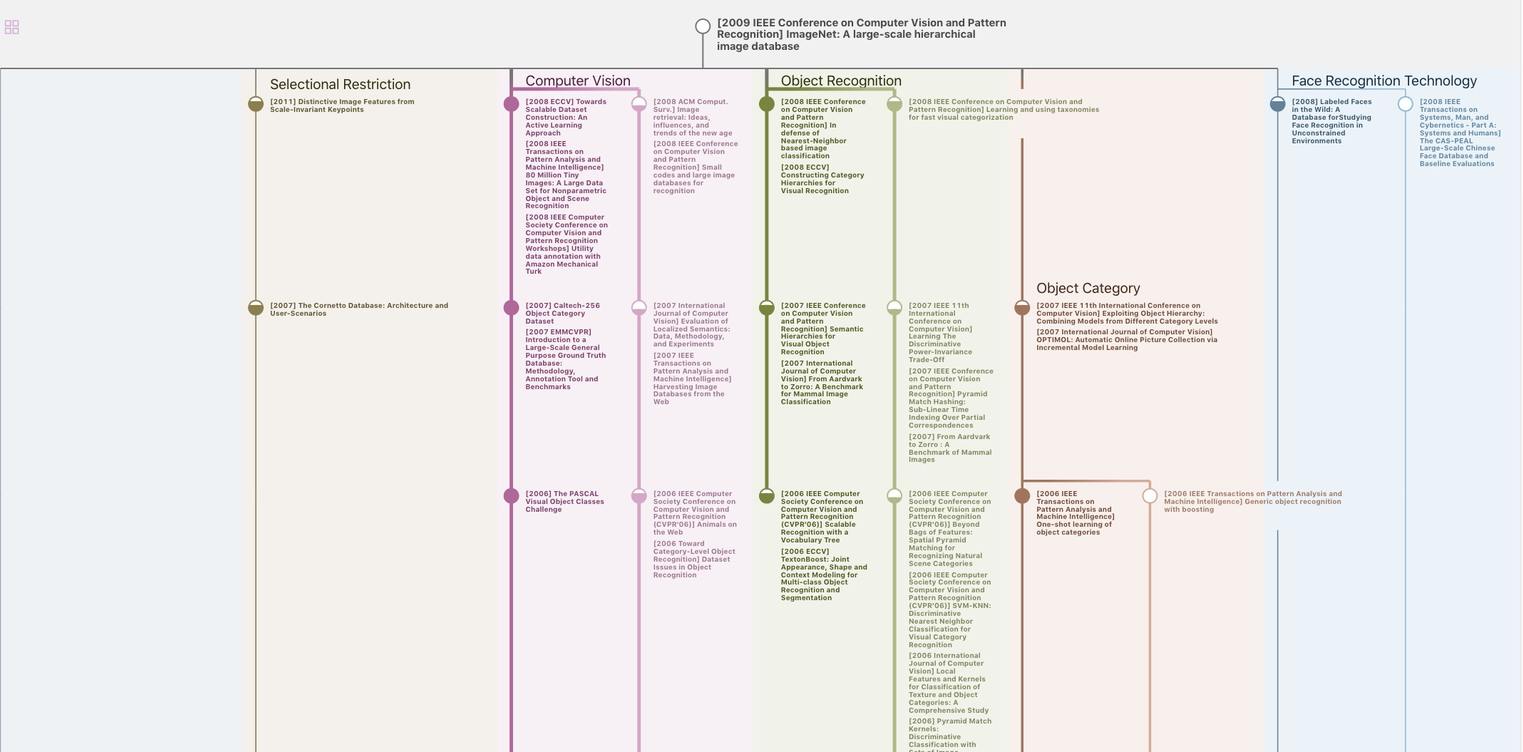
生成溯源树,研究论文发展脉络
Chat Paper
正在生成论文摘要