Causal Discovery with Stage Variables for Health Time Series
CoRR(2023)
摘要
Using observational data to learn causal relationships is essential when randomized experiments are not possible, such as in healthcare. Discovering causal relationships in time-series health data is even more challenging when relationships change over the course of a disease, such as medications that are most effective early on or for individuals with severe disease. Stage variables such as weeks of pregnancy, disease stages, or biomarkers like HbA1c, can influence what causal relationships are true for a patient. However, causal inference within each stage is often not possible due to limited amounts of data, and combining all data risks incorrect or missed inferences. To address this, we propose Causal Discovery with Stage Variables (CDSV), which uses stage variables to reweight data from multiple time-series while accounting for different causal relationships in each stage. In simulated data, CDSV discovers more causes with fewer false discoveries compared to baselines, in eICU it has a lower FDR than baselines, and in MIMIC-III it discovers more clinically relevant causes of high blood pressure.
更多查看译文
关键词
health time series,stage variables,discovery
AI 理解论文
溯源树
样例
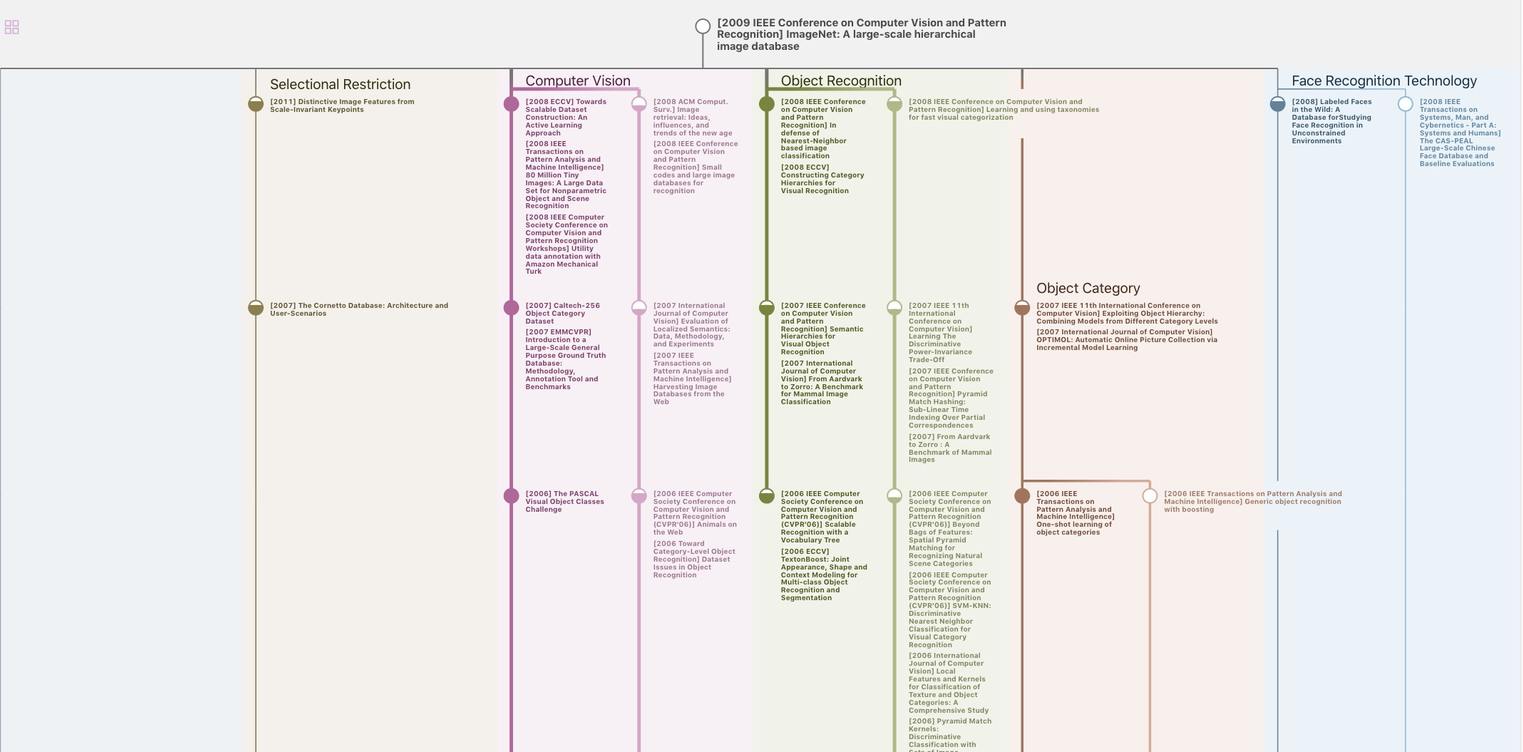
生成溯源树,研究论文发展脉络
Chat Paper
正在生成论文摘要