An Adversarial Non-Autoregressive Model for Text Generation with Incomplete Information
CoRR(2023)
摘要
Non-autoregressive models have been widely studied in the Complete Information Scenario (CIS), in which the models have complete input information to obtain corresponding output. However, their explorations in the Incomplete Information Scenario (IIS) are extremely limited. Our analyses reveal that the IIS's incomplete input information will augment the inherent limitations of existing non-autoregressive models trained under Maximum Likelihood Estimation. In this paper, we propose for the IIS an Adversarial Non-autoregressive Transformer (ANT) which has two novel features: 1) Position Aware Self-Modulation to provide more reasonable hidden representations, and 2) Dependency Feed Forward Network to strengthen its capacity in dependency modeling. We compare ANT with other mainstream models in the IIS and demonstrate that ANT can achieve comparable performance with much fewer decoding iterations. Furthermore, we show its great potential in various applications like latent interpolation and semi-supervised learning.
更多查看译文
关键词
text generation,non-autoregressive
AI 理解论文
溯源树
样例
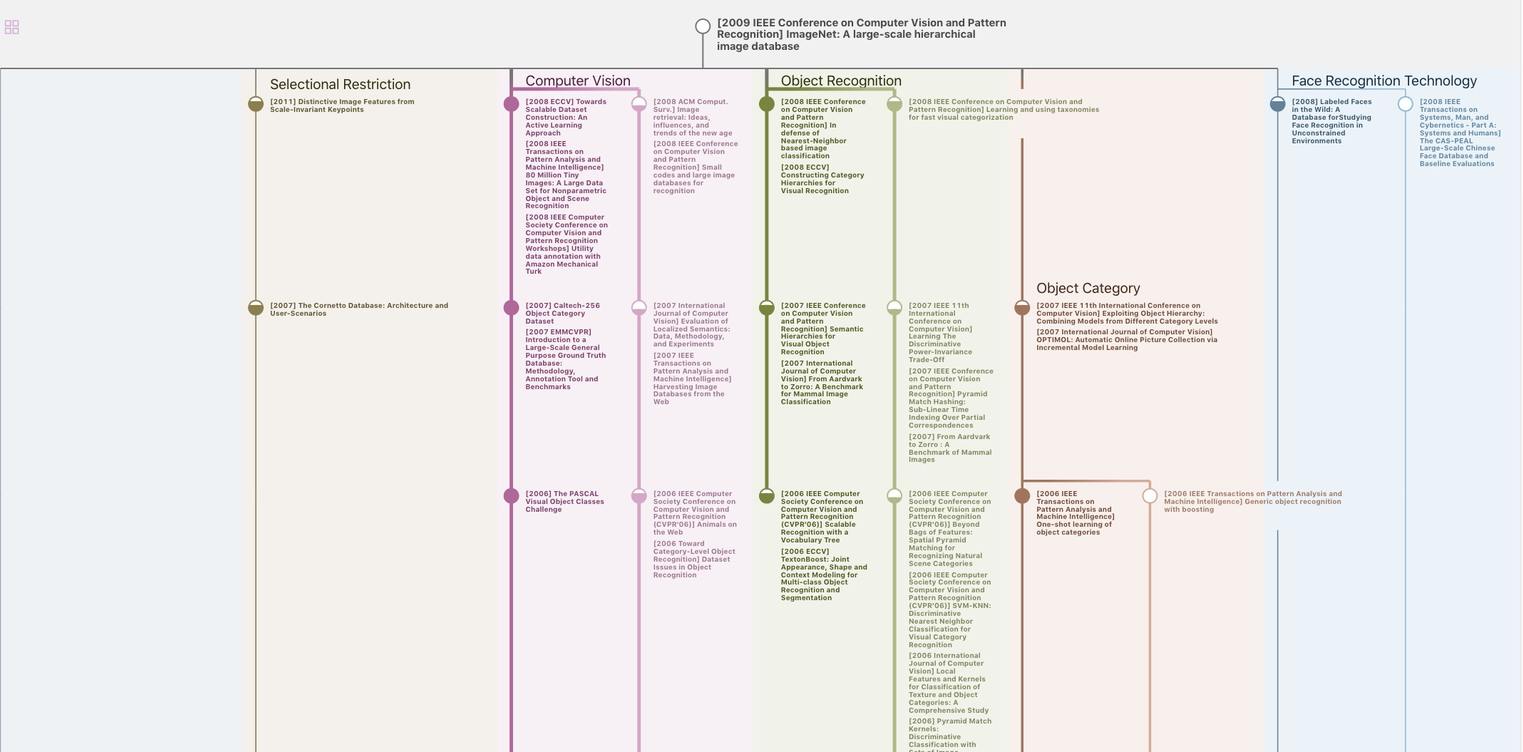
生成溯源树,研究论文发展脉络
Chat Paper
正在生成论文摘要