Emulation Learning for Neuromimetic Systems
2023 62ND IEEE CONFERENCE ON DECISION AND CONTROL, CDC(2023)
摘要
Building on our recent research on neural heuristic quantization systems, results on learning quantized motions and resilience to channel dropouts are reported. We propose a general emulation problem consistent with the neuromimetic paradigm. This optimal quantization problem can be solved by model predictive control (MPC), but because the optimization step involves integer programming, the approach suffers from combinatorial complexity when the number of input channels becomes large. Even if we collect data to train a neural network, collection of training data and the training itself are still time-consuming. Therefore, we propose a general Deep Q Network (DQN) algorithm that not only learns trajectories but also exhibits the advantages of resilience to channel dropout. Furthermore, to transfer the model to other emulation problems, a mapping-based transfer learning approach can be used directly on the current model to obtain the optimal trajectory steps for the new emulation problems.
更多查看译文
AI 理解论文
溯源树
样例
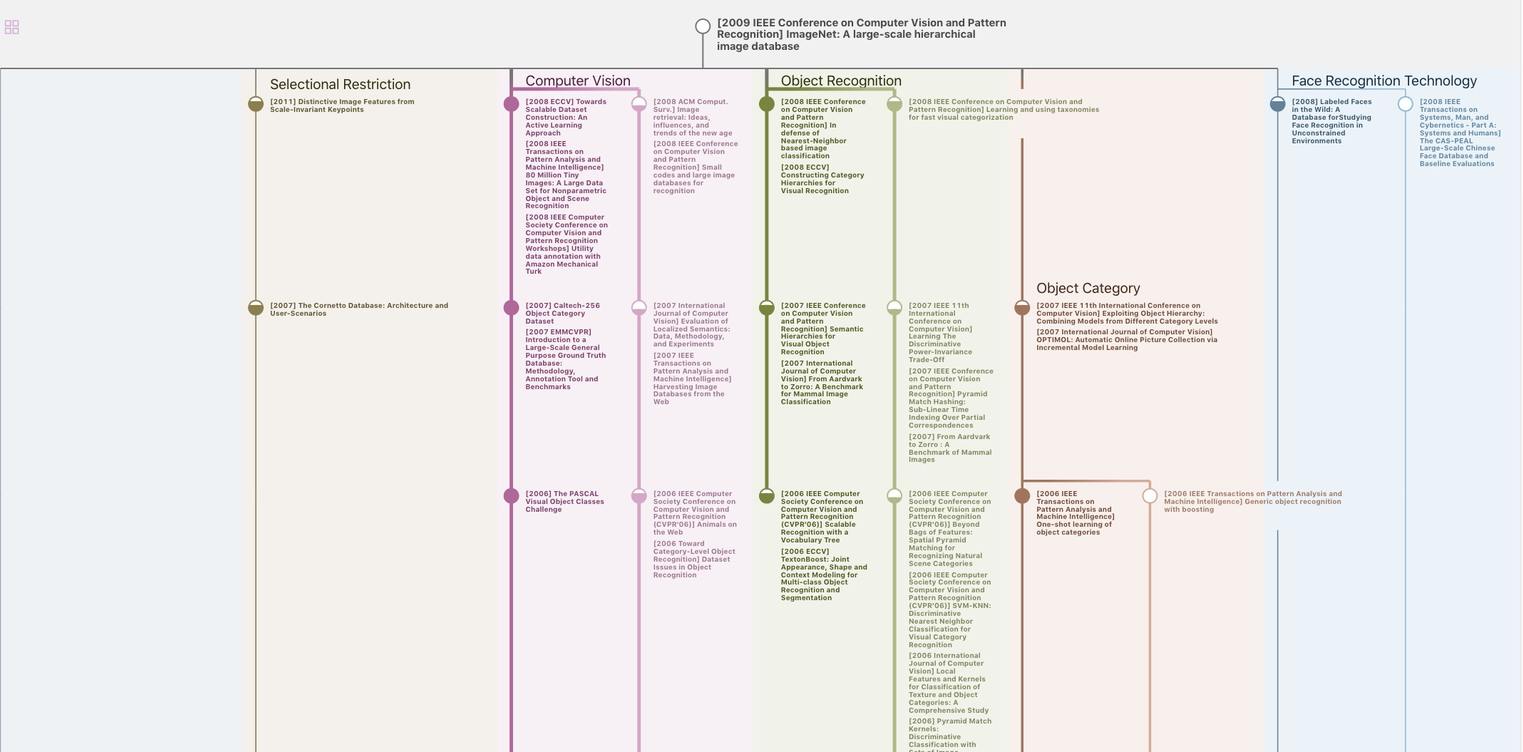
生成溯源树,研究论文发展脉络
Chat Paper
正在生成论文摘要