Steered Mixture-of-Experts Autoencoder Design for Real-Time Image Modelling and Denoising
arXiv (Cornell University)(2023)
摘要
Research in the past years introduced Steered Mixture-of-Experts (SMoE) as a framework to form sparse, edge-aware models for 2D- and higher dimensional pixel data, applicable to compression, denoising, and beyond, and capable to compete with state-of-the-art compression methods. To circumvent the computationally demanding, iterative optimization method used in prior works an autoencoder design is introduced that reduces the run-time drastically while simultaneously improving reconstruction quality for block-based SMoE approaches. Coupling a deep encoder network with a shallow, parameter-free SMoE decoder enforces an efficent and explainable latent representation. Our initial work on the autoencoder design presented a simple model, with limited applicability to compression and beyond. In this paper, we build on the foundation of the first autoencoder design and improve the reconstruction quality by expanding it to models of higher complexity and different block sizes. Furthermore, we improve the noise robustness of the autoencoder for SMoE denoising applications. Our results reveal that the newly adapted autoencoders allow ultra-fast estimation of parameters for complex SMoE models with excellent reconstruction quality, both for noise free input and under severe noise. This enables the SMoE image model framework for a wide range of image processing applications, including compression, noise reduction, and super-resolution.
更多查看译文
关键词
real-time real-time,modelling,mixture-of-experts
AI 理解论文
溯源树
样例
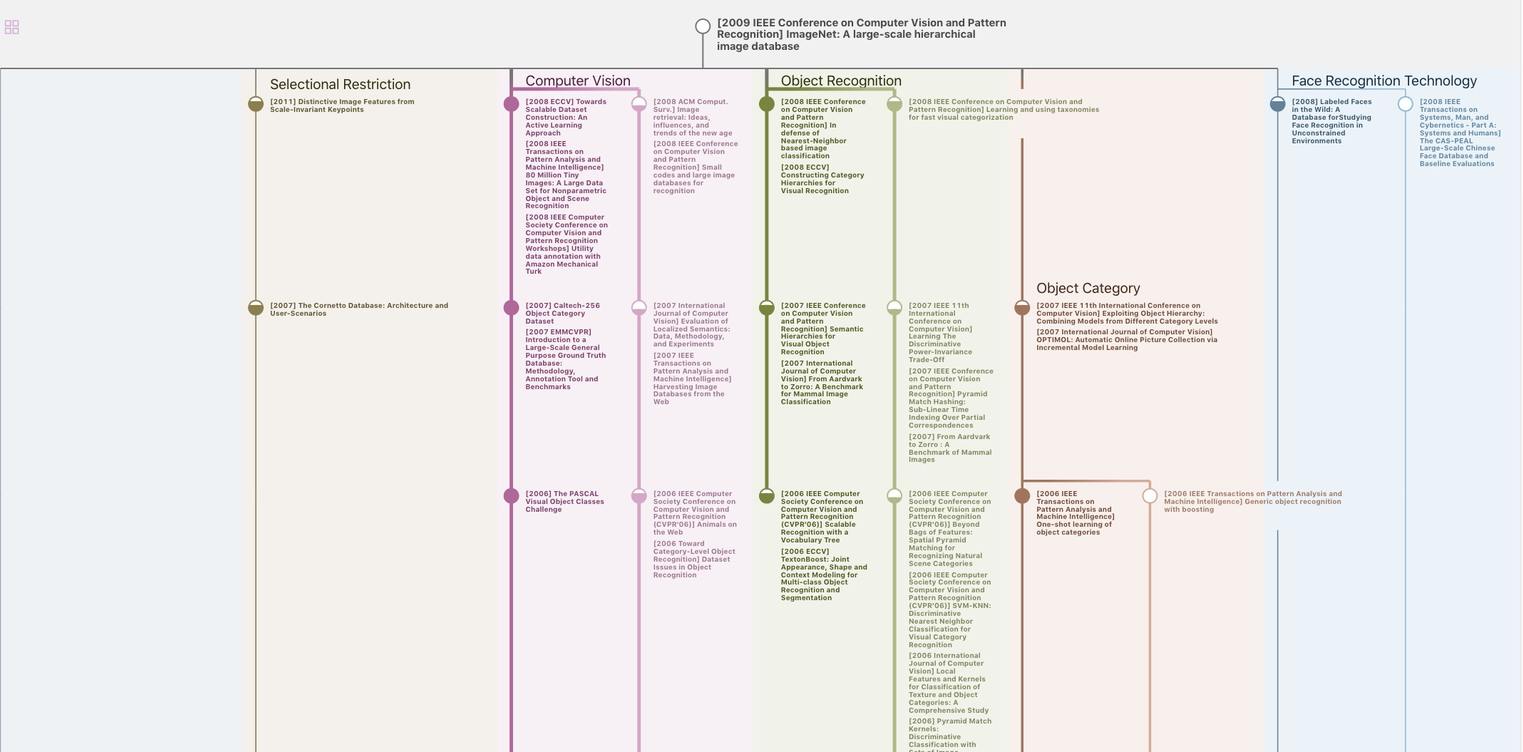
生成溯源树,研究论文发展脉络
Chat Paper
正在生成论文摘要